Initial commit
This commit is contained in:
commit
3b419bab6e
8
AUTHORS
Normal file
8
AUTHORS
Normal file
|
@ -0,0 +1,8 @@
|
||||||
|
Copyright © 2021 European University at St. Petersburg and Skolkovo Institute of Science and Technology
|
||||||
|
|
||||||
|
Moral rights:
|
||||||
|
Kirill Polovnikov
|
||||||
|
Nikita Pospelov
|
||||||
|
Dmitriy Skougarevskiy
|
||||||
|
|
||||||
|
The version control system provides attribution for specific lines of code.
|
29
CITATION.cff
Normal file
29
CITATION.cff
Normal file
|
@ -0,0 +1,29 @@
|
||||||
|
cff-version: 1.2.0
|
||||||
|
message: "If you use this algorithm, please cite it as below."
|
||||||
|
authors:
|
||||||
|
- family-names: "Polovnikov"
|
||||||
|
given-names: "Kirill"
|
||||||
|
orcid: "https://orcid.org/0000-0001-9903-9623"
|
||||||
|
- family-names: "Pospelov"
|
||||||
|
given-names: "Nikita"
|
||||||
|
- family-names: "Skougarevskiy"
|
||||||
|
given-names: "Dmitriy"
|
||||||
|
orcid: "0000-0002-4022-6210"
|
||||||
|
title: "α-Indirect Control in Onion-like Networks"
|
||||||
|
date-released: 2021-09-16
|
||||||
|
year: 2021
|
||||||
|
url: "https://github.com/eusporg/alphaicon"
|
||||||
|
preferred-citation:
|
||||||
|
type: unpublished
|
||||||
|
authors:
|
||||||
|
- family-names: "Polovnikov"
|
||||||
|
given-names: "Kirill"
|
||||||
|
orcid: "https://orcid.org/0000-0001-9903-9623"
|
||||||
|
- family-names: "Pospelov"
|
||||||
|
given-names: "Nikita"
|
||||||
|
- family-names: "Skougarevskiy"
|
||||||
|
given-names: "Dmitriy"
|
||||||
|
orcid: "0000-0002-4022-6210"
|
||||||
|
url: "https://arxiv.org/abs/2109.07181"
|
||||||
|
title: "α-Indirect Control in Onion-like Networks"
|
||||||
|
year: 2021
|
18
DEPENDENCIES
Normal file
18
DEPENDENCIES
Normal file
|
@ -0,0 +1,18 @@
|
||||||
|
Imports:
|
||||||
|
data.table (>= 1.13.2),
|
||||||
|
stringi (>= 1.4.4),
|
||||||
|
stringr (>= 1.3.1),
|
||||||
|
lubridate (>= 1.7.10),
|
||||||
|
remotes (>= 2.3.0),
|
||||||
|
usethis (>= 2.0.1),
|
||||||
|
ndjson (>= 0.8.0),
|
||||||
|
igraph (>= 1.2.6),
|
||||||
|
Matrix (>= 1.3-3),
|
||||||
|
matrixStats (>= 0.59.0),
|
||||||
|
stargazer (>= 5.2.1),
|
||||||
|
fastDummies (>= 1.6.3),
|
||||||
|
ggplot2 (>= 3.3.3),
|
||||||
|
ggthemes (>= 4.2.4),
|
||||||
|
ggrepel (>= 0.9.1),
|
||||||
|
ggnetwork (>= 0.5.9),
|
||||||
|
showtext (>= 0.9-2)
|
395
LICENSE
Normal file
395
LICENSE
Normal file
|
@ -0,0 +1,395 @@
|
||||||
|
Attribution 4.0 International
|
||||||
|
|
||||||
|
=======================================================================
|
||||||
|
|
||||||
|
Creative Commons Corporation ("Creative Commons") is not a law firm and
|
||||||
|
does not provide legal services or legal advice. Distribution of
|
||||||
|
Creative Commons public licenses does not create a lawyer-client or
|
||||||
|
other relationship. Creative Commons makes its licenses and related
|
||||||
|
information available on an "as-is" basis. Creative Commons gives no
|
||||||
|
warranties regarding its licenses, any material licensed under their
|
||||||
|
terms and conditions, or any related information. Creative Commons
|
||||||
|
disclaims all liability for damages resulting from their use to the
|
||||||
|
fullest extent possible.
|
||||||
|
|
||||||
|
Using Creative Commons Public Licenses
|
||||||
|
|
||||||
|
Creative Commons public licenses provide a standard set of terms and
|
||||||
|
conditions that creators and other rights holders may use to share
|
||||||
|
original works of authorship and other material subject to copyright
|
||||||
|
and certain other rights specified in the public license below. The
|
||||||
|
following considerations are for informational purposes only, are not
|
||||||
|
exhaustive, and do not form part of our licenses.
|
||||||
|
|
||||||
|
Considerations for licensors: Our public licenses are
|
||||||
|
intended for use by those authorized to give the public
|
||||||
|
permission to use material in ways otherwise restricted by
|
||||||
|
copyright and certain other rights. Our licenses are
|
||||||
|
irrevocable. Licensors should read and understand the terms
|
||||||
|
and conditions of the license they choose before applying it.
|
||||||
|
Licensors should also secure all rights necessary before
|
||||||
|
applying our licenses so that the public can reuse the
|
||||||
|
material as expected. Licensors should clearly mark any
|
||||||
|
material not subject to the license. This includes other CC-
|
||||||
|
licensed material, or material used under an exception or
|
||||||
|
limitation to copyright. More considerations for licensors:
|
||||||
|
wiki.creativecommons.org/Considerations_for_licensors
|
||||||
|
|
||||||
|
Considerations for the public: By using one of our public
|
||||||
|
licenses, a licensor grants the public permission to use the
|
||||||
|
licensed material under specified terms and conditions. If
|
||||||
|
the licensor's permission is not necessary for any reason--for
|
||||||
|
example, because of any applicable exception or limitation to
|
||||||
|
copyright--then that use is not regulated by the license. Our
|
||||||
|
licenses grant only permissions under copyright and certain
|
||||||
|
other rights that a licensor has authority to grant. Use of
|
||||||
|
the licensed material may still be restricted for other
|
||||||
|
reasons, including because others have copyright or other
|
||||||
|
rights in the material. A licensor may make special requests,
|
||||||
|
such as asking that all changes be marked or described.
|
||||||
|
Although not required by our licenses, you are encouraged to
|
||||||
|
respect those requests where reasonable. More_considerations
|
||||||
|
for the public:
|
||||||
|
wiki.creativecommons.org/Considerations_for_licensees
|
||||||
|
|
||||||
|
=======================================================================
|
||||||
|
|
||||||
|
Creative Commons Attribution 4.0 International Public License
|
||||||
|
|
||||||
|
By exercising the Licensed Rights (defined below), You accept and agree
|
||||||
|
to be bound by the terms and conditions of this Creative Commons
|
||||||
|
Attribution 4.0 International Public License ("Public License"). To the
|
||||||
|
extent this Public License may be interpreted as a contract, You are
|
||||||
|
granted the Licensed Rights in consideration of Your acceptance of
|
||||||
|
these terms and conditions, and the Licensor grants You such rights in
|
||||||
|
consideration of benefits the Licensor receives from making the
|
||||||
|
Licensed Material available under these terms and conditions.
|
||||||
|
|
||||||
|
|
||||||
|
Section 1 -- Definitions.
|
||||||
|
|
||||||
|
a. Adapted Material means material subject to Copyright and Similar
|
||||||
|
Rights that is derived from or based upon the Licensed Material
|
||||||
|
and in which the Licensed Material is translated, altered,
|
||||||
|
arranged, transformed, or otherwise modified in a manner requiring
|
||||||
|
permission under the Copyright and Similar Rights held by the
|
||||||
|
Licensor. For purposes of this Public License, where the Licensed
|
||||||
|
Material is a musical work, performance, or sound recording,
|
||||||
|
Adapted Material is always produced where the Licensed Material is
|
||||||
|
synched in timed relation with a moving image.
|
||||||
|
|
||||||
|
b. Adapter's License means the license You apply to Your Copyright
|
||||||
|
and Similar Rights in Your contributions to Adapted Material in
|
||||||
|
accordance with the terms and conditions of this Public License.
|
||||||
|
|
||||||
|
c. Copyright and Similar Rights means copyright and/or similar rights
|
||||||
|
closely related to copyright including, without limitation,
|
||||||
|
performance, broadcast, sound recording, and Sui Generis Database
|
||||||
|
Rights, without regard to how the rights are labeled or
|
||||||
|
categorized. For purposes of this Public License, the rights
|
||||||
|
specified in Section 2(b)(1)-(2) are not Copyright and Similar
|
||||||
|
Rights.
|
||||||
|
|
||||||
|
d. Effective Technological Measures means those measures that, in the
|
||||||
|
absence of proper authority, may not be circumvented under laws
|
||||||
|
fulfilling obligations under Article 11 of the WIPO Copyright
|
||||||
|
Treaty adopted on December 20, 1996, and/or similar international
|
||||||
|
agreements.
|
||||||
|
|
||||||
|
e. Exceptions and Limitations means fair use, fair dealing, and/or
|
||||||
|
any other exception or limitation to Copyright and Similar Rights
|
||||||
|
that applies to Your use of the Licensed Material.
|
||||||
|
|
||||||
|
f. Licensed Material means the artistic or literary work, database,
|
||||||
|
or other material to which the Licensor applied this Public
|
||||||
|
License.
|
||||||
|
|
||||||
|
g. Licensed Rights means the rights granted to You subject to the
|
||||||
|
terms and conditions of this Public License, which are limited to
|
||||||
|
all Copyright and Similar Rights that apply to Your use of the
|
||||||
|
Licensed Material and that the Licensor has authority to license.
|
||||||
|
|
||||||
|
h. Licensor means the individual(s) or entity(ies) granting rights
|
||||||
|
under this Public License.
|
||||||
|
|
||||||
|
i. Share means to provide material to the public by any means or
|
||||||
|
process that requires permission under the Licensed Rights, such
|
||||||
|
as reproduction, public display, public performance, distribution,
|
||||||
|
dissemination, communication, or importation, and to make material
|
||||||
|
available to the public including in ways that members of the
|
||||||
|
public may access the material from a place and at a time
|
||||||
|
individually chosen by them.
|
||||||
|
|
||||||
|
j. Sui Generis Database Rights means rights other than copyright
|
||||||
|
resulting from Directive 96/9/EC of the European Parliament and of
|
||||||
|
the Council of 11 March 1996 on the legal protection of databases,
|
||||||
|
as amended and/or succeeded, as well as other essentially
|
||||||
|
equivalent rights anywhere in the world.
|
||||||
|
|
||||||
|
k. You means the individual or entity exercising the Licensed Rights
|
||||||
|
under this Public License. Your has a corresponding meaning.
|
||||||
|
|
||||||
|
|
||||||
|
Section 2 -- Scope.
|
||||||
|
|
||||||
|
a. License grant.
|
||||||
|
|
||||||
|
1. Subject to the terms and conditions of this Public License,
|
||||||
|
the Licensor hereby grants You a worldwide, royalty-free,
|
||||||
|
non-sublicensable, non-exclusive, irrevocable license to
|
||||||
|
exercise the Licensed Rights in the Licensed Material to:
|
||||||
|
|
||||||
|
a. reproduce and Share the Licensed Material, in whole or
|
||||||
|
in part; and
|
||||||
|
|
||||||
|
b. produce, reproduce, and Share Adapted Material.
|
||||||
|
|
||||||
|
2. Exceptions and Limitations. For the avoidance of doubt, where
|
||||||
|
Exceptions and Limitations apply to Your use, this Public
|
||||||
|
License does not apply, and You do not need to comply with
|
||||||
|
its terms and conditions.
|
||||||
|
|
||||||
|
3. Term. The term of this Public License is specified in Section
|
||||||
|
6(a).
|
||||||
|
|
||||||
|
4. Media and formats; technical modifications allowed. The
|
||||||
|
Licensor authorizes You to exercise the Licensed Rights in
|
||||||
|
all media and formats whether now known or hereafter created,
|
||||||
|
and to make technical modifications necessary to do so. The
|
||||||
|
Licensor waives and/or agrees not to assert any right or
|
||||||
|
authority to forbid You from making technical modifications
|
||||||
|
necessary to exercise the Licensed Rights, including
|
||||||
|
technical modifications necessary to circumvent Effective
|
||||||
|
Technological Measures. For purposes of this Public License,
|
||||||
|
simply making modifications authorized by this Section 2(a)
|
||||||
|
(4) never produces Adapted Material.
|
||||||
|
|
||||||
|
5. Downstream recipients.
|
||||||
|
|
||||||
|
a. Offer from the Licensor -- Licensed Material. Every
|
||||||
|
recipient of the Licensed Material automatically
|
||||||
|
receives an offer from the Licensor to exercise the
|
||||||
|
Licensed Rights under the terms and conditions of this
|
||||||
|
Public License.
|
||||||
|
|
||||||
|
b. No downstream restrictions. You may not offer or impose
|
||||||
|
any additional or different terms or conditions on, or
|
||||||
|
apply any Effective Technological Measures to, the
|
||||||
|
Licensed Material if doing so restricts exercise of the
|
||||||
|
Licensed Rights by any recipient of the Licensed
|
||||||
|
Material.
|
||||||
|
|
||||||
|
6. No endorsement. Nothing in this Public License constitutes or
|
||||||
|
may be construed as permission to assert or imply that You
|
||||||
|
are, or that Your use of the Licensed Material is, connected
|
||||||
|
with, or sponsored, endorsed, or granted official status by,
|
||||||
|
the Licensor or others designated to receive attribution as
|
||||||
|
provided in Section 3(a)(1)(A)(i).
|
||||||
|
|
||||||
|
b. Other rights.
|
||||||
|
|
||||||
|
1. Moral rights, such as the right of integrity, are not
|
||||||
|
licensed under this Public License, nor are publicity,
|
||||||
|
privacy, and/or other similar personality rights; however, to
|
||||||
|
the extent possible, the Licensor waives and/or agrees not to
|
||||||
|
assert any such rights held by the Licensor to the limited
|
||||||
|
extent necessary to allow You to exercise the Licensed
|
||||||
|
Rights, but not otherwise.
|
||||||
|
|
||||||
|
2. Patent and trademark rights are not licensed under this
|
||||||
|
Public License.
|
||||||
|
|
||||||
|
3. To the extent possible, the Licensor waives any right to
|
||||||
|
collect royalties from You for the exercise of the Licensed
|
||||||
|
Rights, whether directly or through a collecting society
|
||||||
|
under any voluntary or waivable statutory or compulsory
|
||||||
|
licensing scheme. In all other cases the Licensor expressly
|
||||||
|
reserves any right to collect such royalties.
|
||||||
|
|
||||||
|
|
||||||
|
Section 3 -- License Conditions.
|
||||||
|
|
||||||
|
Your exercise of the Licensed Rights is expressly made subject to the
|
||||||
|
following conditions.
|
||||||
|
|
||||||
|
a. Attribution.
|
||||||
|
|
||||||
|
1. If You Share the Licensed Material (including in modified
|
||||||
|
form), You must:
|
||||||
|
|
||||||
|
a. retain the following if it is supplied by the Licensor
|
||||||
|
with the Licensed Material:
|
||||||
|
|
||||||
|
i. identification of the creator(s) of the Licensed
|
||||||
|
Material and any others designated to receive
|
||||||
|
attribution, in any reasonable manner requested by
|
||||||
|
the Licensor (including by pseudonym if
|
||||||
|
designated);
|
||||||
|
|
||||||
|
ii. a copyright notice;
|
||||||
|
|
||||||
|
iii. a notice that refers to this Public License;
|
||||||
|
|
||||||
|
iv. a notice that refers to the disclaimer of
|
||||||
|
warranties;
|
||||||
|
|
||||||
|
v. a URI or hyperlink to the Licensed Material to the
|
||||||
|
extent reasonably practicable;
|
||||||
|
|
||||||
|
b. indicate if You modified the Licensed Material and
|
||||||
|
retain an indication of any previous modifications; and
|
||||||
|
|
||||||
|
c. indicate the Licensed Material is licensed under this
|
||||||
|
Public License, and include the text of, or the URI or
|
||||||
|
hyperlink to, this Public License.
|
||||||
|
|
||||||
|
2. You may satisfy the conditions in Section 3(a)(1) in any
|
||||||
|
reasonable manner based on the medium, means, and context in
|
||||||
|
which You Share the Licensed Material. For example, it may be
|
||||||
|
reasonable to satisfy the conditions by providing a URI or
|
||||||
|
hyperlink to a resource that includes the required
|
||||||
|
information.
|
||||||
|
|
||||||
|
3. If requested by the Licensor, You must remove any of the
|
||||||
|
information required by Section 3(a)(1)(A) to the extent
|
||||||
|
reasonably practicable.
|
||||||
|
|
||||||
|
4. If You Share Adapted Material You produce, the Adapter's
|
||||||
|
License You apply must not prevent recipients of the Adapted
|
||||||
|
Material from complying with this Public License.
|
||||||
|
|
||||||
|
|
||||||
|
Section 4 -- Sui Generis Database Rights.
|
||||||
|
|
||||||
|
Where the Licensed Rights include Sui Generis Database Rights that
|
||||||
|
apply to Your use of the Licensed Material:
|
||||||
|
|
||||||
|
a. for the avoidance of doubt, Section 2(a)(1) grants You the right
|
||||||
|
to extract, reuse, reproduce, and Share all or a substantial
|
||||||
|
portion of the contents of the database;
|
||||||
|
|
||||||
|
b. if You include all or a substantial portion of the database
|
||||||
|
contents in a database in which You have Sui Generis Database
|
||||||
|
Rights, then the database in which You have Sui Generis Database
|
||||||
|
Rights (but not its individual contents) is Adapted Material; and
|
||||||
|
|
||||||
|
c. You must comply with the conditions in Section 3(a) if You Share
|
||||||
|
all or a substantial portion of the contents of the database.
|
||||||
|
|
||||||
|
For the avoidance of doubt, this Section 4 supplements and does not
|
||||||
|
replace Your obligations under this Public License where the Licensed
|
||||||
|
Rights include other Copyright and Similar Rights.
|
||||||
|
|
||||||
|
|
||||||
|
Section 5 -- Disclaimer of Warranties and Limitation of Liability.
|
||||||
|
|
||||||
|
a. UNLESS OTHERWISE SEPARATELY UNDERTAKEN BY THE LICENSOR, TO THE
|
||||||
|
EXTENT POSSIBLE, THE LICENSOR OFFERS THE LICENSED MATERIAL AS-IS
|
||||||
|
AND AS-AVAILABLE, AND MAKES NO REPRESENTATIONS OR WARRANTIES OF
|
||||||
|
ANY KIND CONCERNING THE LICENSED MATERIAL, WHETHER EXPRESS,
|
||||||
|
IMPLIED, STATUTORY, OR OTHER. THIS INCLUDES, WITHOUT LIMITATION,
|
||||||
|
WARRANTIES OF TITLE, MERCHANTABILITY, FITNESS FOR A PARTICULAR
|
||||||
|
PURPOSE, NON-INFRINGEMENT, ABSENCE OF LATENT OR OTHER DEFECTS,
|
||||||
|
ACCURACY, OR THE PRESENCE OR ABSENCE OF ERRORS, WHETHER OR NOT
|
||||||
|
KNOWN OR DISCOVERABLE. WHERE DISCLAIMERS OF WARRANTIES ARE NOT
|
||||||
|
ALLOWED IN FULL OR IN PART, THIS DISCLAIMER MAY NOT APPLY TO YOU.
|
||||||
|
|
||||||
|
b. TO THE EXTENT POSSIBLE, IN NO EVENT WILL THE LICENSOR BE LIABLE
|
||||||
|
TO YOU ON ANY LEGAL THEORY (INCLUDING, WITHOUT LIMITATION,
|
||||||
|
NEGLIGENCE) OR OTHERWISE FOR ANY DIRECT, SPECIAL, INDIRECT,
|
||||||
|
INCIDENTAL, CONSEQUENTIAL, PUNITIVE, EXEMPLARY, OR OTHER LOSSES,
|
||||||
|
COSTS, EXPENSES, OR DAMAGES ARISING OUT OF THIS PUBLIC LICENSE OR
|
||||||
|
USE OF THE LICENSED MATERIAL, EVEN IF THE LICENSOR HAS BEEN
|
||||||
|
ADVISED OF THE POSSIBILITY OF SUCH LOSSES, COSTS, EXPENSES, OR
|
||||||
|
DAMAGES. WHERE A LIMITATION OF LIABILITY IS NOT ALLOWED IN FULL OR
|
||||||
|
IN PART, THIS LIMITATION MAY NOT APPLY TO YOU.
|
||||||
|
|
||||||
|
c. The disclaimer of warranties and limitation of liability provided
|
||||||
|
above shall be interpreted in a manner that, to the extent
|
||||||
|
possible, most closely approximates an absolute disclaimer and
|
||||||
|
waiver of all liability.
|
||||||
|
|
||||||
|
|
||||||
|
Section 6 -- Term and Termination.
|
||||||
|
|
||||||
|
a. This Public License applies for the term of the Copyright and
|
||||||
|
Similar Rights licensed here. However, if You fail to comply with
|
||||||
|
this Public License, then Your rights under this Public License
|
||||||
|
terminate automatically.
|
||||||
|
|
||||||
|
b. Where Your right to use the Licensed Material has terminated under
|
||||||
|
Section 6(a), it reinstates:
|
||||||
|
|
||||||
|
1. automatically as of the date the violation is cured, provided
|
||||||
|
it is cured within 30 days of Your discovery of the
|
||||||
|
violation; or
|
||||||
|
|
||||||
|
2. upon express reinstatement by the Licensor.
|
||||||
|
|
||||||
|
For the avoidance of doubt, this Section 6(b) does not affect any
|
||||||
|
right the Licensor may have to seek remedies for Your violations
|
||||||
|
of this Public License.
|
||||||
|
|
||||||
|
c. For the avoidance of doubt, the Licensor may also offer the
|
||||||
|
Licensed Material under separate terms or conditions or stop
|
||||||
|
distributing the Licensed Material at any time; however, doing so
|
||||||
|
will not terminate this Public License.
|
||||||
|
|
||||||
|
d. Sections 1, 5, 6, 7, and 8 survive termination of this Public
|
||||||
|
License.
|
||||||
|
|
||||||
|
|
||||||
|
Section 7 -- Other Terms and Conditions.
|
||||||
|
|
||||||
|
a. The Licensor shall not be bound by any additional or different
|
||||||
|
terms or conditions communicated by You unless expressly agreed.
|
||||||
|
|
||||||
|
b. Any arrangements, understandings, or agreements regarding the
|
||||||
|
Licensed Material not stated herein are separate from and
|
||||||
|
independent of the terms and conditions of this Public License.
|
||||||
|
|
||||||
|
|
||||||
|
Section 8 -- Interpretation.
|
||||||
|
|
||||||
|
a. For the avoidance of doubt, this Public License does not, and
|
||||||
|
shall not be interpreted to, reduce, limit, restrict, or impose
|
||||||
|
conditions on any use of the Licensed Material that could lawfully
|
||||||
|
be made without permission under this Public License.
|
||||||
|
|
||||||
|
b. To the extent possible, if any provision of this Public License is
|
||||||
|
deemed unenforceable, it shall be automatically reformed to the
|
||||||
|
minimum extent necessary to make it enforceable. If the provision
|
||||||
|
cannot be reformed, it shall be severed from this Public License
|
||||||
|
without affecting the enforceability of the remaining terms and
|
||||||
|
conditions.
|
||||||
|
|
||||||
|
c. No term or condition of this Public License will be waived and no
|
||||||
|
failure to comply consented to unless expressly agreed to by the
|
||||||
|
Licensor.
|
||||||
|
|
||||||
|
d. Nothing in this Public License constitutes or may be interpreted
|
||||||
|
as a limitation upon, or waiver of, any privileges and immunities
|
||||||
|
that apply to the Licensor or You, including from the legal
|
||||||
|
processes of any jurisdiction or authority.
|
||||||
|
|
||||||
|
|
||||||
|
=======================================================================
|
||||||
|
|
||||||
|
Creative Commons is not a party to its public
|
||||||
|
licenses. Notwithstanding, Creative Commons may elect to apply one of
|
||||||
|
its public licenses to material it publishes and in those instances
|
||||||
|
will be considered the “Licensor.” The text of the Creative Commons
|
||||||
|
public licenses is dedicated to the public domain under the CC0 Public
|
||||||
|
Domain Dedication. Except for the limited purpose of indicating that
|
||||||
|
material is shared under a Creative Commons public license or as
|
||||||
|
otherwise permitted by the Creative Commons policies published at
|
||||||
|
creativecommons.org/policies, Creative Commons does not authorize the
|
||||||
|
use of the trademark "Creative Commons" or any other trademark or logo
|
||||||
|
of Creative Commons without its prior written consent including,
|
||||||
|
without limitation, in connection with any unauthorized modifications
|
||||||
|
to any of its public licenses or any other arrangements,
|
||||||
|
understandings, or agreements concerning use of licensed material. For
|
||||||
|
the avoidance of doubt, this paragraph does not form part of the
|
||||||
|
public licenses.
|
||||||
|
|
||||||
|
Creative Commons may be contacted at creativecommons.org.
|
102
Makefile
Normal file
102
Makefile
Normal file
|
@ -0,0 +1,102 @@
|
||||||
|
# List of R dependencies for the project
|
||||||
|
DEPENDENCIES:
|
||||||
|
# Do nothing, the file is created outside the repo
|
||||||
|
noop
|
||||||
|
|
||||||
|
# Helper function to install the dependencies
|
||||||
|
code/helper_functions/install_dependencies.r:
|
||||||
|
# Do nothing, the file is created outside the repo
|
||||||
|
noop
|
||||||
|
|
||||||
|
# People with Significant Control snapshot from Companies House
|
||||||
|
data/uk/persons-with-significant-control-snapshot-2021-08-02.txt:
|
||||||
|
# Do nothing, the file is created outside the repo
|
||||||
|
noop
|
||||||
|
|
||||||
|
# Company Data Product snapshot from Companies House
|
||||||
|
data/uk/BasicCompanyDataAsOneFile-2021-08-01.csv:
|
||||||
|
# Do nothing, the file is created outside the repo
|
||||||
|
noop
|
||||||
|
|
||||||
|
# Industry sector names
|
||||||
|
data/uk/sic_2007_code_list.csv:
|
||||||
|
# Do nothing, the file is created outside the repo
|
||||||
|
noop
|
||||||
|
|
||||||
|
# CorpWatch SEC 10-K filings data: company name-id mapping
|
||||||
|
data/corpwatch_api_tables_csv_14aug21/cik_name_lookup.csv:
|
||||||
|
# Do nothing, the file is created outside the repo
|
||||||
|
noop
|
||||||
|
|
||||||
|
# CorpWatch SEC 10-K filings data: basic company information
|
||||||
|
data/corpwatch_api_tables_csv_14aug21/company_info.csv:
|
||||||
|
# Do nothing, the file is created outside the repo
|
||||||
|
noop
|
||||||
|
|
||||||
|
# CorpWatch SEC 10-K filings data: company locations
|
||||||
|
data/corpwatch_api_tables_csv_14aug21/company_locations.csv:
|
||||||
|
# Do nothing, the file is created outside the repo
|
||||||
|
noop
|
||||||
|
|
||||||
|
# Process the PSC snapshot
|
||||||
|
data/uk/psc_snapshot_2021-08-02.rdata: data/uk/persons-with-significant-control-snapshot-2021-08-02.txt
|
||||||
|
Rscript code/data_preparation/uk/1a_process_psc_snapshot.r
|
||||||
|
|
||||||
|
# Process the live snapshot of companies data
|
||||||
|
data/uk/uk_basic_companies_data_2021-08-01.rdata: data/uk/BasicCompanyDataAsOneFile-2021-08-01.csv data/uk/sic_2007_code_list.csv
|
||||||
|
Rscript code/data_preparation/uk/1b_process_companies_data.r
|
||||||
|
|
||||||
|
# Convert the PSC snapshot to a company-participant clean data
|
||||||
|
output/uk/uk_organisations_participants_2021_long_2aug21.csv: data/uk/psc_snapshot_2021-08-02.rdata data/uk/uk_basic_companies_data_2021-08-01.rdata
|
||||||
|
Rscript code/data_preparation/uk/2_psc_snapshot_to_participants_panel
|
||||||
|
|
||||||
|
# Create SEC 10-K Exhibit 21 company-participant evaluation set matched to PSC and live companies
|
||||||
|
data/uk/uk_parent_subsidiary_mapping_2020_2021_sec_filers_exhibit21.csv: data/corpwatch_api_tables_csv_14aug21/company_info.csv data/corpwatch_api_tables_csv_14aug21/cik_name_lookup.csv data/corpwatch_api_tables_csv_14aug21/company_locations.csv data/uk/psc_snapshot_2021-08-02.rdata data/uk/uk_basic_companies_data_2021-08-01.rdata
|
||||||
|
Rscript code/data_preparation/uk/3_prepare_affiliated_entities_evaluation_data.r
|
||||||
|
|
||||||
|
# Classify the network into SH, ST, C, and I entities
|
||||||
|
output/uk/uk_organisations_participation_graph_core_periphery_membership_6aug21.csv: output/uk/uk_organisations_participants_2021_long_2aug21.csv
|
||||||
|
jupyter nbconvert --ExecutePreprocessor.timeout=-1 --execute code/alphaicon_paper/1_compute_alphaicon.ipynb
|
||||||
|
|
||||||
|
# Compute the shares by transitivity
|
||||||
|
transitiveshares := $(wildcard output/uk/transitive/uk_organisations_transitive_ownership_alpha*.csv)
|
||||||
|
$(transitiveshares): output/uk/uk_organisations_participants_2021_long_2aug21.csv output/uk/uk_organisations_participation_graph_core_periphery_membership_6aug21.csv
|
||||||
|
jupyter nbconvert --ExecutePreprocessor.timeout=-1 --execute code/alphaicon_paper/1_compute_alphaicon.ipynb
|
||||||
|
|
||||||
|
# Helper function implementing NPI/DPI computation
|
||||||
|
code/helper_functions/compute_power_index.r:
|
||||||
|
# Do nothing, the file is created outside the repo
|
||||||
|
noop
|
||||||
|
|
||||||
|
# Compute the DPI shares
|
||||||
|
output/uk/npi_dpi/10000iter/uk_organisations_participants_2021_long_7sep21_dpi_10000iter.csv: code/helper_functions/compute_power_index.r
|
||||||
|
Rscript code/alphaicon_paper/2_compute_npi_dpi.r
|
||||||
|
|
||||||
|
# Compute the NPI shares
|
||||||
|
output/uk/npi_dpi/10000iter/uk_organisations_participants_2021_long_7sep21_npi_10000iter.csv: code/helper_functions/compute_power_index.r
|
||||||
|
Rscript code/alphaicon_paper/2_compute_npi_dpi.r
|
||||||
|
|
||||||
|
# Perform the evaluation of algorithms at different k
|
||||||
|
output/alphaicon_paper/uk_orgs_algorithm_evaluation_recall.csv: output/uk/uk_organisations_participants_2021_long_2aug21.csv output/uk/npi_dpi/10000iter/uk_organisations_participants_2021_long_7sep21_dpi_10000iter.csv output/uk/npi_dpi/10000iter/uk_organisations_participants_2021_long_7sep21_npi_10000iter.csv $(transitiveshares) output/uk/uk_organisations_participation_graph_core_periphery_membership_6aug21.csv data/uk/uk_parent_subsidiary_mapping_2020_2021_sec_filers_exhibit21.csv
|
||||||
|
Rscript code/alphaicon_paper/5_algorithm_evaluation.r
|
||||||
|
|
||||||
|
# Perform the evaluation of algorithms at different path length
|
||||||
|
output/alphaicon_paper/uk_orgs_algorithm_evaluation_recall_by_pathlength.csv: output/uk/uk_organisations_participants_2021_long_2aug21.csv output/uk/npi_dpi/10000iter/uk_organisations_participants_2021_long_7sep21_dpi_10000iter.csv output/uk/npi_dpi/10000iter/uk_organisations_participants_2021_long_7sep21_npi_10000iter.csv $(transitiveshares) output/uk/uk_organisations_participation_graph_core_periphery_membership_6aug21.csv data/uk/uk_parent_subsidiary_mapping_2020_2021_sec_filers_exhibit21.csv
|
||||||
|
Rscript code/alphaicon_paper/5_algorithm_evaluation.r
|
||||||
|
|
||||||
|
# Create the ranking of top-100 holders by each method
|
||||||
|
output/alphaicon_paper/uk_organisations_top100_holders_2021_long_2aug21.csv: output/uk/uk_organisations_participants_2021_long_2aug21.csv output/uk/npi_dpi/10000iter/uk_organisations_participants_2021_long_7sep21_dpi_10000iter.csv output/uk/npi_dpi/10000iter/uk_organisations_participants_2021_long_7sep21_npi_10000iter.csv $(transitiveshares) output/uk/uk_organisations_participation_graph_core_periphery_membership_6aug21.csv data/uk/uk_parent_subsidiary_mapping_2020_2021_sec_filers_exhibit21.csv
|
||||||
|
Rscript code/alphaicon_paper/6_rank_top_holders.r
|
||||||
|
|
||||||
|
# Compute Kendall's tau-b rank correlation of per-company participants for different methods
|
||||||
|
output/alphaicon_paper/kendall_taus_participant_ranks_dpi_npi_transitive_uk_organisations_participants_2021_7sep21.csv: output/uk/uk_organisations_participants_2021_long_2aug21.csv output/uk/npi_dpi/10000iter/uk_organisations_participants_2021_long_7sep21_dpi_10000iter.csv output/uk/npi_dpi/10000iter/uk_organisations_participants_2021_long_7sep21_npi_10000iter.csv $(transitiveshares) output/uk/uk_organisations_participation_graph_core_periphery_membership_6aug21.csv data/uk/uk_parent_subsidiary_mapping_2020_2021_sec_filers_exhibit21.csv
|
||||||
|
Rscript code/alphaicon_paper/6_rank_top_holders.r
|
||||||
|
|
||||||
|
# α-ICON paper
|
||||||
|
alphaicon_paper: output/alphaicon_paper/uk_organisations_top100_holders_2021_long_2aug21.csv output/alphaicon_paper/uk_orgs_algorithm_evaluation_recall.csv output/alphaicon_paper/uk_orgs_algorithm_evaluation_recall_by_pathlength.csv DEPENDENCIES
|
||||||
|
Rscript code/helper_functions/install_dependencies.r
|
||||||
|
Rscript code/helper_functions/compute_power_index.r
|
||||||
|
Rscript code/alphaicon_paper/3_summary_stat_by_node_type.r
|
||||||
|
Rscript code/alphaicon_paper/4_illustrate_algorithm.r
|
||||||
|
Rscript code/alphaicon_paper/5_algorithm_evaluation.r
|
||||||
|
Rscript code/alphaicon_paper/6_rank_top_holders.r
|
113
README.md
Normal file
113
README.md
Normal file
|
@ -0,0 +1,113 @@
|
||||||
|
|
||||||
|
<table>
|
||||||
|
<tbody>
|
||||||
|
<tr>
|
||||||
|
<td valign="top" width=200><img src="https://user-images.githubusercontent.com/3776887/133301237-145e43f0-d4b3-4ae5-bf15-113efc2ad189.png"></td>
|
||||||
|
<td valign="top"><h1>α-Indirect Control in Onion-like Networks</h1>
|
||||||
|
We propose a fast, accurate, and scalable algorithm to detect ultimate controlling entities in global corporate networks. α-ICON uses company-participant links to identify super-holders who exert control in networks with millions of nodes.<br><br>
|
||||||
|
By exploiting onion-like properties of such networks we iteratively peel off the hanging vertices until a dense core remains. This procedure allows for a dramatic speed-up, uncovers meaningful structures, and handles circular ownership by design.<br><br>
|
||||||
|
Read our <a href="https://arxiv.org/abs/2109.07181" target="_blank">paper</a> with the applications. As a toy example, consider the below corporate network where α-ICON designates Mr Philip Mactaggart (in green) as the super-holder exerting control over all other entities, directly or indirectly held:
|
||||||
|
</td>
|
||||||
|
</tr>
|
||||||
|
</tbody>
|
||||||
|
</table>
|
||||||
|
|
||||||
|
<img src="https://user-images.githubusercontent.com/3776887/133299028-152f030a-e1c7-428b-83ef-e5f4e92414bc.png">
|
||||||
|
|
||||||
|
## Installation
|
||||||
|
|
||||||
|
To replicate the analysis you need to clone this repository to your local machine. Then you need to install the required versions of R dependencies listed in `DEPENDENCIES`. `code/helper_functions/install_dependencies.r` automates this step, but you may still need to install the underlying libraries manually with [Homebrew](https://brew.sh) or `apt-get`, depending on your platform. Finally, you need to declare the environment variable `ALPHAICON_PATH` in bash pointing to the repository. Or, better yet, you can add it in your `.Renviron` with
|
||||||
|
```console
|
||||||
|
user:~$ echo 'ALPHAICON_PATH="path_to_cloned_repository"' >> ~/.Renviron
|
||||||
|
```
|
||||||
|
|
||||||
|
The repository does not contain any data due to its size (10+ GB unpacked); most files in `data/` and `output/` folders are zero-byte placeholders. We provide a <a href="https://drive.google.com/drive/folders/10Tq-b4BVsG3gmq2JVa026Nilzj8eojNB" target="_blank">public Google Drive folder</a> with the populated `data/` and `output/` directories. You may still need to unzip them manually.
|
||||||
|
|
||||||
|
A self-contained example of α-ICON is also available in <a href="https://colab.research.google.com/drive/1AvO8hJzwj2LoKsyxk5LfSWK7LW1U02Mc" target="_blank">Google Colaboratory</a>.
|
||||||
|
|
||||||
|
## Repository structure
|
||||||
|
|
||||||
|
```
|
||||||
|
data/
|
||||||
|
├─uk/ # Data on UK companies and participants
|
||||||
|
| ├ persons-with-significant-control-snapshot-2021-08-02.txt # Source PSC data
|
||||||
|
| ├ BasicCompanyDataAsOneFile-2021-08-01.csv # Source data on live companies in UK
|
||||||
|
| ├ sic_2007_code_list.csv # Standard Industrial Classification codes
|
||||||
|
| ├ psc_snapshot_2021-08-02.rdata # Processed People with Significant Control data
|
||||||
|
| └ uk_basic_companies_data_2021-08-01.rdata # Processed Basic Company data
|
||||||
|
|
|
||||||
|
├─corpwatch_api_tables_csv_14aug21/ # Data from CorpWatch Dump
|
||||||
|
| ├ company_info.csv # Source companies data from SEC filings
|
||||||
|
| ├ cik_name_lookup.csv # Company name variants in SEC filings
|
||||||
|
| └ company_locations.csv # Company locations in SEC filings
|
||||||
|
|
|
||||||
|
code/
|
||||||
|
├─helper_functions/
|
||||||
|
| ├ install_dependencies.r # Installs R dependencies used in the project
|
||||||
|
| └ compute_power_index.r # Computes Mizuno et al. (2020) DPI and NPI
|
||||||
|
|
|
||||||
|
├─data_preparation/
|
||||||
|
| └─uk/
|
||||||
|
| ├ 1a_process_psc_snapshot.r # Prepare source PSC data
|
||||||
|
| ├ 1b_process_companies_data.r # Prepare source data on live companies
|
||||||
|
| ├ 2_psc_snapshot_to_participants_panel.r # PSC data to entity-participant info
|
||||||
|
| └ 3_prepare_affiliated_entities_evaluation_data.r # Process CorpWatch data
|
||||||
|
|
|
||||||
|
├─alphaicon_paper/
|
||||||
|
| ├ 1_compute_alphaicon.ipynb # Jupyter Notebook w. α-ICON (also on Google Colab)
|
||||||
|
| ├ 2_compute_npi_dpi.r # Computation of Direct and Network Power Indices
|
||||||
|
| ├ 3_summary_stat_by_node_type.r # UK PSC network statistics by core/SH/ST/I
|
||||||
|
| ├ 4_illustrate_algorithm.r # Visualise selected networks
|
||||||
|
| ├ 5_algorithm_evaluation.r # Compute recall @ k and l for various algorithms
|
||||||
|
| └ 6_rank_top_holders.r # Examine the rankings of super-holders & Kendall's tau
|
||||||
|
|
|
||||||
|
output/
|
||||||
|
├─uk/
|
||||||
|
| ├ uk_organisations_participants_2021_long_2aug21.csv # Primary ownership data
|
||||||
|
| ├ uk_organisations_participation_graph_core_periphery_membership_6aug21.csv
|
||||||
|
| ├─npi_dpi/ # Mizuno et al. (2020) computation results on UK PSC data
|
||||||
|
| | └─10000iter/
|
||||||
|
| | ├ uk_organisations_participants_2021_long_7sep21_dpi_10000iter.csv # DPI
|
||||||
|
| | └ uk_organisations_participants_2021_long_7sep21_npi_10000iter.csv # NPI
|
||||||
|
| |
|
||||||
|
| ├─transitive/ # Computed α-ICON shares on equity shares or DPI weights
|
||||||
|
| | ├ uk_organisations_transitive_ownership_alpha*_2021_long_2aug21.csv # α = *
|
||||||
|
| | └ uk_organisations_transitive_ownership_alpha*_2021_long_7sep21_dpi_....csv
|
||||||
|
| |
|
||||||
|
└─alphaicon_paper/
|
||||||
|
├ uk_orgs_algorithm_evaluation_recall.csv # Algorithm recall by k
|
||||||
|
├ uk_orgs_algorithm_evaluation_recall_by_pathlength.csv # Algorithm recall by l
|
||||||
|
├ uk_organisations_top100_holders_2021_long_2aug21.csv # Top SH in PSC network
|
||||||
|
├ uk_organisations_top100_holders_diff_npi_dpi_2021_long_2aug21.csv # Top-100 SH
|
||||||
|
| # with the largest difference betw. total DPI and NPI
|
||||||
|
├ uk_organisations_top100_holders_diff_transitive_dpi_2021_long_2aug21.csv
|
||||||
|
| # Top-100 SH with the largest difference betw. total DPI and α-ICON (α=0.999)
|
||||||
|
├ uk_organisations_top100_holders_diff_transitive_npi_2021_long_2aug21.csv
|
||||||
|
| # Top-100 SH with the largest difference betw. total NPI and α-ICON (α=0.999)
|
||||||
|
└ network_examples/ # Visualisations of selected networks
|
||||||
|
```
|
||||||
|
|
||||||
|
We provide an annotated `Makefile` that documents the data analysis in our papers.
|
||||||
|
|
||||||
|
To build the ‘<a href="https://arxiv.org/abs/2109.07181" target="_blank">α-Indirect Control in Onion-like Networks</a>’ paper run `make alphaicon_paper` when in the repository folder.
|
||||||
|
|
||||||
|
Please note that those commands will not produce any publication-ready output files (e.g. tables or figures): the export statements are commented out in the code. Our intention is to make the analysis pipeline transparent to the readers with the aid of `make`:
|
||||||
|
|
||||||
|
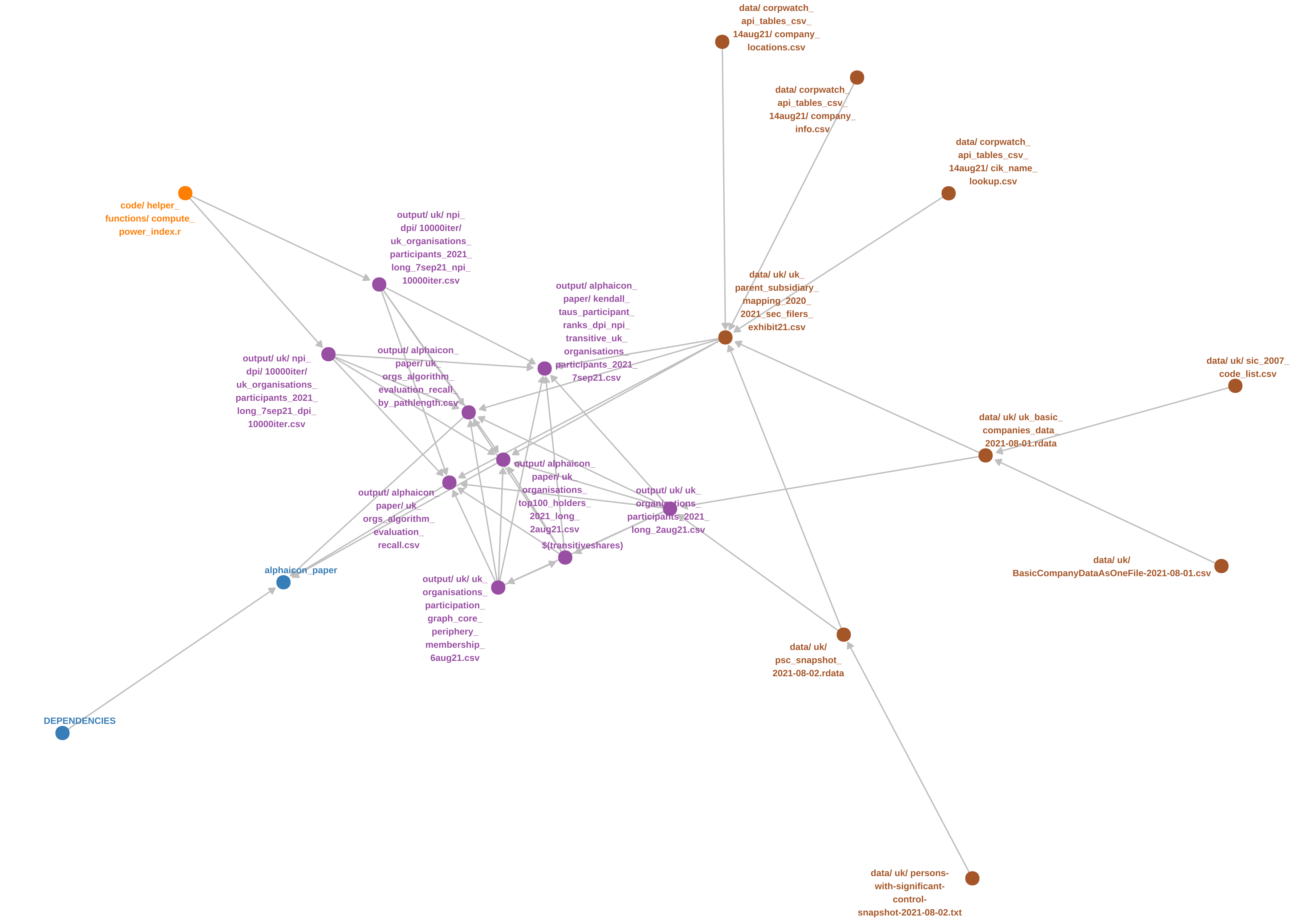
|
||||||
|
|
||||||
|
|
||||||
|
## Licence
|
||||||
|
<a rel="license" href="http://creativecommons.org/licenses/by/4.0/"><img alt="Creative Commons License" style="border-width:0" src="https://i.creativecommons.org/l/by/4.0/88x31.png" /></a><br />
|
||||||
|
Creative Commons License Attribution 4.0 International (CC BY 4.0).
|
||||||
|
|
||||||
|
Copyright © the respective contributors, as shown by the `AUTHORS` file.
|
||||||
|
|
||||||
|
People with Significant Control data is <a href="http://download.companieshouse.gov.uk/en_pscdata.html">distributed</a> by Companies House under <a href="https://www.nationalarchives.gov.uk/doc/open-government-licence/version/3/">Open Government Licence v3.0</a>.
|
||||||
|
|
||||||
|
Free Company Data Product is <a href="http://download.companieshouse.gov.uk/en_output.html">distributed</a> by Companies House under <a href="https://www.nationalarchives.gov.uk/doc/open-government-licence/version/3/">Open Government Licence v3.0</a>.
|
||||||
|
|
||||||
|
|
||||||
|
## Contacts
|
||||||
|
Dmitriy Skougarevskiy, Ph.D.
|
||||||
|
|
||||||
|
dskougarevskiy@eu.spb.ru
|
1349
code/alphaicon_paper/1_compute_alphaicon.ipynb
Normal file
1349
code/alphaicon_paper/1_compute_alphaicon.ipynb
Normal file
File diff suppressed because it is too large
Load diff
63
code/alphaicon_paper/2_compute_npi_dpi.r
Normal file
63
code/alphaicon_paper/2_compute_npi_dpi.r
Normal file
|
@ -0,0 +1,63 @@
|
||||||
|
# This code computes Direct Power Index and
|
||||||
|
# Network Power Index Mizuno, Doi, and Kurizaki (2020, p. 7)
|
||||||
|
# (https://doi.org/10.1371/journal.pone.0237862)
|
||||||
|
# for the UK entities in PSC data
|
||||||
|
library(data.table)
|
||||||
|
|
||||||
|
# NB: there seems to be a bug in multi-threaded data.table group
|
||||||
|
# processing in Windows: https://github.com/Rdatatable/data.table/issues/4294
|
||||||
|
setDTthreads(1)
|
||||||
|
|
||||||
|
set.seed(42)
|
||||||
|
|
||||||
|
# Declare working directory beforehand in an environment variable
|
||||||
|
# ALPHAICON_PATH = "path_to_your_folder"
|
||||||
|
# with the aid of usethis::edit_r_environ()
|
||||||
|
# Restart R session for the changes to make effect
|
||||||
|
setwd(Sys.getenv('ALPHAICON_PATH'))
|
||||||
|
|
||||||
|
# Load the function to compute power index
|
||||||
|
source("code/helper_functions/compute_power_index.r")
|
||||||
|
|
||||||
|
# Load British entities data
|
||||||
|
uk_entities <- fread("output/uk/uk_organisations_participants_2021_long_2aug21.csv", colClasses = c("character", "character", "factor", "numeric"), encoding = "UTF-8", na.strings = "")
|
||||||
|
uk_entities[, kind := NULL ]
|
||||||
|
gc()
|
||||||
|
|
||||||
|
# Remove self-loops and missing equity shares
|
||||||
|
uk_entities <- uk_entities[ participant_id != company_number & !is.na(equity_share) ]
|
||||||
|
|
||||||
|
# Normalize equity to one
|
||||||
|
uk_entities[, equity_share := 100*equity_share/sum(equity_share), by = "company_number" ]
|
||||||
|
|
||||||
|
# Run DPI computation on 10000 iterations
|
||||||
|
tic <- Sys.time()
|
||||||
|
uk_entities_dpi_10000iter <- compute_power_index(uk_entities,
|
||||||
|
participant_var = "participant_id",
|
||||||
|
entity_var = "company_number",
|
||||||
|
weight_var = "equity_share",
|
||||||
|
iterations = 10000,
|
||||||
|
powerindex = "dpi",
|
||||||
|
quota = 50,
|
||||||
|
save_labels = FALSE
|
||||||
|
)
|
||||||
|
toc <- Sys.time()
|
||||||
|
toc - tic # Takes 2.353837 days on Intel Xeon E5-2620
|
||||||
|
fwrite(uk_entities_dpi_10000iter, file = paste0("output/uk/npi_dpi/10000iter/uk_organisations_participants_2021_long_7sep21_dpi_10000iter.csv"), eol = "\n")
|
||||||
|
|
||||||
|
# Run NPI computation on 10000 iterations
|
||||||
|
tic <- Sys.time()
|
||||||
|
uk_entities_npi_10000iter <- compute_power_index(uk_entities,
|
||||||
|
participant_var = "participant_id",
|
||||||
|
entity_var = "company_number",
|
||||||
|
weight_var = "equity_share",
|
||||||
|
iterations = 10000,
|
||||||
|
powerindex = "npi",
|
||||||
|
quota = 50,
|
||||||
|
epsilon = 0.01,
|
||||||
|
iterations_to_discard = 1000,
|
||||||
|
save_labels = FALSE
|
||||||
|
)
|
||||||
|
toc <- Sys.time()
|
||||||
|
toc - tic # Takes 3.755192 days on Intel Xeon E5-2620
|
||||||
|
fwrite(uk_entities_npi_10000iter, file = paste0("output/uk/npi_dpi/10000iter/uk_organisations_participants_2021_long_7sep21_npi_10000iter.csv"), eol = "\n")
|
213
code/alphaicon_paper/3_summary_stat_by_node_type.r
Normal file
213
code/alphaicon_paper/3_summary_stat_by_node_type.r
Normal file
|
@ -0,0 +1,213 @@
|
||||||
|
# This code creates summary statistics
|
||||||
|
# table by node type for the α-ICON paper
|
||||||
|
library(data.table)
|
||||||
|
library(stringi)
|
||||||
|
library(stringr)
|
||||||
|
library(lubridate)
|
||||||
|
library(igraph)
|
||||||
|
library(stargazer)
|
||||||
|
|
||||||
|
# Declare working directory beforehand in an environment variable
|
||||||
|
# ALPHAICON_PATH = "path_to_your_folder"
|
||||||
|
# with the aid of usethis::edit_r_environ()
|
||||||
|
# Restart R session for the changes to make effect
|
||||||
|
setwd(Sys.getenv('ALPHAICON_PATH'))
|
||||||
|
|
||||||
|
############
|
||||||
|
# Data loading
|
||||||
|
|
||||||
|
# Load the active participants snapshot of PSC (prepared by data_preparation/uk/2_psc_snapshot_to_participants_panel.r)
|
||||||
|
psc <- fread("output/uk/uk_organisations_participants_2021_long_2aug21.csv", integer64 = "character", na.strings = "", encoding = "UTF-8")
|
||||||
|
setnames(psc, "equity_share", "share")
|
||||||
|
psc[, share := share/100]
|
||||||
|
psc <- psc[ company_number != participant_id & !is.na(share) ]
|
||||||
|
|
||||||
|
# Load the companies data (prepared by data_preparation/uk/1b_process_companies_data.r)
|
||||||
|
load("data/uk/uk_basic_companies_data_2021-08-01.rdata")
|
||||||
|
|
||||||
|
# Shorter industry names
|
||||||
|
uk_basic_companies_data[ industrysection_1 == "Financial and insurance activities", industrysection_1 := "Finance/Insurance"]
|
||||||
|
uk_basic_companies_data[ industrysection_1 == "Information and communication", industrysection_1 := "IT"]
|
||||||
|
uk_basic_companies_data[ industrysection_1 == "Other service activities", industrysection_1 := "Other service"]
|
||||||
|
uk_basic_companies_data[ industrysection_1 == "Professional, scientific and technical activities", industrysection_1 := "Profess./Science activ"]
|
||||||
|
uk_basic_companies_data[ industrysection_1 == "Mining and Quarrying", industrysection_1 := "Mining/Quarrying"]
|
||||||
|
uk_basic_companies_data[ industrysection_1 == "Mining and Quarrying", industrysection_1 := "Mining/Quarrying"]
|
||||||
|
uk_basic_companies_data[ industrysection_1 == "Real estate activities", industrysection_1 := "Real estate"]
|
||||||
|
uk_basic_companies_data[ industrysection_1 == "Arts, entertainment and recreation", industrysection_1 := "Arts/Entertainment"]
|
||||||
|
uk_basic_companies_data[ industrysection_1 == "Administrative and support service activities", industrysection_1 := "Admin./Support activ"]
|
||||||
|
uk_basic_companies_data[ industrysection_1 == "Accommodation and food service activities", industrysection_1 := "Hospitality/Food"]
|
||||||
|
uk_basic_companies_data[ industrysection_1 == "Human health and social work activities", industrysection_1 := "Health/Social work"]
|
||||||
|
uk_basic_companies_data[ industrysection_1 == "Wholesale and retail trade; repair of motor vehicles and", industrysection_1 := "Trade"]
|
||||||
|
uk_basic_companies_data[ industrysection_1 == "Transportation and storage", industrysection_1 := "Transportation/Storage"]
|
||||||
|
uk_basic_companies_data[ industrysection_1 == "Agriculture, Forestry and Fishing", industrysection_1 := "Agriculture"]
|
||||||
|
uk_basic_companies_data[ industrysection_1 == "Activities of households as employers; undifferentiated", industrysection_1 := "Households as employers"]
|
||||||
|
uk_basic_companies_data[ industrysection_1 == "Water supply, sewerage, waste management and", industrysection_1 := "Water/Sewerage/Waste"]
|
||||||
|
uk_basic_companies_data[ industrysection_1 == "Public administration and defence; compulsory social", industrysection_1 := "Public admin/Defence"]
|
||||||
|
uk_basic_companies_data[ industrysection_1 == "Electricity, gas, steam and air conditioning supply", industrysection_1 := "Electricity/Gas/Steam"]
|
||||||
|
uk_basic_companies_data[ industrysection_1 == "Activities of extraterritorial organisations and", industrysection_1 := "Extraterritorial orgs"]
|
||||||
|
|
||||||
|
# Company age
|
||||||
|
uk_basic_companies_data[, age := as.numeric(ymd("2021-08-01") - IncorporationDate)/31/12 ]
|
||||||
|
|
||||||
|
# Load the transitive ownership of each participant at alpha = 0.999
|
||||||
|
# (prepared by alphaicon_paper/1_compute_alphaicon.ipynb)
|
||||||
|
holders <- fread("output/uk/transitive/uk_organisations_transitive_ownership_alpha0.999_2021_long_2aug21.csv", integer64 = "character", na.strings = "", encoding = "UTF-8", colClasses = c("character", "character", "numeric"))
|
||||||
|
|
||||||
|
# Load the data on graph membership
|
||||||
|
# (prepared by alphaicon_paper/1_compute_alphaicon.ipynb)
|
||||||
|
graph_membership <- fread("output/uk/uk_organisations_participation_graph_core_periphery_membership_6aug21.csv", integer64 = "character", na.strings = "", encoding = "UTF-8", header = T, colClasses = c("numeric", "character", "factor"))
|
||||||
|
graph_membership[, V1 := NULL ]
|
||||||
|
setnames(graph_membership, "company_number/id", "participant_id")
|
||||||
|
gc()
|
||||||
|
|
||||||
|
# Load the data for evaluation of the algorithms (prepared by data_preparation/uk/3_prepare_affiliated_entities_evaluation_data.r)
|
||||||
|
affiliated_entities <- fread("data/uk/uk_parent_subsidiary_mapping_2020_2021_sec_filers_exhibit21.csv", integer64 = "character", na.strings = "", encoding = "UTF-8")
|
||||||
|
# Remove the CorpWatch entity identifiers
|
||||||
|
affiliated_entities[, c("subsidiary_cw_id", "parent_cw_id") := NULL ]
|
||||||
|
|
||||||
|
# Add the data on the shortest paths from parent to subsidiary
|
||||||
|
psc_graph <- graph_from_data_frame(psc[participant_id != company_number & !is.na(share), c("participant_id", "company_number", "share"), with = F], directed = T)
|
||||||
|
|
||||||
|
# Compute all the distances on the graph
|
||||||
|
# from parent companies in the gold standard
|
||||||
|
# data to the subsidiates
|
||||||
|
affiliated_distance_mat <- distances(psc_graph, v = which(V(psc_graph)$name %in% unique(affiliated_entities$participant_id)), to = which(V(psc_graph)$name %in% affiliated_entities$company_number), mode = "out")
|
||||||
|
|
||||||
|
# Arrange them so that the distance is on the main diagonal
|
||||||
|
affiliated_distance <- as.data.table(as.table(affiliated_distance_mat))
|
||||||
|
names(affiliated_distance) <- c("participant_id", "company_number", "path_length")
|
||||||
|
# Remove non-reachable entries
|
||||||
|
affiliated_distance <- affiliated_distance[ is.finite(path_length) ]
|
||||||
|
|
||||||
|
# Add the path lengths to the affiliated entities data
|
||||||
|
affiliated_entities <- merge(affiliated_entities, affiliated_distance, by = c("company_number", "participant_id"), all.x = T, all.y = F, sort = F)
|
||||||
|
#nrow(affiliated_entities[!is.na(path_length)] # 1007
|
||||||
|
|
||||||
|
# Keep only matched orgs
|
||||||
|
affiliated_entities <- affiliated_entities[!is.na(path_length)]
|
||||||
|
|
||||||
|
############
|
||||||
|
# Graph membership data preparation
|
||||||
|
|
||||||
|
# Add the selected characteristics to the graph membership data
|
||||||
|
## Is entity or individual dummy
|
||||||
|
graph_membership[, kind := psc[ match(graph_membership$participant_id, psc$participant_id)]$kind ]
|
||||||
|
graph_membership[is.na(kind) & participant_id %in% psc$company_number, kind := "entity" ]
|
||||||
|
graph_membership <- graph_membership[ !is.na(kind) ]
|
||||||
|
|
||||||
|
graph_membership[, is_person := NA_real_ ]
|
||||||
|
graph_membership[ !is.na(kind), is_person := 0]
|
||||||
|
graph_membership[ kind == "individual", is_person := 1]
|
||||||
|
|
||||||
|
## Industry
|
||||||
|
graph_membership[, industrysection := uk_basic_companies_data[ match(graph_membership$participant_id, uk_basic_companies_data$CompanyNumber)]$industrysection_1 ]
|
||||||
|
## Add individuals to the said list
|
||||||
|
graph_membership[ is_person == 1, industrysection := "Individual"]
|
||||||
|
|
||||||
|
## Company age
|
||||||
|
graph_membership[, age := uk_basic_companies_data[ match(graph_membership$participant_id, uk_basic_companies_data$CompanyNumber)]$age ]
|
||||||
|
|
||||||
|
## Is public company
|
||||||
|
graph_membership[, companytype := uk_basic_companies_data[ match(graph_membership$participant_id, uk_basic_companies_data$CompanyNumber)]$CompanyCategory ]
|
||||||
|
graph_membership[, is_public := NA_real_ ]
|
||||||
|
graph_membership[!is.na(companytype) | is_person == 1, is_public := 0 ]
|
||||||
|
graph_membership[ companytype %in% c("Public Limited Company"), is_public := 1]
|
||||||
|
|
||||||
|
uk_basic_companies_data[, is_public := NA_real_ ]
|
||||||
|
uk_basic_companies_data[!is.na(CompanyCategory), is_public := 0 ]
|
||||||
|
uk_basic_companies_data[ CompanyCategory %in% c("Public Limited Company"), is_public := 1]
|
||||||
|
|
||||||
|
## Is in validation data
|
||||||
|
graph_membership[, in_validation := 0 ]
|
||||||
|
graph_membership[ participant_id %in% affiliated_entities$company_number | participant_id %in% affiliated_entities$participant_id, in_validation := 1 ]
|
||||||
|
|
||||||
|
uk_basic_companies_data[, in_validation := 0 ]
|
||||||
|
uk_basic_companies_data[ CompanyNumber %in% c(affiliated_entities$company_number, affiliated_entities$participant_id), in_validation := 1 ]
|
||||||
|
|
||||||
|
## In- and outdegree
|
||||||
|
psc_graph_degree <- data.table(participant_id = V(psc_graph)$name, indegree = degree(psc_graph, mode = "in"), outdegree = degree(psc_graph, mode = "out"))
|
||||||
|
graph_membership <- merge(graph_membership, psc_graph_degree, by = "participant_id", all.x = T, all.y = F, sort = T)
|
||||||
|
|
||||||
|
uk_basic_companies_data <- merge(uk_basic_companies_data, psc_graph_degree, by.x = "CompanyNumber", by.y = "participant_id", all.x = T, all.y = F, sort = F)
|
||||||
|
|
||||||
|
############
|
||||||
|
# Compute summary stat by graph membership
|
||||||
|
|
||||||
|
## Industry shares by type in PSC graph
|
||||||
|
industry_by_graphtype <- graph_membership[, list(N = .N), by = c("type", "industrysection") ]
|
||||||
|
industry_by_graphtype[ is.na(N), N := 0 ]
|
||||||
|
industry_by_graphtype[, percent := round(100*N/sum(N), 2), by = "type"]
|
||||||
|
# Add the total
|
||||||
|
industry_by_graphtype <- rbind(industry_by_graphtype, data.table(industrysection = "Total", industry_by_graphtype[, list( N = sum(N)), by = "type"], percent = 100))
|
||||||
|
|
||||||
|
## Industry shares in the company universe
|
||||||
|
industry_universe <- uk_basic_companies_data[, list(N_universe = .N), by = c("industrysection_1") ]
|
||||||
|
setnames(industry_universe, "industrysection_1", "industrysection")
|
||||||
|
industry_universe[ is.na(N_universe), N_universe := 0 ]
|
||||||
|
industry_universe[, percent_universe := round(100*N_universe/sum(N_universe), 2)]
|
||||||
|
# Add the total
|
||||||
|
industry_universe <- rbind(industry_universe, data.table( industrysection = "Total", N_universe = sum(industry_universe$N), percent_universe = 100), fill = T)
|
||||||
|
|
||||||
|
## Industry shares in the validation data
|
||||||
|
industry_validation <- graph_membership[ in_validation == 1, list(N_validation = .N), by = c("industrysection") ]
|
||||||
|
industry_validation[ is.na(N_validation), N_validation := 0 ]
|
||||||
|
industry_validation[, percent_validation := round(100*N_validation/sum(N_validation), 2)]
|
||||||
|
# Add the total
|
||||||
|
industry_validation <- rbind(industry_validation, data.table( industrysection = "Total", N_validation = sum(industry_validation$N), percent_validation = 100), fill = T)
|
||||||
|
|
||||||
|
# Compute means of in/outdegree by type, for the entire universe, and for validation data
|
||||||
|
means_by_graphtype <- graph_membership[, lapply(.SD, mean, na.rm = T), .SDcols = c("indegree", "outdegree", "age", "is_public"), by = "type" ]
|
||||||
|
means_by_graphtype[, industrysection := "tempname" ]
|
||||||
|
means_by_graphtype[, c("indegree", "outdegree", "age") := lapply(.SD, round, 2), .SDcols = c("indegree", "outdegree", "age") ]
|
||||||
|
means_by_graphtype[, is_public := round(100*is_public, 2)]
|
||||||
|
temp <- rbind(dcast(means_by_graphtype, industrysection ~ type, value.var = "indegree"), dcast(means_by_graphtype, industrysection ~ type, value.var = "outdegree"))
|
||||||
|
temp <- rbind(temp, dcast(means_by_graphtype, industrysection ~ type, value.var = "age"))
|
||||||
|
temp <- rbind(temp, dcast(means_by_graphtype, industrysection ~ type, value.var = "is_public"))
|
||||||
|
temp$industrysection <- c("Average indegree", "Average outdegree", "Entity age", "Public limited company")
|
||||||
|
names(temp)[2:ncol(temp)] <- paste0("percent_", names(temp)[2:ncol(temp)])
|
||||||
|
means_by_graphtype <- temp
|
||||||
|
|
||||||
|
means_universe <- uk_basic_companies_data[, lapply(.SD, mean, na.rm = T), .SDcols = c("indegree", "outdegree", "age", "is_public")]
|
||||||
|
means_universe[, industrysection := "tempname" ]
|
||||||
|
means_universe[, c("indegree", "outdegree", "age") := lapply(.SD, round, 2), .SDcols = c("indegree", "outdegree", "age") ]
|
||||||
|
means_universe[, is_public := round(100*is_public, 2)]
|
||||||
|
temp <- rbind(dcast(means_universe, industrysection ~ ., value.var = "indegree"), dcast(means_universe, industrysection ~ ., value.var = "outdegree"))
|
||||||
|
temp <- rbind(temp, dcast(means_universe, industrysection ~ ., value.var = "age"))
|
||||||
|
temp <- rbind(temp, dcast(means_universe, industrysection ~ ., value.var = "is_public"))
|
||||||
|
temp$industrysection <- c("Average indegree", "Average outdegree", "Entity age", "Public limited company")
|
||||||
|
setnames(temp, ".", "percent_universe")
|
||||||
|
means_universe <- temp
|
||||||
|
|
||||||
|
means_validation <- graph_membership[ in_validation == 1, lapply(.SD, mean, na.rm = T), .SDcols = c("indegree", "outdegree", "age", "is_public")]
|
||||||
|
means_validation[, industrysection := "tempname" ]
|
||||||
|
means_validation[, c("indegree", "outdegree", "age") := lapply(.SD, round, 2), .SDcols = c("indegree", "outdegree", "age") ]
|
||||||
|
means_validation[, is_public := round(100*is_public, 2)]
|
||||||
|
temp <- rbind(dcast(means_validation, industrysection ~ ., value.var = "indegree"), dcast(means_validation, industrysection ~ ., value.var = "outdegree"))
|
||||||
|
temp <- rbind(temp, dcast(means_validation, industrysection ~ ., value.var = "age"))
|
||||||
|
temp <- rbind(temp, dcast(means_validation, industrysection ~ ., value.var = "is_public"))
|
||||||
|
temp$industrysection <- c("Average indegree", "Average outdegree", "Entity age", "Public limited company")
|
||||||
|
setnames(temp, ".", "percent_validation")
|
||||||
|
means_validation <- temp
|
||||||
|
|
||||||
|
# Long per industry data to wide summary table
|
||||||
|
summary_table <- dcast(industry_by_graphtype, industrysection ~ type, value.var = c("N", "percent"), fill = 0)
|
||||||
|
# Add the data on universe
|
||||||
|
summary_table <- merge(summary_table, industry_universe, by = "industrysection", all = T)
|
||||||
|
summary_table[ is.na(N_universe) & industrysection != "Individual", N_universe := 0]
|
||||||
|
summary_table[ is.na(percent_universe) & industrysection != "Individual", percent_universe := 0]
|
||||||
|
|
||||||
|
# Add the data on validation set
|
||||||
|
summary_table <- merge(summary_table, industry_validation, by = "industrysection", all = T)
|
||||||
|
summary_table[ is.na(N_validation) & industrysection != "Individual", N_validation := 0]
|
||||||
|
summary_table[ is.na(percent_validation) & industrysection != "Individual", percent_validation := 0]
|
||||||
|
|
||||||
|
# Proper order, so that total goes first
|
||||||
|
setorderv(summary_table, "percent_universe", -1)
|
||||||
|
|
||||||
|
# Add the data on degree and other variables to the bottom of the table
|
||||||
|
summary_table <- rbind(summary_table, means_by_graphtype, fill = T)
|
||||||
|
summary_table <- rbind(summary_table, means_universe, fill = T)
|
||||||
|
summary_table <- rbind(summary_table, means_validation, fill = T)
|
||||||
|
|
||||||
|
# Export to .tex table for future editing
|
||||||
|
#stargazer(summary_table, type = "latex", summary = F, out = "output/alphaicon_paper/summary_stat_by_type.tex", float = F, multicolumn = T, digits = 2)
|
257
code/alphaicon_paper/4_illustrate_algorithm.r
Normal file
257
code/alphaicon_paper/4_illustrate_algorithm.r
Normal file
|
@ -0,0 +1,257 @@
|
||||||
|
# This code plots the selected sub-networks
|
||||||
|
# in the PSC data and produces the figures
|
||||||
|
# for the α-ICON paper
|
||||||
|
library(data.table)
|
||||||
|
library(igraph)
|
||||||
|
library(stringi)
|
||||||
|
library(stringr)
|
||||||
|
library(ggplot2)
|
||||||
|
library(ggnetwork)
|
||||||
|
library(ggthemes)
|
||||||
|
library(showtext)
|
||||||
|
|
||||||
|
# Add the font to use
|
||||||
|
font_add_google("Open Sans", "Open Sans")
|
||||||
|
showtext_auto()
|
||||||
|
|
||||||
|
# Declare working directory beforehand in an environment variable
|
||||||
|
# ALPHAICON_PATH = "path_to_your_folder"
|
||||||
|
# with the aid of usethis::edit_r_environ()
|
||||||
|
# Restart R session for the changes to make effect
|
||||||
|
setwd(Sys.getenv('ALPHAICON_PATH'))
|
||||||
|
|
||||||
|
# Load the active participants snapshot of PSC (prepared by data_preparation/uk/2_psc_snapshot_to_participants_panel.r)
|
||||||
|
psc <- fread("output/uk/uk_organisations_participants_2021_long_2aug21.csv", integer64 = "character", na.strings = "", encoding = "UTF-8")
|
||||||
|
|
||||||
|
# Load the companies data (prepared by data_preparation/uk/1b_process_companies_data.r)
|
||||||
|
load("data/uk/uk_basic_companies_data_2021-08-01.rdata")
|
||||||
|
|
||||||
|
# Load the transitive ownership of each participant at alpha = 0.999
|
||||||
|
# (prepared by alphaicon_paper/1_compute_alphaicon.ipynb)
|
||||||
|
holders <- fread("output/uk/transitive/uk_organisations_transitive_ownership_alpha0.999_2021_long_2aug21.csv", integer64 = "character", na.strings = "", encoding = "UTF-8", colClasses = c("character", "character", "numeric"))
|
||||||
|
|
||||||
|
# Load the data on graph membership
|
||||||
|
# (prepared by alphaicon_paper/1_compute_alphaicon.ipynb)
|
||||||
|
graph_membership <- fread("output/uk/uk_organisations_participation_graph_core_periphery_membership_6aug21.csv", integer64 = "character", na.strings = "", encoding = "UTF-8", header = T, colClasses = c("numeric", "character", "factor"))
|
||||||
|
graph_membership[, V1 := NULL ]
|
||||||
|
setnames(graph_membership, "company_number/id", "participant_id")
|
||||||
|
gc()
|
||||||
|
|
||||||
|
# We need to pick up an illustrative organization
|
||||||
|
|
||||||
|
# Ideally this organization should have a small network size
|
||||||
|
# and have at least one entity in graph core
|
||||||
|
|
||||||
|
# Orgs in the core with all other types in the subgraph
|
||||||
|
# and manageable network size
|
||||||
|
temp <- holders[ participant_id %in% graph_membership[ type == "C"]$participant_id ]
|
||||||
|
temp[, company_type := graph_membership[ match(temp$company_number, graph_membership$participant_id) ]$type ]
|
||||||
|
temp[, participant_type := graph_membership[ match(temp$participant_id, graph_membership$participant_id) ]$type ]
|
||||||
|
|
||||||
|
# Devise a set of candidate orgs for illustration
|
||||||
|
# where all organization types are present in the company network
|
||||||
|
candidate_orgs <- temp[, list( company_types = uniqueN(company_type), network_size = uniqueN(company_number)), by = "participant_id"]
|
||||||
|
|
||||||
|
# Consider moderately sized networks with all types of orgs there
|
||||||
|
candidate_ids <- unique(candidate_orgs[ company_types > 2 & network_size <= 30 & network_size > 10]$participant_id)
|
||||||
|
|
||||||
|
# Additionally, consider the largest structure in terms
|
||||||
|
# of graph path from participant to company
|
||||||
|
# This is the result of igraph::farthest_vertices
|
||||||
|
# applied to the psc graph
|
||||||
|
farthest_components <- c("11443992")
|
||||||
|
|
||||||
|
# Also consider participant SEAN DINNEN
|
||||||
|
# he has DPI and NPI of 0 but very large transitivity score
|
||||||
|
sean_dinnen <- "SEAN$MICHAEL$DINNEN$1968$3"
|
||||||
|
|
||||||
|
# Example of long path
|
||||||
|
heidelbergcement <- "HEIDELBERGCEMENT AG$GERMANY$HRB 33082"
|
||||||
|
|
||||||
|
# Finally, consider the organizations with large difference between
|
||||||
|
# the super-holder scores (computed in alphaicon_paper/6_rank_top_holders.r)
|
||||||
|
top100_holders_diff_npi_dpi <- fread("output/alphaicon_paper/uk_organisations_top100_holders_diff_npi_dpi_2021_long_2aug21.csv", encoding = "UTF-8")
|
||||||
|
top100_holders_diff_transitive_dpi <- fread("output/alphaicon_paper/uk_organisations_top100_holders_diff_transitive_dpi_2021_long_2aug21.csv", encoding = "UTF-8")
|
||||||
|
top100_holders_diff_transitive_npi <- fread("output/alphaicon_paper/uk_organisations_top100_holders_diff_transitive_npi_2021_long_2aug21.csv", encoding = "UTF-8")
|
||||||
|
|
||||||
|
# Define the candidates to consider (from affiliated_entities
|
||||||
|
# and other inspection)
|
||||||
|
organizations_of_interest <- unique(c("IHS MARKIT LTD$BERMUDA$48610", "06647317", "11594795", candidate_ids, farthest_components, sean_dinnen))
|
||||||
|
|
||||||
|
# Or specify one organization for the graph in the paper:
|
||||||
|
#organizations_of_interest <- "03782947" # OPUS
|
||||||
|
|
||||||
|
organizations_of_interest <- c(top100_holders_diff_transitive_dpi[1]$participant_id, # SPECSAVERS OPTICAL SUPERSTORES LTD
|
||||||
|
top100_holders_diff_npi_dpi[82]$participant_id, # LENDLEASE INTERNATIONAL PTY LIMITED
|
||||||
|
top100_holders_diff_transitive_dpi[99]$participant_id, # THE BERKELEY GROUP PLC
|
||||||
|
top100_holders_diff_transitive_npi[17]$participant_id) # BAJLINDER$KAUR$BOPARAN$1968$1. NB: her husband RANJIT$SINGH$BOPARAN$1966$8 has alpha-ICON sum 73
|
||||||
|
|
||||||
|
# Iterate over multiple graph candidates
|
||||||
|
for( org_interest in organizations_of_interest ) {
|
||||||
|
|
||||||
|
# Ultimate companies held by this organization
|
||||||
|
holders_subset_ultimate <- holders[ participant_id %in% org_interest ]
|
||||||
|
|
||||||
|
# Consider their total interest in all entities
|
||||||
|
holders_subset <- psc[ participant_id %in% unique(holders_subset_ultimate$company_number) | company_number %in% unique(holders_subset_ultimate$company_number) ]
|
||||||
|
holders_subset <- holders_subset[ !is.na(equity_share)]
|
||||||
|
setnames(holders_subset, "equity_share", "share")
|
||||||
|
|
||||||
|
# Network size
|
||||||
|
#uniqueN(holders_subset$company_number)
|
||||||
|
#uniqueN(holders_subset$participant_id)
|
||||||
|
|
||||||
|
# Add company and participant names and countries of origin
|
||||||
|
holders_subset[, company_name := uk_basic_companies_data[ match(holders_subset$company_number, uk_basic_companies_data$CompanyNumber)]$CompanyName ]
|
||||||
|
#holders_subset[, company_country := uk_basic_companies_data[ match(holders_subset$company_number, uk_basic_companies_data$CompanyNumber)]$CountryOfOrigin ]
|
||||||
|
|
||||||
|
holders_subset[, participant_name := uk_basic_companies_data[ match(holders_subset$participant_id, uk_basic_companies_data$CompanyNumber)]$CompanyName ]
|
||||||
|
#holders_subset[, participant_country := uk_basic_companies_data[ match(holders_subset$participant_id, uk_basic_companies_data$CompanyNumber)]$CountryOfOrigin ]
|
||||||
|
|
||||||
|
# Proper name handling for individuals: first name-surname
|
||||||
|
holders_subset[grepl("$", participant_id, fixed = T) & kind != "individual", participant_name := as.data.table(stri_split_fixed(participant_id, "$", n = 3, simplify = T)[,1])]
|
||||||
|
holders_subset[kind == "individual", participant_name := paste0(stri_split_fixed(participant_id, "$", n = 4, simplify = T)[, 1], " ", stri_split_fixed(participant_id, "$", n = 4, simplify = T)[, 3])]
|
||||||
|
|
||||||
|
#holders_subset[, company_country := gsub("United Kingdom", "UK", company_country) ]
|
||||||
|
#holders_subset[, participant_country := gsub("United Kingdom", "UK", participant_country) ]
|
||||||
|
|
||||||
|
# Are the names unique?
|
||||||
|
#uniqueN(holders_subset$company_name)
|
||||||
|
#uniqueN(holders_subset$participant_name)
|
||||||
|
|
||||||
|
# Add the graph types of organizations
|
||||||
|
holders_subset[, company_type := graph_membership[ match(holders_subset$company_number, graph_membership$participant_id) ]$type ]
|
||||||
|
holders_subset[, participant_type := graph_membership[ match(holders_subset$participant_id, graph_membership$participant_id) ]$type ]
|
||||||
|
holders_subset[, share_range := NA_character_ ]
|
||||||
|
holders_subset[ share == 87.5, share_range := "75-100%"]
|
||||||
|
holders_subset[ share == 62.5, share_range := "50-75%"]
|
||||||
|
holders_subset[ share == 37.5, share_range := "25-50%"]
|
||||||
|
|
||||||
|
## Total number of holdings
|
||||||
|
holdings_count <- holders_subset[, list(count_holdings_total = .N), by = "participant_name"]
|
||||||
|
|
||||||
|
## Total number of holders
|
||||||
|
holders_count <- holders_subset[, list(count_holders_total = .N), by = "company_name"]
|
||||||
|
|
||||||
|
# To igraph object
|
||||||
|
## Create an object with vertex metadata
|
||||||
|
vertex_metadata <- unique(rbind(unique(data.table( name = holders_subset$company_name, type = holders_subset$company_type)),
|
||||||
|
data.table( name = holders_subset$participant_name, type = holders_subset$participant_type)
|
||||||
|
), by = "name")
|
||||||
|
vertex_metadata <- merge(vertex_metadata, holdings_count, by.x = "name", by.y = "participant_name", all.x = T, all.y = F, sort = F)
|
||||||
|
vertex_metadata <- merge(vertex_metadata, holders_count, by.x = "name", by.y = "company_name", all.x = T, all.y = F, sort = F)
|
||||||
|
vertex_metadata[is.na(count_holdings_total), count_holdings_total := 0]
|
||||||
|
vertex_metadata[is.na(count_holders_total), count_holders_total := 0]
|
||||||
|
# NA to mock 0
|
||||||
|
vertex_metadata[count_holdings_total == 0, count_holdings_total := 1]
|
||||||
|
# Remove intermediaries with no holders
|
||||||
|
vertex_metadata <- vertex_metadata[ !(count_holders_total == 0 & type == "I") ]
|
||||||
|
|
||||||
|
## Convert to igraph
|
||||||
|
holders_example_graph <- graph_from_data_frame(holders_subset[participant_name %in% vertex_metadata$name & company_name %in% vertex_metadata$name, c("participant_name", "company_name", "share", "share_range")], directed = T, vertices = vertex_metadata)
|
||||||
|
|
||||||
|
# Line-wrap the name
|
||||||
|
V(holders_example_graph)$name_label <- str_wrap(V(holders_example_graph)$name, 15)
|
||||||
|
|
||||||
|
# Graph to ggnetwork
|
||||||
|
holders_example_net <- ggnetwork(holders_example_graph, layout = with_kk(), arrow.gap = 0.01)
|
||||||
|
|
||||||
|
# Empty name for certain types if network is large
|
||||||
|
if(nrow(holders_example_net) > 150) {
|
||||||
|
|
||||||
|
holders_example_net[!(holders_example_net$type %in% c("SH", "C") | holders_example_net$name %in% c("SPECSAVERS UK HOLDINGS LIMITED", "HANSON PACKED PRODUCTS LIMITED", "HOUSERATE LIMITED", "HANSON LIMITED", "HEIDELBERGCEMENT UK HOLDING LIMITED") ), "name_label"] <- ""
|
||||||
|
|
||||||
|
}
|
||||||
|
|
||||||
|
# Plot the graph
|
||||||
|
holders_example_plot <- ggplot(holders_example_net, aes(x = x, y = y, xend = xend, yend = yend)) +
|
||||||
|
geom_edges(color = "#ebecf0", arrow = arrow(angle = 45, length = unit(5, "pt"), type = "closed"), show.legend = F) +
|
||||||
|
geom_nodes(aes(color = type, size = log(count_holdings_total)), show.legend = T) +
|
||||||
|
{ if( org_interest %in% c("03782947", "PHILIP$AULD$MACTAGGART$1956$2", "BAJLINDER$KAUR$BOPARAN$1968$1")) geom_edgetext(aes(label = share_range), color = "grey25", size = 1.5) } +
|
||||||
|
geom_nodetext_repel(aes(label = name_label, color = type), fontface = "bold", size = 2, show.legend = F, point.padding = 5, max.overlaps = 300) +
|
||||||
|
#{ if(nrow(holders_example_net) > 150) geom_nodetext_repel(aes(color = type), label = "", fontface = "bold", size = 2, show.legend = F, point.padding = 5) } +
|
||||||
|
#{ if(nrow(holders_example_net) <= 150) geom_nodetext_repel(aes(label = name, color = type), fontface = "bold", size = 2, show.legend = F, point.padding = 5) } +
|
||||||
|
scale_size(guide = "none") +
|
||||||
|
scale_color_manual(name = "node type", labels = c("super-holder", "super-target", "intermediary", "core"), values = c("SH" = "#66c2a5", "ST" = "#8da0cb", "I" = "grey", "C" = "#fc8d62")) +
|
||||||
|
guides(color = guide_legend(override.aes = list(size = 5))) +
|
||||||
|
theme_blank() + theme(text = element_text(family = "Open Sans"), legend.position = "bottom", plot.margin = unit(c(0, 0, 0, 0), "cm"))
|
||||||
|
|
||||||
|
# Export to an image
|
||||||
|
graph_file_name <- gsub("[[:punct:]]", "", stri_split_fixed(org_interest, "$", simplify = T)[1,1])
|
||||||
|
|
||||||
|
if( org_interest %in% c("SEAN$MICHAEL$DINNEN$1968$3", "SPECSAVERS OPTICAL SUPERSTORES LTD$UNITED KINGDOM$1721624") ) {
|
||||||
|
|
||||||
|
ggsave(paste0("output/alphaicon_paper/network_examples/uk_example ", graph_file_name, ".pdf"), height = 20, width = 20, scale = 1.2, device = cairo_pdf)
|
||||||
|
|
||||||
|
} else {
|
||||||
|
|
||||||
|
ggsave(paste0("output/alphaicon_paper/network_examples/uk_example ", graph_file_name, ".pdf"), height = 10, width = 10, device = cairo_pdf)
|
||||||
|
|
||||||
|
}
|
||||||
|
|
||||||
|
message(graph_file_name)
|
||||||
|
|
||||||
|
}
|
||||||
|
|
||||||
|
########
|
||||||
|
# Plot the graph with the core components only
|
||||||
|
core <- psc[ participant_id %in% unique(graph_membership[type == "C"]$participant_id) & company_number %in% unique(graph_membership[type == "C"]$participant_id) ]
|
||||||
|
core <- core[ !is.na(equity_share)]
|
||||||
|
setnames(core, "equity_share", "share")
|
||||||
|
|
||||||
|
# Add company and participant names and countries of origin
|
||||||
|
core[, company_name := uk_basic_companies_data[ match(core$company_number, uk_basic_companies_data$CompanyNumber)]$CompanyName ]
|
||||||
|
core[, participant_name := uk_basic_companies_data[ match(core$participant_id, uk_basic_companies_data$CompanyNumber)]$CompanyName ]
|
||||||
|
|
||||||
|
# Proper name handling for individuals: first name-surname
|
||||||
|
core[grepl("$", participant_id, fixed = T) & kind != "individual", participant_name := as.data.table(stri_split_fixed(participant_id, "$", n = 3, simplify = T)[,1])]
|
||||||
|
core[kind == "individual", participant_name := paste0(stri_split_fixed(participant_id, "$", n = 4, simplify = T)[, 1], " ", stri_split_fixed(participant_id, "$", n = 4, simplify = T)[, 3])]
|
||||||
|
|
||||||
|
# Add the graph types of organizations
|
||||||
|
core[, company_type := graph_membership[ match(core$company_number, graph_membership$participant_id) ]$type ]
|
||||||
|
core[, participant_type := graph_membership[ match(core$participant_id, graph_membership$participant_id) ]$type ]
|
||||||
|
core[, share_range := NA_character_ ]
|
||||||
|
core[ share == 87.5, share_range := "75-100%"]
|
||||||
|
core[ share == 62.5, share_range := "50-75%"]
|
||||||
|
core[ share == 37.5, share_range := "25-50%"]
|
||||||
|
|
||||||
|
# Add the total number of companies where core participants
|
||||||
|
## Total number of holdings
|
||||||
|
core_holdings <- holders[participant_id %in% graph_membership[type == "C"]$participant_id, list(count_holdings_total = .N), by = "participant_id"]
|
||||||
|
## Holdings in core
|
||||||
|
core_holdings <- merge(core_holdings, holders[participant_id %in% graph_membership[type == "C"]$participant_id & company_number %in% graph_membership[type == "C"]$participant_id, list(count_holdings_core = .N), by = "participant_id"], by = "participant_id", all = T)
|
||||||
|
|
||||||
|
# To igraph object
|
||||||
|
## Create an object with vertex metadata
|
||||||
|
vertex_metadata <- unique(rbind(unique(data.table(name = core$company_name, type = core$company_type, id = core$company_number)),
|
||||||
|
data.table( name = core$participant_name, type = core$participant_type, id = core$participant_id)
|
||||||
|
), by = "name")
|
||||||
|
|
||||||
|
# Add the counts of holdings
|
||||||
|
vertex_metadata <- merge(vertex_metadata, core_holdings, by.x = "id", by.y = "participant_id", all = T)
|
||||||
|
vertex_metadata[, id := NULL]
|
||||||
|
|
||||||
|
# Keep only non-trivial components
|
||||||
|
vertex_metadata <- vertex_metadata[ count_holdings_core > 2 ]
|
||||||
|
|
||||||
|
## Convert to igraph
|
||||||
|
core_graph <- graph_from_data_frame(core[ participant_name %in% vertex_metadata$name | company_name %in% vertex_metadata$name, c("participant_name", "company_name", "share", "share_range")], directed = T, vertices = vertex_metadata)
|
||||||
|
|
||||||
|
# Line-wrap the name
|
||||||
|
V(core_graph)$name_label <- str_wrap(V(core_graph)$name, 15)
|
||||||
|
|
||||||
|
# Graph to ggnetwork
|
||||||
|
core_net <- ggnetwork(core_graph, layout = with_fr(), arrow.gap = 0.01)
|
||||||
|
|
||||||
|
# Plot the graph
|
||||||
|
core_plot <- ggplot(core_net, aes(x = x, y = y, xend = xend, yend = yend)) +
|
||||||
|
geom_edges(color = "grey", arrow = arrow(angle = 45, length = unit(3, "pt"), type = "closed"), show.legend = F) +
|
||||||
|
geom_nodes(aes(color = type, size = count_holdings_total), show.legend = T) +
|
||||||
|
geom_edgetext(aes(label = share_range), color = "grey25", size = 1) +
|
||||||
|
geom_nodetext_repel(aes(label = name_label, color = type), fontface = "bold", size = 2, show.legend = F, point.padding = 5) +
|
||||||
|
scale_size(guide = "none") +
|
||||||
|
scale_color_manual(name = "node type", labels = c("super-holder", "super-target", "intermediary", "core"), values = c("SH" = "#66c2a5", "ST" = "#8da0cb", "I" = "grey", "C" = "#fc8d62")) +
|
||||||
|
guides(color = guide_legend(override.aes = list(size = 5))) +
|
||||||
|
theme_blank() + theme(text = element_text(family = "Open Sans"), legend.position = "none", plot.margin = unit(c(0, 0, 0, 0), "cm"))
|
||||||
|
|
||||||
|
ggsave(paste0("output/network_examples/core_plot.pdf"), height = 10, width = 10, scale = 1.1, device = cairo_pdf)
|
412
code/alphaicon_paper/5_algorithm_evaluation.r
Normal file
412
code/alphaicon_paper/5_algorithm_evaluation.r
Normal file
|
@ -0,0 +1,412 @@
|
||||||
|
# This code evaluates various algorithms
|
||||||
|
# for ultimate owner identification
|
||||||
|
|
||||||
|
library(data.table)
|
||||||
|
library(stringi)
|
||||||
|
library(stringr)
|
||||||
|
library(igraph)
|
||||||
|
|
||||||
|
# Declare working directory beforehand in an environment variable
|
||||||
|
# ALPHAICON_PATH = "path_to_your_folder"
|
||||||
|
# with the aid of usethis::edit_r_environ()
|
||||||
|
# Restart R session for the changes to make effect
|
||||||
|
setwd(Sys.getenv('ALPHAICON_PATH'))
|
||||||
|
|
||||||
|
############
|
||||||
|
# Load and prepare the data
|
||||||
|
|
||||||
|
# Load the active participants snapshot of PSC (prepared by data_preparation/uk/2_psc_snapshot_to_participants_panel.r)
|
||||||
|
psc <- fread("output/uk/uk_organisations_participants_2021_long_2aug21.csv", integer64 = "character", na.strings = "", encoding = "UTF-8")
|
||||||
|
|
||||||
|
# Load the DPI of each participant after 10000 iterations (computed by alphaicon_paper/2_compute_npi_dpi.r)
|
||||||
|
holders_dpi <- fread("output/uk/npi_dpi/10000iter/uk_organisations_participants_2021_long_7sep21_dpi_10000iter.csv", integer64 = "character", na.strings = "", encoding = "UTF-8")
|
||||||
|
setnames(holders_dpi, c("entity", "participant", "dpi"), c("company_number", "participant_id", "share"))
|
||||||
|
holders_dpi[, times_pivotal := NULL ]
|
||||||
|
|
||||||
|
# Load the NPI of each participant after 10000 iterations (computed by alphaicon_paper/2_compute_npi_dpi.r)
|
||||||
|
holders_npi <- fread("output/uk/npi_dpi/10000iter/uk_organisations_participants_2021_long_7sep21_npi_10000iter.csv", integer64 = "character", na.strings = "", encoding = "UTF-8")
|
||||||
|
setnames(holders_npi, c("entity", "participant", "npi"), c("company_number", "participant_id", "share"))
|
||||||
|
holders_npi[, times_pivotal := NULL ]
|
||||||
|
|
||||||
|
# Load the transitive ownership data at various alpha
|
||||||
|
# (prepared by alphaicon_paper/1_compute_alphaicon.ipynb)
|
||||||
|
alphas <- c("0.0", "0.1", "0.2", "0.3", "0.4", "0.5", "0.6", "0.7", "0.8", "0.9", "0.999")
|
||||||
|
|
||||||
|
for( a in alphas) {
|
||||||
|
|
||||||
|
# Transitive ownership on equity shares
|
||||||
|
temp1 <- fread(paste0("output/uk/transitive/uk_organisations_transitive_ownership_alpha", a, "_2021_long_2aug21.csv"), integer64 = "character", na.strings = "", encoding = "UTF-8", colClasses = c("character", "character", "numeric"))
|
||||||
|
|
||||||
|
assign(paste0("holders_transitive_alpha", a), temp1)
|
||||||
|
|
||||||
|
# Transitive ownership on DPI weights
|
||||||
|
temp2 <- fread(paste0("output/uk/transitive/uk_organisations_transitive_ownership_alpha", a, "_2021_long_7sep21_dpi_10000iter.csv"), integer64 = "character", na.strings = "", encoding = "UTF-8", colClasses = c("character", "character", "numeric"))
|
||||||
|
|
||||||
|
assign(paste0("holders_transitivedpi_alpha", a), temp2)
|
||||||
|
|
||||||
|
rm(temp1); rm(temp2)
|
||||||
|
|
||||||
|
}
|
||||||
|
|
||||||
|
# Load the data on graph membership
|
||||||
|
# (prepared by alphaicon_paper/1_compute_alphaicon.ipynb)
|
||||||
|
graph_membership <- fread("output/uk/uk_organisations_participation_graph_core_periphery_membership_6aug21.csv", integer64 = "character", na.strings = "", encoding = "UTF-8", header = T, colClasses = c("numeric", "character", "factor"))
|
||||||
|
graph_membership[, V1 := NULL ]
|
||||||
|
setnames(graph_membership, "company_number/id", "participant_id")
|
||||||
|
|
||||||
|
# Load the data for evaluation of the algorithms (prepared by data_preparation/uk/3_prepare_affiliated_entities_evaluation_data.r)
|
||||||
|
affiliated_entities <- fread("data/uk/uk_parent_subsidiary_mapping_2020_2021_sec_filers_exhibit21.csv", integer64 = "character", na.strings = "", encoding = "UTF-8")
|
||||||
|
# Remove the CorpWatch entity identifiers
|
||||||
|
affiliated_entities[, c("subsidiary_cw_id", "parent_cw_id") := NULL ]
|
||||||
|
|
||||||
|
# Add the data on the shortest paths from parent to subsidiary
|
||||||
|
psc_graph <- graph_from_data_frame(psc[participant_id != company_number & !is.na(equity_share), c("participant_id", "company_number", "equity_share"), with = F], directed = T)
|
||||||
|
|
||||||
|
# Compute all the distances on the graph
|
||||||
|
# from parent companies in the gold standard
|
||||||
|
# data to the subsidiates
|
||||||
|
affiliated_distance_mat <- distances(psc_graph, v = which(V(psc_graph)$name %in% unique(affiliated_entities$participant_id)), to = which(V(psc_graph)$name %in% affiliated_entities$company_number), mode = "out")
|
||||||
|
|
||||||
|
# Arrange them so that the distance is on the main diagonal
|
||||||
|
affiliated_distance <- as.data.table(as.table(affiliated_distance_mat))
|
||||||
|
names(affiliated_distance) <- c("participant_id", "company_number", "path_length")
|
||||||
|
# Remove non-reachable entries
|
||||||
|
affiliated_distance <- affiliated_distance[ is.finite(path_length) ]
|
||||||
|
|
||||||
|
# Add the path lengths to the affiliated entities data
|
||||||
|
affiliated_entities <- merge(affiliated_entities, affiliated_distance, by = c("company_number", "participant_id"), all.x = T, all.y = F, sort = F)
|
||||||
|
#affiliated_entities[, .N, by = "path_length"]
|
||||||
|
#nrow(affiliated_entities[!is.na(path_length)] # 1007
|
||||||
|
|
||||||
|
# Keep only affiliated entities reachable on the PSC graph
|
||||||
|
affiliated_entities <- affiliated_entities[ !is.na(path_length) ]
|
||||||
|
|
||||||
|
# List all holder objects that are loaded in the memory
|
||||||
|
holder_objects <- paste0("holders_", c("dpi", "npi", paste0("transitive_alpha", alphas), paste0("transitivedpi_alpha", alphas)))
|
||||||
|
|
||||||
|
############
|
||||||
|
# Subset holder objects
|
||||||
|
|
||||||
|
# Keep only the affiliated entities in the holder objects (to speed up the computation time)
|
||||||
|
for(obj in holder_objects) {
|
||||||
|
|
||||||
|
assign(obj, get(obj)[ company_number %in% affiliated_entities$company_number | company_number %in% affiliated_entities$participant_id | participant_id %in% affiliated_entities$company_number | participant_id %in% affiliated_entities$participant_id])
|
||||||
|
|
||||||
|
# Add the entity type for objects
|
||||||
|
assign(obj, merge(get(obj), graph_membership, by = "participant_id", all.x = T, all.y = F, sort = F))
|
||||||
|
|
||||||
|
}
|
||||||
|
|
||||||
|
# Add the ranks
|
||||||
|
for(obj in holder_objects) {
|
||||||
|
|
||||||
|
# Sort by descending share within companies
|
||||||
|
setorderv(get(obj), c("company_number", "share"), c(1, -1))
|
||||||
|
|
||||||
|
# Add the rank
|
||||||
|
get(obj)[, rank := 1:.N, by = "company_number"]
|
||||||
|
|
||||||
|
# Devise another rank
|
||||||
|
# that ranks participants within types
|
||||||
|
get(obj)[, rankbygraphtype := 1:.N, by = c("company_number", "type")]
|
||||||
|
|
||||||
|
}
|
||||||
|
|
||||||
|
# Debug: holders_transitive_alpha0.5[company_number == "SL033942"]
|
||||||
|
|
||||||
|
# Create a single object with ranks under different algorithms
|
||||||
|
algorithms <- c("dpi", "npi", paste0("transitive_alpha", alphas), paste0("transitivedpi_alpha", alphas))
|
||||||
|
|
||||||
|
# Start with the actual participants data (for the baseline algorithm)
|
||||||
|
holders_algorithm_ranks <- psc[ company_number %in% affiliated_entities$company_number, c("company_number", "participant_id", "equity_share"), with = F]
|
||||||
|
holders_algorithm_ranks <- holders_algorithm_ranks[ !is.na(equity_share) ]
|
||||||
|
|
||||||
|
# For the baseline algorithm simply take the equity share
|
||||||
|
setorderv(holders_algorithm_ranks, c("company_number", "equity_share"), c(1, -1))
|
||||||
|
holders_algorithm_ranks[, rank_baseline := 1:.N, by = "company_number" ]
|
||||||
|
holders_algorithm_ranks[, equity_share := NULL ]
|
||||||
|
|
||||||
|
# For the remaining ranks query the holders object
|
||||||
|
for(alg in algorithms) {
|
||||||
|
|
||||||
|
cols_to_keep <- intersect(names(get(paste0("holders_", alg))), c("company_number", "participant_id", "rank", "rankbygraphtype"))
|
||||||
|
|
||||||
|
holders_algorithm_ranks <- merge(holders_algorithm_ranks, get(paste0("holders_", alg))[, c(cols_to_keep), with = F], by = c("company_number", "participant_id"), all.x = T, all.y = T, sort = F)
|
||||||
|
setnames(holders_algorithm_ranks, "rank", paste0("rank_", alg))
|
||||||
|
setnames(holders_algorithm_ranks, "rankbygraphtype", paste0("rankbygraphtype_", alg), skip_absent = T)
|
||||||
|
|
||||||
|
message(alg)
|
||||||
|
|
||||||
|
}
|
||||||
|
|
||||||
|
# Add the participant type in graph
|
||||||
|
holders_algorithm_ranks[, type := graph_membership[ match(holders_algorithm_ranks$participant_id, graph_membership$participant_id)]$type ]
|
||||||
|
|
||||||
|
# What rank do the actual super-holders from the affiliated entities evaluation data
|
||||||
|
# take under each algorithm
|
||||||
|
holders_algorithm_ranks_affiliated <- holders_algorithm_ranks[ company_number %in% affiliated_entities$company_number]
|
||||||
|
|
||||||
|
# Add the ground truth participant id
|
||||||
|
holders_algorithm_ranks_affiliated <- merge(holders_algorithm_ranks_affiliated, affiliated_entities[, -"path_length"], by = c("company_number"), all = T, sort = F)
|
||||||
|
|
||||||
|
# NB: We have duplicates:
|
||||||
|
# holders_algorithm_ranks_affiliated[ company_number == "00906355"]
|
||||||
|
|
||||||
|
# Debug:
|
||||||
|
#holders_algorithm_ranks[ company_number == "00027883"]
|
||||||
|
#holders_algorithm_ranks_affiliated[ company_number == "00027883"]
|
||||||
|
|
||||||
|
# Add the path lengths to the data
|
||||||
|
holders_algorithm_ranks_affiliated <- merge(holders_algorithm_ranks_affiliated, affiliated_entities, by.x = c("company_number", "participant_id.y"), by.y = c("company_number", "participant_id"), sort = F, all.x = T, all.y = F)
|
||||||
|
|
||||||
|
# What rank does the truth take under each algorithm
|
||||||
|
algorithms <- unique(c("baseline", algorithms))
|
||||||
|
|
||||||
|
for(alg in algorithms) {
|
||||||
|
|
||||||
|
holders_algorithm_ranks_affiliated[, paste0("rank_true_", alg) := NA_real_ ]
|
||||||
|
|
||||||
|
holders_algorithm_ranks_affiliated[participant_id.x == participant_id.y, paste0("rank_true_", alg) := get(paste0("rank_", alg)) ]
|
||||||
|
|
||||||
|
# Add the rank when we consider only super-holders
|
||||||
|
if( !grepl("baseline", alg) ) {
|
||||||
|
|
||||||
|
holders_algorithm_ranks_affiliated[ participant_id.x == participant_id.y & type == "SH", paste0("rankonlysuperholder_true_", alg) := get(paste0("rankbygraphtype_", alg))]
|
||||||
|
|
||||||
|
# Restore the original value if no rank for super-holder
|
||||||
|
holders_algorithm_ranks_affiliated[ is.na(get(paste0("rankonlysuperholder_true_", alg))) , paste0("rankonlysuperholder_true_", alg) := get(paste0("rank_true_", alg)) ]
|
||||||
|
|
||||||
|
|
||||||
|
}
|
||||||
|
|
||||||
|
}
|
||||||
|
|
||||||
|
# Aggregate at company level
|
||||||
|
cols_of_interest <- names(holders_algorithm_ranks_affiliated)[ grepl("rank.*_true_", names(holders_algorithm_ranks_affiliated))]
|
||||||
|
cols_of_interest <- c(cols_of_interest, "path_length")
|
||||||
|
|
||||||
|
affiliated_evaluation_results <- holders_algorithm_ranks_affiliated[, lapply(.SD, min, na.rm = T), .SDcols = cols_of_interest, by = "company_number"]
|
||||||
|
affiliated_evaluation_results[, c(cols_of_interest) := lapply(.SD, function(x) ifelse(is.finite(x), x, NA)), .SDcols = cols_of_interest]
|
||||||
|
|
||||||
|
# With the above min-aggregation we resolve the issue with duplicates
|
||||||
|
# affiliated_evaluation_results[ company_number == "00906355"]
|
||||||
|
|
||||||
|
# Compute recall @ different k for algorithms
|
||||||
|
## Init an object to store the recall
|
||||||
|
algorithm_recall <- data.table()
|
||||||
|
|
||||||
|
## Init an object to store the recall at different path lengths
|
||||||
|
algorithm_recall_by_pathlength <- data.table()
|
||||||
|
|
||||||
|
path_lengths <- unique(holders_algorithm_ranks_affiliated$path_length)
|
||||||
|
path_lengths <- path_lengths[ order(path_lengths) ]
|
||||||
|
path_lengths <- path_lengths[ !is.na(path_lengths) ]
|
||||||
|
|
||||||
|
for(k in 1:10) {
|
||||||
|
|
||||||
|
for( alg in algorithms ) {
|
||||||
|
|
||||||
|
# How many organisations have the true participant out of all k participants
|
||||||
|
recall <- nrow(affiliated_evaluation_results[ get(paste0("rank_true_", alg)) <= k ])/nrow(affiliated_evaluation_results)
|
||||||
|
|
||||||
|
algorithm_recall <- rbind(algorithm_recall, data.table(algorithm = alg, k = k, superholderpriority = 0, recall = recall), fill = T)
|
||||||
|
|
||||||
|
# Special recall considering only super-holders
|
||||||
|
if( !grepl("baseline", alg) ) {
|
||||||
|
|
||||||
|
recallsh <- nrow(affiliated_evaluation_results[ get(paste0("rankonlysuperholder_true_", alg)) <= k ])/nrow(affiliated_evaluation_results)
|
||||||
|
|
||||||
|
algorithm_recall <- rbind(algorithm_recall, data.table(algorithm = alg, k = k, superholderpriority = 1, recall = recallsh), fill = T)
|
||||||
|
|
||||||
|
}
|
||||||
|
|
||||||
|
# Same for each path lengths
|
||||||
|
for( p in path_lengths ) {
|
||||||
|
|
||||||
|
recallpath <- nrow(affiliated_evaluation_results[ get(paste0("rank_true_", alg)) <= k & path_length == p ])/nrow(affiliated_evaluation_results[ path_length == p | is.na(path_length) ])
|
||||||
|
|
||||||
|
|
||||||
|
algorithm_recall_by_pathlength <- rbind(algorithm_recall_by_pathlength, data.table(algorithm = alg, k = k, superholderpriority = 0, path = p, recall = recallpath), fill = T)
|
||||||
|
|
||||||
|
if( !grepl("baseline", alg) ) {
|
||||||
|
|
||||||
|
recallpathsh <- nrow(affiliated_evaluation_results[ get(paste0("rankonlysuperholder_true_", alg)) <= k & path_length == p ])/nrow(affiliated_evaluation_results[ path_length == p | is.na(path_length) ] )
|
||||||
|
|
||||||
|
algorithm_recall_by_pathlength <- rbind(algorithm_recall_by_pathlength, data.table(algorithm = alg, k = k, superholderpriority = 1, path = p, recall = recallpathsh), fill = T)
|
||||||
|
|
||||||
|
}
|
||||||
|
|
||||||
|
}
|
||||||
|
|
||||||
|
}
|
||||||
|
|
||||||
|
}
|
||||||
|
|
||||||
|
# Save point
|
||||||
|
fwrite(algorithm_recall, file = "output/alphaicon_paper/uk_orgs_algorithm_evaluation_recall.csv")
|
||||||
|
fwrite(algorithm_recall_by_pathlength, file = "output/alphaicon_paper/uk_orgs_algorithm_evaluation_recall_by_pathlength.csv")
|
||||||
|
|
||||||
|
# Manual inspection for tables
|
||||||
|
#algorithm_recall[ algorithm %in% c("baseline", "dpi", "npi", "transitive_alpha0.0", "transitive_alpha0.999", "transitivedpi_alpha0.999") & k == 1 ]
|
||||||
|
#algorithm_recall[ algorithm %in% c("baseline", "dpi", "npi", "transitive_alpha0.0", "transitive_alpha0.999", "transitivedpi_alpha0.999") & k == 3 ]
|
||||||
|
#algorithm_recall[ algorithm %in% c("baseline", "dpi", "npi", "transitive_alpha0.0", "transitive_alpha0.999", "transitivedpi_alpha0.999") & k == 5 ]
|
||||||
|
#algorithm_recall[ algorithm %in% c("baseline", "dpi", "npi", "transitive_alpha0.0", "transitive_alpha0.999", "transitivedpi_alpha0.999") & k == 10 ]
|
||||||
|
|
||||||
|
#algorithm_recall_by_pathlength[ algorithm %in% c("baseline", "dpi", "npi", "transitive_alpha0.0", "transitive_alpha0.999", "transitivedpi_alpha0.999") & k == 1 & path == 1 ]
|
||||||
|
#algorithm_recall_by_pathlength[ algorithm %in% c("baseline", "dpi", "npi", "transitive_alpha0.0", "transitive_alpha0.999", "transitivedpi_alpha0.999") & k == 1 & path == 2 ]
|
||||||
|
#algorithm_recall_by_pathlength[ algorithm %in% c("baseline", "dpi", "npi", "transitive_alpha0.0", "transitive_alpha0.999", "transitivedpi_alpha0.999") & k == 1 & path == 3 ]
|
||||||
|
#algorithm_recall_by_pathlength[ algorithm %in% c("baseline", "dpi", "npi", "transitive_alpha0.0", "transitive_alpha0.999", "transitivedpi_alpha0.999") & k == 1 & path == 4 ]
|
||||||
|
#algorithm_recall_by_pathlength[ algorithm %in% c("baseline", "dpi", "npi", "transitive_alpha0.0", "transitive_alpha0.999", "transitivedpi_alpha0.999") & k == 1 & path == 5 ]
|
||||||
|
#algorithm_recall_by_pathlength[ algorithm %in% c("baseline", "dpi", "npi", "transitive_alpha0.0", "transitive_alpha0.999", "transitivedpi_alpha0.999") & k == 1 & path == 10 ]
|
||||||
|
#algorithm_recall_by_pathlength[ algorithm %in% c("baseline", "dpi", "npi", "transitive_alpha0.0", "transitive_alpha0.999", "transitivedpi_alpha0.999") & k == 1 & path == 12 ]
|
||||||
|
|
||||||
|
|
||||||
|
############
|
||||||
|
# Plot the results
|
||||||
|
|
||||||
|
library(data.table)
|
||||||
|
library(ggplot2)
|
||||||
|
library(ggthemes)
|
||||||
|
library(ggrepel)
|
||||||
|
library(stringi)
|
||||||
|
library(showtext)
|
||||||
|
|
||||||
|
setwd(Sys.getenv('ALPHAICON_PATH'))
|
||||||
|
|
||||||
|
# Add the font to use
|
||||||
|
font_add_google("Open Sans", "Open Sans")
|
||||||
|
showtext_auto()
|
||||||
|
|
||||||
|
# Load the files with evaluation results
|
||||||
|
algorithm_recall <- fread("output/alphaicon_paper/uk_orgs_algorithm_evaluation_recall.csv", encoding = "UTF-8")
|
||||||
|
algorithm_recall_by_pathlength <- fread("output/alphaicon_paper/uk_orgs_algorithm_evaluation_recall_by_pathlength.csv", encoding = "UTF-8")
|
||||||
|
|
||||||
|
for(obj in c("algorithm_recall", "algorithm_recall_by_pathlength") ) {
|
||||||
|
|
||||||
|
# Column marking whether we use DPI weights
|
||||||
|
get(obj)[, dpi_weights := 0]
|
||||||
|
get(obj)[grepl("dpi", algorithm, ignore.case = T), dpi_weights := 1]
|
||||||
|
|
||||||
|
# Shorter names
|
||||||
|
get(obj)[, algorithm := gsub("transitive.*_alpha", "α=", algorithm)]
|
||||||
|
get(obj)[, algorithm := gsub("transitivedpi_alpha", "α=", algorithm)]
|
||||||
|
|
||||||
|
# Extract alpha values from the name
|
||||||
|
get(obj)[, alpha := as.numeric(stri_split_fixed(algorithm, "α=", n = 2, simplify = T)[,2]) ]
|
||||||
|
|
||||||
|
# Add the superholder mark to corresponding algorithm names
|
||||||
|
get(obj)[ superholderpriority == 1, algorithm := paste0(algorithm, " SH")]
|
||||||
|
|
||||||
|
# Add the DPI weight mark to corresponding algorithm names
|
||||||
|
get(obj)[ dpi_weights == 1 & grepl("α", algorithm), algorithm := paste0(algorithm, " DPI")]
|
||||||
|
|
||||||
|
# Certain names to upper case
|
||||||
|
get(obj)[, algorithm := gsub("dpi", "DPI", algorithm) ]
|
||||||
|
get(obj)[, algorithm := gsub("npi", "NPI", algorithm) ]
|
||||||
|
|
||||||
|
}
|
||||||
|
|
||||||
|
########
|
||||||
|
# Produce the evaluation plots (overall)
|
||||||
|
|
||||||
|
# One subset for display: only the best performing α-ICON versus other algorithms
|
||||||
|
algorithm_recall_otheralgs <- algorithm_recall[ algorithm %in% c("DPI", "NPI", "α=0.999", "α=0.999 SH")]
|
||||||
|
|
||||||
|
## Other algorithms vs best and worst-performing α-ICON algorithm
|
||||||
|
recall_plot <- ggplot(aes(x = k, y = recall, group = algorithm, color = algorithm), data = algorithm_recall_otheralgs) +
|
||||||
|
geom_line(size = 1.5, alpha = 1) +
|
||||||
|
geom_point(size = 2) +
|
||||||
|
scale_x_continuous(breaks = 1:10) +
|
||||||
|
geom_text(aes(label = algorithm, colour = algorithm, x = k-1, y = recall), data = algorithm_recall_otheralgs[k == 1 & algorithm == "DPI"], size = 8, hjust = 0.3) +
|
||||||
|
geom_text(aes(label = algorithm, colour = algorithm, x = k-1, y = recall), data = algorithm_recall_otheralgs[k == 1 & algorithm == "α=0.999"], size = 8, hjust = 0.7) +
|
||||||
|
geom_text(aes(label = algorithm, colour = algorithm, x = k-1, y = recall), data = algorithm_recall_otheralgs[k == 1 & algorithm == "NPI"], size = 8, hjust = 0.3) +
|
||||||
|
geom_text(aes(label = algorithm, colour = algorithm, x = k-1, y = recall), data = algorithm_recall_otheralgs[k == 1 & algorithm == "α=0.999 SH"], size = 8, hjust = 0.5, vjust = -0.6) +
|
||||||
|
#geom_text_repel(aes(label = algorithm, colour = algorithm, x = k-1, y = recall), data = algorithm_recall_otheralgs[k == 1], size = 8, hjust = 0.5) +
|
||||||
|
labs(y = expression("Recall @ "~italic("k")), x = expression(italic("k"))) +
|
||||||
|
#scale_colour_brewer(type = "qual", palette = 2) +
|
||||||
|
scale_color_manual(name = "", values = c("#b2df8a", "#33a02c", "#a6cee3", "#1f78b4")) +
|
||||||
|
expand_limits(x = c(-1.5, 10)) +
|
||||||
|
theme_minimal() + theme(legend.position = "none", text = element_text(size = 18, family = "Open Sans"), panel.grid.major = element_blank(), panel.grid.minor = element_blank())
|
||||||
|
|
||||||
|
ggsave(recall_plot, file = "output/alphaicon_paper/recall_plot.pdf", width = 12, height = 9, device = cairo_pdf, scale = 0.6)
|
||||||
|
|
||||||
|
# Another subset for display: only α-ICON under different alphas
|
||||||
|
algorithm_recall_onlyalphas <- algorithm_recall[ alpha > 0 & superholderpriority == 1 & dpi_weights == 0]
|
||||||
|
|
||||||
|
# Group the duplicated values of recall at alphas
|
||||||
|
alpha_group <- algorithm_recall_onlyalphas[ k == 1, list(alphagroup = .GRP), by = "recall"]
|
||||||
|
alpha_group <- merge(alpha_group, algorithm_recall_onlyalphas[ k == 1, c("alpha", "recall")], by = "recall")
|
||||||
|
alpha_group[, recall := NULL]
|
||||||
|
# Generate the label text for alpha group
|
||||||
|
alpha_group[, minalpha := min(alpha), by = "alphagroup"]
|
||||||
|
alpha_group[, maxalpha := max(alpha), by = "alphagroup"]
|
||||||
|
|
||||||
|
alpha_group[ minalpha == maxalpha, alphagrouplabel := as.character(round(alpha, 1))]
|
||||||
|
alpha_group[ minalpha != maxalpha, alphagrouplabel := as.character(paste0(round(minalpha, 1), "-", round(maxalpha, 3)))]
|
||||||
|
alpha_group[, alphagrouplabel := paste0("α=", alphagrouplabel)]
|
||||||
|
|
||||||
|
algorithm_recall_onlyalphas[, alphagroup := alpha_group[match(algorithm_recall_onlyalphas$alpha, alpha_group$alpha)]$alphagrouplabel]
|
||||||
|
|
||||||
|
# Make alpha groups unique
|
||||||
|
algorithm_recall_onlyalphas <- unique(algorithm_recall_onlyalphas, by = c("alphagroup", "k"))
|
||||||
|
|
||||||
|
## Other algorithms vs best and worst-performing transitive algorithm
|
||||||
|
recall_plot_alphas <- ggplot(aes(x = k, y = recall, group = alphagroup, color = as.factor(alpha)), data = unique(algorithm_recall_onlyalphas)) +
|
||||||
|
geom_line(size = 1.5, alpha = 1) +
|
||||||
|
geom_point(size = 2) +
|
||||||
|
scale_x_continuous(breaks = 1:10) +
|
||||||
|
geom_text(aes(label = alphagroup, colour = as.factor(alpha), group = alphagroup, x = k-2.5, y = recall), size = 8, data = algorithm_recall_onlyalphas[k == 4 & alpha == 0.1 ], hjust = 0.3) +
|
||||||
|
geom_text(aes(label = alphagroup, colour = as.factor(alpha), group = alphagroup, x = k-2.5, y = recall), size = 8, data = algorithm_recall_onlyalphas[k == 2 & alpha == 0.5 ], vjust = -4, hjust = 0.1) +
|
||||||
|
geom_text(aes(label = alphagroup, colour = as.factor(alpha), group = alphagroup, x = k-2.5, y = recall), size = 8, data = algorithm_recall_onlyalphas[k == 3 & alpha == 0.7 ], hjust = -0.1) +
|
||||||
|
geom_text(aes(label = alphagroup, colour = as.factor(alpha), group = alphagroup, x = k-2.5, y = recall), size = 8, data = algorithm_recall_onlyalphas[k == 1 & alpha == 0.8 ], vjust = 1, hjust = -0.1) +
|
||||||
|
geom_text(aes(label = alphagroup, colour = as.factor(alpha), group = alphagroup, x = k-2.5, y = recall), size = 8, data = algorithm_recall_onlyalphas[k == 2 & alpha == 0.9 ], hjust = 0.4) +
|
||||||
|
#geom_text_repel(aes(label = alphagroup, colour = as.factor(alpha), group = alphagroup, x = k-2.5, y = recall), size = 8, data = algorithm_recall_onlyalphas[k == 1], hjust = 0, min.segment.length = 10) +
|
||||||
|
scale_colour_brewer(type = "qual", palette = 2) +
|
||||||
|
labs(y = expression("Recall @ "~italic("k")), x = expression(italic("k"))) +
|
||||||
|
expand_limits(x = c(-2, 10)) +
|
||||||
|
theme_minimal() + theme(legend.position = "none", text = element_text(size = 18, family = "Open Sans"), panel.grid.major = element_blank(), panel.grid.minor = element_blank())
|
||||||
|
|
||||||
|
ggsave(recall_plot_alphas, file = "output/alphaicon_paper/recall_plot_alphas.pdf", width = 12, height = 9, device = cairo_pdf, scale = 0.6)
|
||||||
|
|
||||||
|
# For reporting: table with recall
|
||||||
|
algorithms_to_report <- c("baseline", "DPI", "NPI", "NPI SH", "α=0.0", "α=0.999", "α=0.999 SH", "α=0.999 SH DPI")
|
||||||
|
|
||||||
|
algorithm_recall[, recall := round(recall, 3) ]
|
||||||
|
algorithm_recall[ k == 1 & algorithm %in% algorithms_to_report, c("algorithm", "recall")]
|
||||||
|
algorithm_recall[ k == 3 & algorithm %in% algorithms_to_report, c("algorithm", "recall")]
|
||||||
|
algorithm_recall[ k == 5 & algorithm %in% algorithms_to_report, c("algorithm", "recall")]
|
||||||
|
algorithm_recall[ k == 10 & algorithm %in% algorithms_to_report, c("algorithm", "recall")]
|
||||||
|
|
||||||
|
|
||||||
|
########
|
||||||
|
# Produce the evaluation plots (by path length)
|
||||||
|
|
||||||
|
# One subset for display: only the best performing α-ICON versus other algorithms
|
||||||
|
algorithm_recall_by_pathlength_otheralgs <- algorithm_recall_by_pathlength[ algorithm %in% c("NPI", "α=0.999", "α=0.999 SH") ]
|
||||||
|
|
||||||
|
## Other algorithms vs best and worst-performing α-ICON algorithm
|
||||||
|
recall_path_plot <- ggplot(aes(x = path, y = recall, group = algorithm, color = algorithm), data = algorithm_recall_by_pathlength_otheralgs[ k == 1 ]) +
|
||||||
|
geom_line(size = 1.5, alpha = 1) +
|
||||||
|
geom_point(size = 2) +
|
||||||
|
scale_x_continuous(breaks = 1:12) +
|
||||||
|
scale_y_continuous(limits = c(0, 1)) +
|
||||||
|
labs(y = "Recall @ 1 for parents within path", x = expression(italic("l")~", path length from subsidiary to parent")) +
|
||||||
|
#scale_colour_brewer(type = "qual", palette = 3) +
|
||||||
|
scale_color_manual(name = "", values = c("#b2df8a", "#33a02c", "#1f78b4")) +
|
||||||
|
expand_limits(x = c(1, 12)) +
|
||||||
|
theme_minimal() + theme(legend.position = "bottom", text = element_text(size = 18, family = "Open Sans"), panel.grid.major = element_blank(), panel.grid.minor = element_blank())
|
||||||
|
|
||||||
|
ggsave(recall_path_plot, file = "output/alphaicon_paper/recall_path_plot_at_1.pdf", width = 12, height = 9, device = cairo_pdf, scale = 0.6)
|
||||||
|
|
||||||
|
# For reporting: table with recall
|
||||||
|
algorithm_recall_by_pathlength[, recall := round(recall, 3) ]
|
||||||
|
|
||||||
|
algorithms_path_length_to_report <- c("baseline", "DPI", "NPI", "NPI SH", "α=0.0", "α=0.999", "α=0.999 SH", "α=0.999 SH DPI")
|
||||||
|
|
||||||
|
algorithm_recall_by_pathlength[ path == 1 & algorithm %in% algorithms_path_length_to_report & k == 1, c("algorithm", "recall") ]
|
||||||
|
algorithm_recall_by_pathlength[ path == 2 & algorithm %in% algorithms_path_length_to_report & k == 1, c("algorithm", "recall") ]
|
||||||
|
algorithm_recall_by_pathlength[ path == 3 & algorithm %in% algorithms_path_length_to_report & k == 1, c("algorithm", "recall") ]
|
||||||
|
algorithm_recall_by_pathlength[ path == 4 & algorithm %in% algorithms_path_length_to_report & k == 1, c("algorithm", "recall") ]
|
||||||
|
algorithm_recall_by_pathlength[ path == 5 & algorithm %in% algorithms_path_length_to_report & k == 1, c("algorithm", "recall") ]
|
||||||
|
algorithm_recall_by_pathlength[ path == 10 & algorithm %in% algorithms_path_length_to_report & k == 1, c("algorithm", "recall") ]
|
||||||
|
algorithm_recall_by_pathlength[ path == 12 & algorithm %in% algorithms_path_length_to_report & k == 1, c("algorithm", "recall") ]
|
431
code/alphaicon_paper/6_rank_top_holders.r
Normal file
431
code/alphaicon_paper/6_rank_top_holders.r
Normal file
|
@ -0,0 +1,431 @@
|
||||||
|
# This code sums the computed control shares
|
||||||
|
# of the super-holders in the PSC data and
|
||||||
|
# produces the rankings under different algorithms
|
||||||
|
# for the α-ICON paper
|
||||||
|
library(data.table)
|
||||||
|
library(lubridate)
|
||||||
|
library(stringi)
|
||||||
|
library(stringr)
|
||||||
|
library(stargazer)
|
||||||
|
|
||||||
|
# Declare working directory beforehand in an environment variable
|
||||||
|
# ALPHAICON_PATH = "path_to_your_folder"
|
||||||
|
# with the aid of usethis::edit_r_environ()
|
||||||
|
# Restart R session for the changes to make effect
|
||||||
|
setwd(Sys.getenv('ALPHAICON_PATH'))
|
||||||
|
|
||||||
|
############
|
||||||
|
# Data loading
|
||||||
|
|
||||||
|
# Load the active participants snapshot of PSC (prepared by data_preparation/uk/2_psc_snapshot_to_participants_panel.r)
|
||||||
|
psc <- fread("output/uk/uk_organisations_participants_2021_long_2aug21.csv", integer64 = "character", na.strings = "", encoding = "UTF-8")
|
||||||
|
|
||||||
|
# Load the companies data (prepared by data_preparation/uk/1b_process_companies_data.r)
|
||||||
|
load("data/uk/uk_basic_companies_data_2021-08-01.rdata")
|
||||||
|
|
||||||
|
# Load the DPI of each participant after 10000 iterations (computed by alphaicon_paper/2_compute_npi_dpi.r)
|
||||||
|
holders_dpi <- fread("output/uk/npi_dpi/10000iter/uk_organisations_participants_2021_long_7sep21_dpi_10000iter.csv", integer64 = "character", na.strings = "", encoding = "UTF-8")
|
||||||
|
setnames(holders_dpi, c("entity", "participant", "dpi"), c("company_number", "participant_id", "share"))
|
||||||
|
holders_dpi[, times_pivotal := NULL ]
|
||||||
|
|
||||||
|
# Load the NPI of each participant after 10000 iterations (computed by alphaicon_paper/2_compute_npi_dpi.r)
|
||||||
|
holders_npi <- fread("output/uk/npi_dpi/10000iter/uk_organisations_participants_2021_long_7sep21_npi_10000iter.csv", integer64 = "character", na.strings = "", encoding = "UTF-8")
|
||||||
|
setnames(holders_npi, c("entity", "participant", "npi"), c("company_number", "participant_id", "share"))
|
||||||
|
holders_npi[, times_pivotal := NULL ]
|
||||||
|
|
||||||
|
# Load the transitive ownership data at various alpha
|
||||||
|
# (prepared by alphaicon_paper/1_compute_alphaicon.ipynb)
|
||||||
|
alphas <- c("0.0", "0.1", "0.2", "0.3", "0.4", "0.5", "0.6", "0.7", "0.8", "0.9", "0.999")
|
||||||
|
|
||||||
|
for( a in alphas) {
|
||||||
|
|
||||||
|
temp <- fread(paste0("output/uk/transitive/uk_organisations_transitive_ownership_alpha", a, "_2021_long_2aug21.csv"), integer64 = "character", na.strings = "", encoding = "UTF-8", colClasses = c("character", "character", "numeric"))
|
||||||
|
|
||||||
|
assign(paste0("holders_transitive_alpha", a), temp)
|
||||||
|
|
||||||
|
rm(temp)
|
||||||
|
|
||||||
|
}
|
||||||
|
|
||||||
|
# Load the data on graph membership
|
||||||
|
# (prepared by alphaicon_paper/1_compute_alphaicon.ipynb)
|
||||||
|
graph_membership <- fread("output/uk/uk_organisations_participation_graph_core_periphery_membership_6aug21.csv", integer64 = "character", na.strings = "", encoding = "UTF-8", header = T, colClasses = c("numeric", "character", "factor"))
|
||||||
|
graph_membership[, V1 := NULL ]
|
||||||
|
setnames(graph_membership, "company_number/id", "participant_id")
|
||||||
|
|
||||||
|
# All holder objects that are loaded in the memory
|
||||||
|
holder_objects <- paste0("holders_", c("dpi", "npi", paste0("transitive_alpha", alphas)))
|
||||||
|
|
||||||
|
# Add the graph membership and remove self-loops
|
||||||
|
for(obj in holder_objects) {
|
||||||
|
|
||||||
|
get(obj)[, participant_type := graph_membership[ match(get(obj)$participant_id, graph_membership$participant_id) ]$type ]
|
||||||
|
get(obj)[, company_type := graph_membership[ match(get(obj)$company_number, graph_membership$participant_id) ]$type ]
|
||||||
|
|
||||||
|
assign(obj, get(obj)[ company_number != participant_id ])
|
||||||
|
|
||||||
|
}
|
||||||
|
|
||||||
|
psc[, participant_type := graph_membership[ match(psc$participant_id, graph_membership$participant_id) ]$type ]
|
||||||
|
psc[, company_type := graph_membership[ match(psc$company_number, graph_membership$participant_id) ]$type ]
|
||||||
|
|
||||||
|
|
||||||
|
############
|
||||||
|
# Identify top holders
|
||||||
|
|
||||||
|
# Compute total holdings (weighted and unweighted by assets)
|
||||||
|
holdings_dpi <- holders_dpi[, list( sumdpi = sum(share)), by = "participant_id"]
|
||||||
|
holdings_dpi[, rankdpi := frankv(sumdpi, order = -1, ties.method = "first")]
|
||||||
|
|
||||||
|
holdings_npi <- holders_npi[, list( sumnpi = sum(share)), by = "participant_id"]
|
||||||
|
holdings_npi[, ranknpi := frankv(sumnpi, order = -1, ties.method = "first")]
|
||||||
|
|
||||||
|
# Normalize shares by type to one for our transitive data
|
||||||
|
holders_transitive_alpha0.999[, share_typenormalized := share/sum(share), by = c("company_number", "participant_type")]
|
||||||
|
|
||||||
|
# Keep only super-holders and sum up their shares
|
||||||
|
holdings_transitive <- holders_transitive_alpha0.999[participant_type == "SH", list( sumtransitive = sum(share_typenormalized)), by = "participant_id"]
|
||||||
|
|
||||||
|
# Rank the holders by their share
|
||||||
|
holdings_transitive[, ranktransitive := frankv(sumtransitive, order = -1, ties.method = "first")]
|
||||||
|
|
||||||
|
# Top-100 participants by our metric compared to other metrics
|
||||||
|
top_holders <- setorderv(holdings_transitive, "ranktransitive", 1)[1:100]
|
||||||
|
|
||||||
|
# Add ranks from other algorithms
|
||||||
|
top_holders <- merge(top_holders, holdings_dpi, by = "participant_id", all.x = T, all.y = F, sort = F)
|
||||||
|
top_holders <- merge(top_holders, holdings_npi, by = "participant_id", all.x = T, all.y = F, sort = F)
|
||||||
|
|
||||||
|
# Proper names
|
||||||
|
## First, match on company number with companies data
|
||||||
|
top_holders[, participant_name := uk_basic_companies_data[ match(top_holders$participant_id, uk_basic_companies_data$CompanyNumber)]$CompanyName ]
|
||||||
|
## Second, extract the company name
|
||||||
|
top_holders[ str_count(participant_id, fixed("$")) == 2, participant_name := stri_split_fixed(participant_id, "$", n = 3, simplify = T)[,1] ]
|
||||||
|
## Third, add the first and last names of the persons
|
||||||
|
top_holders[ str_count(participant_id, fixed("$")) == 4, participant_name := paste0(stri_split_fixed(participant_id, "$", n = 4, simplify = T)[, 1], " ", stri_split_fixed(participant_id, "$", n = 4, simplify = T)[, 3]) ]
|
||||||
|
|
||||||
|
## We no longer need participant id
|
||||||
|
#top_holders[, participant_id := NULL ]
|
||||||
|
|
||||||
|
# Proper column order
|
||||||
|
setcolorder(top_holders, c("participant_name", "participant_id", "ranktransitive", "ranknpi", "rankdpi", "sumtransitive", "sumnpi", "sumdpi"))
|
||||||
|
|
||||||
|
# Round the score
|
||||||
|
top_holders[, c("sumtransitive", "sumnpi", "sumdpi") := lapply(.SD, round, 1), .SDcols = c("sumtransitive", "sumnpi", "sumdpi") ]
|
||||||
|
|
||||||
|
# Add the size of the network of holdings and the core-shell distribution
|
||||||
|
top_holders_network_chars <- data.table()
|
||||||
|
|
||||||
|
for(superholder_id in top_holders$participant_id) {
|
||||||
|
|
||||||
|
# Debug: superholder_id <- "SC095000"
|
||||||
|
|
||||||
|
# Ultimate companies held by this organization
|
||||||
|
holders_subset_ultimate <- holders_transitive_alpha0.999[ participant_id %in% superholder_id ]
|
||||||
|
|
||||||
|
# Consider their total interest in all entities
|
||||||
|
holders_subset <- psc[ participant_id %in% unique(holders_subset_ultimate$company_number) | company_number %in% unique(holders_subset_ultimate$company_number) ]
|
||||||
|
holders_subset <- holders_subset[ !is.na(equity_share)]
|
||||||
|
holders_subset <- holders_subset[ participant_id != company_number]
|
||||||
|
|
||||||
|
# Share to 0-1 range
|
||||||
|
setnames(holders_subset, "equity_share", "share")
|
||||||
|
holders_subset[, share := share/100]
|
||||||
|
|
||||||
|
## Create an object with vertex metadata
|
||||||
|
vertex_metadata <- unique(rbind(unique(data.table( name = holders_subset$company_number, type = holders_subset$company_type)),
|
||||||
|
data.table( name = holders_subset$participant_id, type = holders_subset$participant_type)
|
||||||
|
), by = "name")
|
||||||
|
|
||||||
|
# Count all vertex types
|
||||||
|
out <- vertex_metadata[, .N, by = "type"]
|
||||||
|
# Transpose
|
||||||
|
out <- dcast(melt(out, id.vars = "type"), variable ~ type)
|
||||||
|
out[, variable := NULL ]
|
||||||
|
|
||||||
|
# Keep only the columns of interest in the order of interest
|
||||||
|
types_of_interest <- c("SH", "ST", "I", "C")
|
||||||
|
names_outside_types_of_interest <- types_of_interest[!(types_of_interest %in% names(out))]
|
||||||
|
if( length(names_outside_types_of_interest) > 0) {
|
||||||
|
out[, c(names_outside_types_of_interest) := 0]
|
||||||
|
}
|
||||||
|
out <- out[, types_of_interest, with = F]
|
||||||
|
|
||||||
|
# Add the network size
|
||||||
|
out <- data.table(participant_id = superholder_id, nodes = nrow(vertex_metadata), out)
|
||||||
|
|
||||||
|
top_holders_network_chars <- rbind(top_holders_network_chars, out, fill = T)
|
||||||
|
|
||||||
|
message(superholder_id)
|
||||||
|
|
||||||
|
}
|
||||||
|
|
||||||
|
# Add to the top holders data
|
||||||
|
top_holders <- merge(top_holders, top_holders_network_chars, by = "participant_id", sort = F)
|
||||||
|
|
||||||
|
# Write to CSV
|
||||||
|
fwrite(top_holders, file = "output/alphaicon_paper/uk_organisations_top100_holders_2021_long_2aug21.csv")
|
||||||
|
|
||||||
|
# Produce a TeX table
|
||||||
|
#stargazer(top_holders[1:25, -"participant_id"], type = "latex", summary = F, out = "output/alphaicon_paper/uk_organisations_top25_holders_2021_long_2aug21.tex", float = F, multicolumn = T, digits = 1)
|
||||||
|
|
||||||
|
# Export the information on top-25 entities with the largest deviation between ranks
|
||||||
|
combined_holdings <- merge(holdings_dpi, holdings_npi, by = "participant_id", all = T)
|
||||||
|
combined_holdings <- merge(combined_holdings, holdings_transitive, by = "participant_id", all = T)
|
||||||
|
|
||||||
|
combined_holdings[, diff_npi_dpi := sumnpi - sumdpi ]
|
||||||
|
combined_holdings[, diff_transitive_dpi := sumtransitive - sumdpi ]
|
||||||
|
combined_holdings[, diff_transitive_npi := sumtransitive - sumnpi ]
|
||||||
|
|
||||||
|
top100_holders_diff_npi_dpi <- setorderv(combined_holdings, "diff_npi_dpi", -1, na.last = T)[1:100]
|
||||||
|
top100_holders_diff_transitive_dpi <- setorderv(combined_holdings, "diff_transitive_dpi", -1, na.last = T)[1:100]
|
||||||
|
top100_holders_diff_transitive_npi <- setorderv(combined_holdings, "diff_transitive_npi", -1, na.last = T)[1:100]
|
||||||
|
|
||||||
|
# Export point
|
||||||
|
#fwrite(top100_holders_diff_npi_dpi, file = "output/alphaicon_paper/uk_organisations_top100_holders_diff_npi_dpi_2021_long_2aug21.csv")
|
||||||
|
#fwrite(top100_holders_diff_transitive_dpi, file = "output/alphaicon_paper/uk_organisations_top100_holders_diff_transitive_dpi_2021_long_2aug21.csv")
|
||||||
|
#fwrite(top100_holders_diff_transitive_npi, file = "output/alphaicon_paper/uk_organisations_top100_holders_diff_transitive_npi_2021_long_2aug21.csv")
|
||||||
|
|
||||||
|
############
|
||||||
|
# Agreement between rankings
|
||||||
|
|
||||||
|
# Add the ranks (takes time)
|
||||||
|
for(obj in holder_objects) {
|
||||||
|
|
||||||
|
# Create temporary variable prioritising super-holders
|
||||||
|
if( grepl("transitive", obj) ) {
|
||||||
|
|
||||||
|
get(obj)[, superholder := 0]
|
||||||
|
get(obj)[participant_type == "SH", superholder := 1]
|
||||||
|
|
||||||
|
}
|
||||||
|
# Sort by descending share within companies
|
||||||
|
if( grepl("transitive", obj) ) {
|
||||||
|
|
||||||
|
setorderv(get(obj), c("company_number", "superholder", "share"), c(1, -1, -1))
|
||||||
|
|
||||||
|
} else {
|
||||||
|
|
||||||
|
setorderv(get(obj), c("company_number", "share"), c(1, -1))
|
||||||
|
|
||||||
|
}
|
||||||
|
|
||||||
|
# Add the rank that prioritises super-holders
|
||||||
|
get(obj)[, rank := 1:.N, by = c("company_number")]
|
||||||
|
|
||||||
|
message(obj)
|
||||||
|
|
||||||
|
}
|
||||||
|
|
||||||
|
# Combine the ranks from all the methods
|
||||||
|
vars_to_keep <- c("company_number", "participant_id", "rank")
|
||||||
|
|
||||||
|
## DPI and NPI
|
||||||
|
holders_rankings <- merge(holders_dpi[, c(vars_to_keep), with = F], holders_npi[, c(vars_to_keep), with = F], by = c("company_number", "participant_id"), all = T)
|
||||||
|
setnames(holders_rankings, c("rank.x", "rank.y"), c("rank_dpi", "rank_npi"))
|
||||||
|
|
||||||
|
## Add transitive holder hankings (only super-holders or top-ranking non-super-holders)
|
||||||
|
for( a in alphas) {
|
||||||
|
|
||||||
|
holders_rankings <- merge(holders_rankings, get(paste0("holders_transitive_alpha", a))[ participant_type == "SH" | (participant_type != "SH" & rank == 1), c(vars_to_keep), with = F], by = c("company_number", "participant_id"), all = T)
|
||||||
|
setnames(holders_rankings, c("rank"), paste0("rank_transitive", a))
|
||||||
|
|
||||||
|
}
|
||||||
|
|
||||||
|
# Count holders by company from each method
|
||||||
|
holders_rankings[, countholders_dpi := sum(!is.na(rank_dpi)), by = "company_number"]
|
||||||
|
|
||||||
|
holders_rankings[, countholders_npi := sum(!is.na(rank_npi)), by = "company_number"]
|
||||||
|
|
||||||
|
for( a in alphas) {
|
||||||
|
|
||||||
|
holders_rankings[, paste0("countholders_transitive", a) := sum(!is.na(get(paste0("rank_transitive", a)))), by = "company_number"]
|
||||||
|
|
||||||
|
message(a)
|
||||||
|
|
||||||
|
}
|
||||||
|
|
||||||
|
# Pairs of counts of holders by company
|
||||||
|
holders_rankings[, countholders_dpi_npi := sum(!is.na(rank_dpi) & !is.na(rank_npi)), by = "company_number"]
|
||||||
|
|
||||||
|
for( a in alphas) {
|
||||||
|
|
||||||
|
holders_rankings[, paste0("countholders_dpi_transitive", a) := sum(!is.na(rank_dpi) & !is.na(get(paste0("rank_transitive", a)))), by = "company_number"]
|
||||||
|
|
||||||
|
holders_rankings[, paste0("countholders_npi_transitive", a) := sum(!is.na(rank_npi) & !is.na(get(paste0("rank_transitive", a)))), by = "company_number"]
|
||||||
|
|
||||||
|
message(a)
|
||||||
|
|
||||||
|
}
|
||||||
|
|
||||||
|
## Compute company-level Kendal's tau-b rank correlation coefficient
|
||||||
|
### (takes about 15 minutes per pair)
|
||||||
|
tau_dpi_npi <- holders_rankings[ countholders_dpi_npi > 1 & !is.na(rank_dpi) & !is.na(rank_npi), list(kendalltau_dpi_npi = cor(rank_dpi, rank_npi, method = "kendall", use = "pairwise.complete.obs")), by = "company_number"]
|
||||||
|
|
||||||
|
for( a in alphas) {
|
||||||
|
|
||||||
|
eval(parse(text = paste0("tau_dpi_transitive", a, " <- holders_rankings[ countholders_dpi_transitive", a, " > 1 & !is.na(rank_dpi) & !is.na(rank_transitive", a, "), list(kendalltau_dpi_transitive", a, " = cor(rank_dpi, rank_transitive", a, ", method = 'kendall', use = 'pairwise.complete.obs')), by = 'company_number']")))
|
||||||
|
|
||||||
|
eval(parse(text = paste0("tau_npi_transitive", a, " <- holders_rankings[ countholders_npi_transitive", a, " > 1 & !is.na(rank_npi) & !is.na(rank_transitive", a, "), list(kendalltau_npi_transitive", a, " = cor(rank_npi, rank_transitive", a, ", method = 'kendall', use = 'pairwise.complete.obs')), by = 'company_number']")))
|
||||||
|
|
||||||
|
message(a)
|
||||||
|
|
||||||
|
}
|
||||||
|
|
||||||
|
# Examples of companies with complex rankings
|
||||||
|
#holders_rankings[ company_number == "00026306"]
|
||||||
|
#holders_rankings[ company_number == "00000529"]
|
||||||
|
#holders_rankings[ company_number == "00018751"]
|
||||||
|
|
||||||
|
# Debug: these should be zeros
|
||||||
|
#holders_rankings[ countholders_dpi == 1 & countholders_npi == 1 & rank_dpi != rank_npi]
|
||||||
|
#holders_rankings[ countholders_transitive0.999 == 1 & countholders_npi == 1 & rank_transitive0.999 != rank_npi]
|
||||||
|
|
||||||
|
# Add the tau-b = 1 for companies with one participant (both direct and indirect)
|
||||||
|
tau_dpi_npi <- rbind(tau_dpi_npi, data.table(company_number = unique(holders_rankings[ countholders_dpi == 1 & countholders_npi == 1 & !is.na(rank_dpi) & !is.na(rank_npi) ]$company_number), kendalltau_dpi_npi = 1))
|
||||||
|
|
||||||
|
for( a in alphas) {
|
||||||
|
|
||||||
|
eval(parse(text = paste0("tau_dpi_transitive", a, " <- rbind(tau_dpi_transitive", a, ", data.table( company_number = unique(holders_rankings[ countholders_dpi == 1 & countholders_transitive", a, " == 1 & !is.na(rank_dpi) & !is.na(rank_transitive", a, ")]$company_number), kendalltau_dpi_transitive", a, " = 1))")))
|
||||||
|
|
||||||
|
eval(parse(text = paste0("tau_npi_transitive", a, " <- rbind(tau_npi_transitive", a, ", data.table( company_number = unique(holders_rankings[ countholders_npi == 1 & countholders_transitive", a, " == 1 & !is.na(rank_npi) & !is.na(rank_transitive", a, ")]$company_number), kendalltau_npi_transitive", a, " = 1))")))
|
||||||
|
|
||||||
|
}
|
||||||
|
|
||||||
|
# Add the tau-b = -1 for companies with differing number of participants
|
||||||
|
# where ranks do not match. Example: holders_rankings[ company_number == "00026306"]
|
||||||
|
tau_dpi_npi <- rbind(tau_dpi_npi, data.table(company_number = unique(holders_rankings[ !(company_number %in% tau_dpi_npi$company_number)]$company_number), kendalltau_dpi_npi = -1))
|
||||||
|
|
||||||
|
for( a in alphas) {
|
||||||
|
|
||||||
|
eval(parse(text = paste0("tau_dpi_transitive", a, " <- rbind(tau_dpi_transitive", a, ", data.table( company_number = unique(holders_rankings[ !(company_number %in% tau_dpi_transitive", a, "$company_number) ]$company_number), kendalltau_dpi_transitive", a, " = -1))")))
|
||||||
|
|
||||||
|
eval(parse(text = paste0("tau_npi_transitive", a, " <- rbind(tau_npi_transitive", a, ", data.table( company_number = unique(holders_rankings[ !(company_number %in% tau_npi_transitive", a, "$company_number) ]$company_number), kendalltau_npi_transitive", a, " = -1))")))
|
||||||
|
|
||||||
|
|
||||||
|
}
|
||||||
|
|
||||||
|
# Finally, we need to fill in NAs like tau_dpi_transitive0.999[company_number == "00001615"]
|
||||||
|
# holders_rankings[ company_number == "00001615" ]
|
||||||
|
# holders_rankings[ company_number == "SO307326" ]
|
||||||
|
# holders_rankings[ company_number == "00000529" ]
|
||||||
|
|
||||||
|
# Merge the taus
|
||||||
|
for( a in alphas) {
|
||||||
|
|
||||||
|
if( which(alphas == a) == 1 ) {
|
||||||
|
|
||||||
|
kendall_taus_participant_ranks <- merge(tau_dpi_npi, get(paste0("tau_dpi_transitive", a)), by = "company_number", all = T)
|
||||||
|
kendall_taus_participant_ranks <- merge(kendall_taus_participant_ranks, get(paste0("tau_npi_transitive", a)), by = "company_number", all = T)
|
||||||
|
|
||||||
|
|
||||||
|
} else {
|
||||||
|
|
||||||
|
kendall_taus_participant_ranks <- merge(kendall_taus_participant_ranks, get(paste0("tau_dpi_transitive", a)), by = "company_number", all = T)
|
||||||
|
kendall_taus_participant_ranks <- merge(kendall_taus_participant_ranks, get(paste0("tau_npi_transitive", a)), by = "company_number", all = T)
|
||||||
|
|
||||||
|
}
|
||||||
|
|
||||||
|
message(a)
|
||||||
|
}
|
||||||
|
|
||||||
|
# Add the information on the count of holders
|
||||||
|
kendall_taus_participant_ranks <- merge(kendall_taus_participant_ranks, unique(holders_rankings[, c("company_number", "countholders_dpi", "countholders_npi", paste0("countholders_transitive", alphas)), with = F], by = "company_number"), by = "company_number", all.x = T, all.y = F)
|
||||||
|
|
||||||
|
# Save point
|
||||||
|
fwrite(kendall_taus_participant_ranks, file = "output/alphaicon_paper/kendall_taus_participant_ranks_dpi_npi_transitive_uk_organisations_participants_2021_7sep21.csv")
|
||||||
|
|
||||||
|
############
|
||||||
|
# Analyse the agreement between rankings
|
||||||
|
library(data.table)
|
||||||
|
library(ggplot2)
|
||||||
|
library(ggthemes)
|
||||||
|
library(ggrepel)
|
||||||
|
library(stringi)
|
||||||
|
library(stringr)
|
||||||
|
library(stargazer)
|
||||||
|
library(showtext)
|
||||||
|
|
||||||
|
setwd(Sys.getenv('ALPHAICON_PATH'))
|
||||||
|
|
||||||
|
# Add the font to use
|
||||||
|
font_add_google("Open Sans", "Open Sans")
|
||||||
|
showtext_auto()
|
||||||
|
|
||||||
|
# Load the produced CSV with Kendall's tau-b
|
||||||
|
taus_longs <- fread("output/alphaicon_paper/kendall_taus_participant_ranks_dpi_npi_transitive_uk_organisations_participants_2021_7sep21.csv", encoding = "UTF-8")
|
||||||
|
|
||||||
|
# To long form (each line is method and alpha)
|
||||||
|
taus <- melt(taus_longs, id.vars = "company_number", measure.vars = names(taus_longs)[grepl("kendalltau", names(taus_longs))], variable.name = "pair", value.name = "tau", na.rm = T, variable.factor = T)
|
||||||
|
|
||||||
|
countholders <- melt(taus_longs, id.vars = "company_number", measure.vars = names(taus_longs)[grepl("countholders", names(taus_longs))], variable.name = "algorithm", value.name = "countholders", na.rm = T, variable.factor = T)
|
||||||
|
|
||||||
|
# Convert pairs to extract useful information
|
||||||
|
alg_pairs <- data.table(pair = levels(taus$pair))
|
||||||
|
alg_pairs[, pair_modif := gsub("kendalltau_", "", pair, fixed = T)]
|
||||||
|
alg_pairs[, c("algorithm1", "algorithm2") := as.data.table(stri_split_fixed(pair_modif, "_", n = 2, simplify = T))]
|
||||||
|
alg_pairs[, pair_modif := NULL]
|
||||||
|
alg_pairs[, pair := factor(pair, levels = levels(taus$pair))]
|
||||||
|
alg_pairs[, alpha := NA_real_ ]
|
||||||
|
alg_pairs[ grepl("transitive", algorithm2), alpha := as.numeric(gsub("transitive", "", algorithm2))]
|
||||||
|
alg_pairs[ grepl("transitive", algorithm2), algorithm2 := gsub("[^transitive]", "", algorithm2)]
|
||||||
|
alg_pairs[, algorithm1 := factor(algorithm1, levels = c("dpi", "npi", "transitive"))]
|
||||||
|
alg_pairs[, algorithm2 := factor(algorithm2, levels = c("dpi", "npi", "transitive"))]
|
||||||
|
|
||||||
|
taus <- merge(taus, alg_pairs, by = "pair")
|
||||||
|
taus[, pair := NULL]
|
||||||
|
gc()
|
||||||
|
|
||||||
|
# Same for countholders
|
||||||
|
alg_counts <- data.table(algorithm = levels(countholders$algorithm))
|
||||||
|
alg_counts[, alg_modif := gsub("countholders_", "", algorithm)]
|
||||||
|
alg_counts[ grepl("transitive", alg_modif), alpha := as.numeric(gsub("transitive", "", alg_modif))]
|
||||||
|
alg_counts[ grepl("transitive", alg_modif), alg_modif := gsub("[^transitive]", "", alg_modif)]
|
||||||
|
alg_counts[, alg_modif := factor(alg_modif, levels = c("dpi", "npi", "transitive"))]
|
||||||
|
|
||||||
|
countholders <- merge(countholders, alg_counts, by = "algorithm")
|
||||||
|
countholders[, algorithm := NULL]
|
||||||
|
setnames(countholders, "alg_modif", "algorithm")
|
||||||
|
gc()
|
||||||
|
|
||||||
|
# Add count holders to tau
|
||||||
|
#taus <- merge(taus, countholders, by.x = c("algorithm1", "alpha"), by.y = c("algorithm", "alpha"))
|
||||||
|
#setnames(taus, "countholders", "countholders1")
|
||||||
|
|
||||||
|
#taus <- merge(taus, countholders, by.x = c("algorithm2", "alpha"), by.y = c("algorithm", "alpha"))
|
||||||
|
#setnames(taus, "countholders", "countholders2")
|
||||||
|
|
||||||
|
# Add entity industry to the data (peak use of 40 GB)
|
||||||
|
taus[, industrysection := uk_basic_companies_data[ match(taus$company_number, uk_basic_companies_data$CompanyNumber)]$industrysection_1 ]
|
||||||
|
gc()
|
||||||
|
|
||||||
|
# Summary statistics of Kendall's tau-b
|
||||||
|
|
||||||
|
## Mean, overall
|
||||||
|
taus_overall_mean <- taus[!is.na(tau), list(tau = mean(tau), tausemean = sd(tau)/sqrt(.N)), by = c("algorithm1", "algorithm2", "alpha")]
|
||||||
|
|
||||||
|
## Mean, by industry
|
||||||
|
taus_industry_mean <- taus[!is.na(tau) & !is.na(industrysection), list( tau = mean(tau), tausemean = sd(tau)/sqrt(.N)), by = c("algorithm1", "algorithm2", "alpha", "industrysection")]
|
||||||
|
|
||||||
|
# Export point
|
||||||
|
#fwrite(taus_overall_mean, file = "output/alphaicon_paper/mean_kendall_taus_participant_ranks_dpi_npi_transitive_uk_organisations_participants_2021_7sep21.csv")
|
||||||
|
#fwrite(taus_industry_mean, file = "output/alphaicon_paper/mean_by_industrysection_kendall_taus_participant_ranks_dpi_npi_transitive_uk_organisations_participants_2021_7sep21.csv")
|
||||||
|
|
||||||
|
# Produce the plot with overall mean
|
||||||
|
taus_overall_mean[ grepl("dpi|npi", algorithm1), algorithm1 := toupper(algorithm1)]
|
||||||
|
|
||||||
|
## Other algorithms vs best and worst-performing transitive algorithm
|
||||||
|
taus_mean_plot <- ggplot(aes(x = alpha, y = tau, group = algorithm1, color = algorithm1), data = taus_overall_mean[ algorithm1 == "NPI" & algorithm2 == "transitive"]) +
|
||||||
|
geom_line(size = 1.5, alpha = 1) +
|
||||||
|
geom_point(size = 2) +
|
||||||
|
geom_text(aes(label = round(tau, 4)), size = 2.5, nudge_y = 0.0005) +
|
||||||
|
scale_x_continuous( breaks = c(seq(0, 0.9, 0.1), 0.999), labels = c(seq(0, 0.9, 0.1), 0.999), guide = guide_axis(n.dodge = 1)) +
|
||||||
|
#facet_wrap(. ~ algorithm1, scales = "free_y")+
|
||||||
|
labs(y = expression("Kendall's "~tau~"-b betw. NPI\nand "~alpha~"-ICON SH ranks"), x = expression(alpha)) +
|
||||||
|
scale_colour_brewer(type = "qual", palette = 2) +
|
||||||
|
theme_minimal() + theme(legend.position = "none", text = element_text(size = 14, family = "Open Sans"), panel.grid.major = element_blank(), panel.grid.minor = element_blank())
|
||||||
|
|
||||||
|
#ggsave(taus_mean_plot, file = "output/alphaicon_paper/taus_mean_plot.pdf", width = 8.5, height = 4, device = cairo_pdf, scale = 1)
|
||||||
|
|
||||||
|
# Export to a table
|
||||||
|
#stargazer(taus_overall_mean[ algorithm1 == "NPI" & algorithm2 == "transitive"], type = "latex", summary = F, out = "output/alphaicon_paper/taus_overall_mean.tex", float = F, multicolumn = T, digits = 5)
|
52
code/data_preparation/uk/1a_process_psc_snapshot.r
Normal file
52
code/data_preparation/uk/1a_process_psc_snapshot.r
Normal file
|
@ -0,0 +1,52 @@
|
||||||
|
# This code reads in the JSONL with daily
|
||||||
|
# Persons with Significant Control snapshot
|
||||||
|
# and saves the data on control in long format
|
||||||
|
# to a cleaned .rdata object
|
||||||
|
library(data.table)
|
||||||
|
library(ndjson)
|
||||||
|
library(lubridate)
|
||||||
|
library(stringi)
|
||||||
|
library(stringr)
|
||||||
|
|
||||||
|
# Declare working directory beforehand in an environment variable
|
||||||
|
# ALPHAICON_PATH = "path_to_your_folder"
|
||||||
|
# with the aid of usethis::edit_r_environ()
|
||||||
|
# Restart R session for the changes to make effect
|
||||||
|
setwd(Sys.getenv('ALPHAICON_PATH'))
|
||||||
|
|
||||||
|
# Stream in the JSONL with daily Persons with Significant Control
|
||||||
|
# information from http://download.companieshouse.gov.uk/en_pscdata.html
|
||||||
|
# (takes about 2 hours to load)
|
||||||
|
psc_snapshot <- ndjson::stream_in("data/uk/persons-with-significant-control-snapshot-2021-08-02.txt", cls = "dt")
|
||||||
|
|
||||||
|
# Unify the names
|
||||||
|
names(psc_snapshot) <- gsub("^data\\.", "", names(psc_snapshot), perl = T)
|
||||||
|
|
||||||
|
# Coerce variables to relevant types
|
||||||
|
## To dates
|
||||||
|
to_ymd_dates <- unique(c("ceased_on", "notified_on", names(psc_snapshot)[grepl("exempt_from|exempt_to", names(psc_snapshot))]))
|
||||||
|
|
||||||
|
psc_snapshot[, c(to_ymd_dates) := lapply(.SD, ymd), .SDcols = to_ymd_dates ]
|
||||||
|
|
||||||
|
# Remove last row with overall statistics
|
||||||
|
psc_snapshot[, c("exemptions_count", "generated_at", "persons_of_significant_control_count", "statements_count") := NULL ]
|
||||||
|
psc_snapshot <- psc_snapshot[!is.na(company_number)]
|
||||||
|
|
||||||
|
# Remove exemptions
|
||||||
|
psc_snapshot <- psc_snapshot[ kind != "exemptions"]
|
||||||
|
psc_snapshot[, names(psc_snapshot)[grepl("exempt", names(psc_snapshot))] := NULL ]
|
||||||
|
|
||||||
|
# Remove statements
|
||||||
|
psc_snapshot <- psc_snapshot[ kind != "persons-with-significant-control-statement" ]
|
||||||
|
psc_snapshot[, statement := NULL ]
|
||||||
|
|
||||||
|
# Remove variables with all NAs
|
||||||
|
na_count <- sapply(psc_snapshot, function(y) sum(length(which(is.na(y)))))
|
||||||
|
empty_vars <- names(na_count[ which( nrow(psc_snapshot) - na_count == 0) ])
|
||||||
|
psc_snapshot[, c(empty_vars) := NULL ]
|
||||||
|
|
||||||
|
# Kind to factor
|
||||||
|
psc_snapshot[, kind := as.factor(kind)]
|
||||||
|
|
||||||
|
# Save point
|
||||||
|
save(psc_snapshot, file = "data/uk/psc_snapshot_2021-08-02.rdata", compress = "gzip")
|
107
code/data_preparation/uk/1b_process_companies_data.r
Normal file
107
code/data_preparation/uk/1b_process_companies_data.r
Normal file
|
@ -0,0 +1,107 @@
|
||||||
|
# This code reads in the CSV with data
|
||||||
|
# on live UK companies and saves the data
|
||||||
|
# to a cleaned .rdata object
|
||||||
|
library(data.table)
|
||||||
|
library(lubridate)
|
||||||
|
library(stringi)
|
||||||
|
library(stringr)
|
||||||
|
|
||||||
|
# Declare working directory beforehand in an environment variable
|
||||||
|
# ALPHAICON_PATH = "path_to_your_folder"
|
||||||
|
# with the aid of usethis::edit_r_environ()
|
||||||
|
# Restart R session for the changes to make effect
|
||||||
|
setwd(Sys.getenv('ALPHAICON_PATH'))
|
||||||
|
|
||||||
|
# Read in the CSV with Free Company Data Product snapshot
|
||||||
|
# from http://download.companieshouse.gov.uk/en_output.html
|
||||||
|
uk_basic_companies_data <- fread("data/uk/BasicCompanyDataAsOneFile-2021-08-01.csv", encoding = "UTF-8", na.strings = "")
|
||||||
|
|
||||||
|
# Remove certain columns
|
||||||
|
cols_to_remove <- names(uk_basic_companies_data)[ grepl("PreviousName|Mortgages|ConfStmt", names(uk_basic_companies_data))]
|
||||||
|
uk_basic_companies_data[, c(cols_to_remove) := NULL ]
|
||||||
|
|
||||||
|
# Empty to NA
|
||||||
|
for(j in seq_along(uk_basic_companies_data)) {
|
||||||
|
set(uk_basic_companies_data, i = which(uk_basic_companies_data[[j]] == ""), j=j, value = NA)
|
||||||
|
}
|
||||||
|
|
||||||
|
# Convert dates
|
||||||
|
cols_to_date <- names(uk_basic_companies_data)[ grepl("Date", names(uk_basic_companies_data))]
|
||||||
|
|
||||||
|
uk_basic_companies_data[, c(cols_to_date) := lapply(.SD, dmy), .SDcols = cols_to_date ]
|
||||||
|
|
||||||
|
# Vars to factor
|
||||||
|
cols_to_factor <- c("RegAddress.Country", "CompanyCategory", "CompanyStatus", "CountryOfOrigin", "Accounts.AccountCategory")
|
||||||
|
|
||||||
|
uk_basic_companies_data[, c(cols_to_factor) := lapply(.SD, as.factor), .SDcols = cols_to_factor ]
|
||||||
|
|
||||||
|
# Split into industry code and industry name
|
||||||
|
uk_basic_companies_data[, SICCode.SicText_1 := as.character(SICCode.SicText_1) ]
|
||||||
|
uk_basic_companies_data[, SICCode.SicText_2 := as.character(SICCode.SicText_2) ]
|
||||||
|
uk_basic_companies_data[, SICCode.SicText_3 := as.character(SICCode.SicText_3) ]
|
||||||
|
uk_basic_companies_data[, SICCode.SicText_4 := as.character(SICCode.SicText_4) ]
|
||||||
|
|
||||||
|
uk_basic_companies_data[, c("industrycode_1", "industryname_1") := as.data.table(stri_split_fixed(SICCode.SicText_1, " - ", n = 2, simplify = T))]
|
||||||
|
uk_basic_companies_data[, c("industrycode_2", "industryname_2") := as.data.table(stri_split_fixed(SICCode.SicText_2, " - ", n = 2, simplify = T))]
|
||||||
|
uk_basic_companies_data[, c("industrycode_3", "industryname_3") := as.data.table(stri_split_fixed(SICCode.SicText_3, " - ", n = 2, simplify = T))]
|
||||||
|
uk_basic_companies_data[, c("industrycode_4", "industryname_4") := as.data.table(stri_split_fixed(SICCode.SicText_4, " - ", n = 2, simplify = T))]
|
||||||
|
|
||||||
|
# Fix empty entries
|
||||||
|
uk_basic_companies_data[ nchar(industryname_1) < 2, industrycode_1 := NA_character_]
|
||||||
|
uk_basic_companies_data[ nchar(industryname_1) < 2, industryname_1 := NA_character_]
|
||||||
|
|
||||||
|
uk_basic_companies_data[ nchar(industryname_2) < 2, industrycode_2 := NA_character_]
|
||||||
|
uk_basic_companies_data[ nchar(industryname_2) < 2, industryname_2 := NA_character_]
|
||||||
|
|
||||||
|
uk_basic_companies_data[ nchar(industryname_3) < 2, industrycode_3 := NA_character_]
|
||||||
|
uk_basic_companies_data[ nchar(industryname_3) < 2, industryname_3 := NA_character_]
|
||||||
|
|
||||||
|
uk_basic_companies_data[ nchar(industryname_4) < 2, industrycode_4 := NA_character_]
|
||||||
|
uk_basic_companies_data[ nchar(industryname_4) < 2, industryname_4 := NA_character_]
|
||||||
|
|
||||||
|
uk_basic_companies_data[, c("SICCode.SicText_1", "SICCode.SicText_2", "SICCode.SicText_3", "SICCode.SicText_4") := NULL ]
|
||||||
|
|
||||||
|
# Load SIC 2007 numeric coding of industries
|
||||||
|
sic_codes <- fread("data/uk/sic_2007_code_list.csv", encoding = "UTF-8", na.strings = "", colClasses = "character")
|
||||||
|
|
||||||
|
# Add leading zeros to industry codes
|
||||||
|
sic_codes[ nchar(sic_code) == 4, sic_code := paste0("0", sic_code) ]
|
||||||
|
|
||||||
|
# Debug
|
||||||
|
#uk_basic_companies_data[ industryname_1 %in% sic_codes[ substr(sic_code, 1, 1) == "0" ]$sic_description & substr(industrycode_1, 1, 1) != "0" ]
|
||||||
|
#uk_basic_companies_data[ industryname_2 %in% sic_codes[ substr(sic_code, 1, 1) == "0" ]$sic_description & substr(industrycode_2, 1, 1) != "0" ]
|
||||||
|
|
||||||
|
# Add industry section
|
||||||
|
uk_basic_companies_data[, industrysection_1 := sic_codes[ match(substr(uk_basic_companies_data$industrycode_1, 1, 2), substr(sic_codes$sic_code, 1, 2)) ]$section_description ]
|
||||||
|
uk_basic_companies_data[ industrycode_1 == "99999", industrysection_1 := NA ]
|
||||||
|
|
||||||
|
uk_basic_companies_data[, industrysection_2 := sic_codes[ match(substr(uk_basic_companies_data$industrycode_2, 1, 2), substr(sic_codes$sic_code, 1, 2)) ]$section_description ]
|
||||||
|
uk_basic_companies_data[ industrycode_2 == "99999", industrysection_2 := NA ]
|
||||||
|
|
||||||
|
uk_basic_companies_data[, industrysection_3 := sic_codes[ match(substr(uk_basic_companies_data$industrycode_3, 1, 2), substr(sic_codes$sic_code, 1, 2)) ]$section_description ]
|
||||||
|
uk_basic_companies_data[ industrycode_3 == "99999", industrysection_3 := NA ]
|
||||||
|
|
||||||
|
uk_basic_companies_data[, industrysection_4 := sic_codes[ match(substr(uk_basic_companies_data$industrycode_4, 1, 2), substr(sic_codes$sic_code, 1, 2)) ]$section_description ]
|
||||||
|
uk_basic_companies_data[ industrycode_4 == "99999", industrysection_4 := NA ]
|
||||||
|
|
||||||
|
# Factor coercion for industry codes
|
||||||
|
industry_names <- unique(c(uk_basic_companies_data$industryname_1, uk_basic_companies_data$industryname_2, uk_basic_companies_data$industryname_3, uk_basic_companies_data$industryname_4))
|
||||||
|
industry_names <- industry_names[!is.na(industry_names)]
|
||||||
|
industry_names <- industry_names[order(industry_names)]
|
||||||
|
|
||||||
|
industry_sections <- unique(c(uk_basic_companies_data$industrysection_1, uk_basic_companies_data$industrysection_2, uk_basic_companies_data$industrysection_3, uk_basic_companies_data$industrysection_4))
|
||||||
|
industry_sections <- industry_sections[!is.na(industry_sections)]
|
||||||
|
industry_sections <- industry_sections[order(industry_sections)]
|
||||||
|
|
||||||
|
uk_basic_companies_data[, industryname_1 := factor(industryname_1, levels = industry_names) ]
|
||||||
|
uk_basic_companies_data[, industryname_2 := factor(industryname_2, levels = industry_names) ]
|
||||||
|
uk_basic_companies_data[, industryname_3 := factor(industryname_3, levels = industry_names) ]
|
||||||
|
uk_basic_companies_data[, industryname_4 := factor(industryname_4, levels = industry_names) ]
|
||||||
|
|
||||||
|
uk_basic_companies_data[, industrysection_1 := factor(industrysection_1, levels = industry_sections) ]
|
||||||
|
uk_basic_companies_data[, industrysection_2 := factor(industrysection_2, levels = industry_sections) ]
|
||||||
|
uk_basic_companies_data[, industrysection_3 := factor(industrysection_3, levels = industry_sections) ]
|
||||||
|
uk_basic_companies_data[, industrysection_4 := factor(industrysection_4, levels = industry_sections) ]
|
||||||
|
|
||||||
|
# Save point
|
||||||
|
save(uk_basic_companies_data, file = "data/uk/uk_basic_companies_data_2021-08-01.rdata", compress = "gzip")
|
|
@ -0,0 +1,89 @@
|
||||||
|
# This code converts PSC data into
|
||||||
|
# a long CSV with entity-participant
|
||||||
|
# mapping that we will in the study
|
||||||
|
library(data.table)
|
||||||
|
library(lubridate)
|
||||||
|
library(stringi)
|
||||||
|
library(stringr)
|
||||||
|
|
||||||
|
# Declare working directory beforehand in an environment variable
|
||||||
|
# ALPHAICON_PATH = "path_to_your_folder"
|
||||||
|
# with the aid of usethis::edit_r_environ()
|
||||||
|
# Restart R session for the changes to make effect
|
||||||
|
setwd(Sys.getenv('ALPHAICON_PATH'))
|
||||||
|
|
||||||
|
# Load the PSC snapshot (prepared by 1a_process_psc_snapshot.r)
|
||||||
|
load("data/uk/psc_snapshot_2021-08-02.rdata")
|
||||||
|
|
||||||
|
# Load the companies data as well (prepared by 1b_process_companies_data.r)
|
||||||
|
load("data/uk/uk_basic_companies_data_2021-08-01.rdata")
|
||||||
|
|
||||||
|
# Keep only active companies and exclude data on secret participants
|
||||||
|
participants <- psc_snapshot[ is.na(ceased) & is.na(ceased_on) & company_number %in% uk_basic_companies_data[ CompanyStatus == "Active"]$CompanyNumber & kind != "super-secure-person-with-significant-control", c("company_number", "date_of_birth.month", "date_of_birth.year", "kind", "name", "name_elements.forename", "name_elements.middle_name", "name_elements.surname", "identification.country_registered", "identification.registration_number", names(psc_snapshot)[grepl("natures_of_control", names(psc_snapshot))] ), with = F ]
|
||||||
|
|
||||||
|
# Create participant name
|
||||||
|
## For individuals it is a concatenation of name and DOB
|
||||||
|
participants[kind == "individual-person-with-significant-control", participant_name := paste(toupper(name_elements.forename), toupper(name_elements.middle_name), toupper(name_elements.surname), date_of_birth.year, date_of_birth.month, sep = "$") ]
|
||||||
|
## For entities it is a concatenation of name, ID and country of incorporation
|
||||||
|
participants[kind != "individual-person-with-significant-control", participant_name := paste(toupper(name), toupper(identification.country_registered), toupper(identification.registration_number), sep = "$") ]
|
||||||
|
## Add NAs
|
||||||
|
participants[is.na(name) & is.na(name_elements.forename) & is.na(name_elements.middle_name) & is.na(name_elements.surname), participant_name := NA ]
|
||||||
|
|
||||||
|
# Participant ID is either domestic company number or individual name
|
||||||
|
## Consider company number as identifier
|
||||||
|
participants[ grepl("England|scotland|wales|^uk$|uk\\s+|United Kingdom|Great Britain|london|Cardiff|Engalnd|E\\&W|britain|Gb-Sct|N\\.Ireland|N\\. Ireland|Englan|Englad|Engand|Companies House|United Kindom|U\\.K|Gb-Nir|Unitied Kingdom|United Kingom|Endland|Great Britian|United Kigdom|Northern Ireland|Belfast|Englang|Gb-Wls|Kent|Enland|Sotland|Rngland|Englane|Scotlan|Englanf|Enagland|Staffordshire|Northen Ireland|Enagland|Englang|Englamd|Northamptonshire|Engkand|Emgland|England|United Kingtom|English|Devon|Durham|Stirlingshire|Register Of Companies|United Kingdo|Unitedd Kingdom|United Kingd|Scotlnd|Cumbria|Renfrewshire|Uunited Kingdom|Noerthern Ireland|Shropshire|Englabnd|Uk Company|United Kingdown|United King|U\\. K\\.|Ukl", identification.country_registered, perl = T, ignore.case = T) & identification.registration_number %in% unique(c(uk_basic_companies_data$CompanyNumber, psc_snapshot$company_number)), participant_id := identification.registration_number ]
|
||||||
|
|
||||||
|
## Create unique company name-company number mapping
|
||||||
|
## to restore company numbers
|
||||||
|
uk_company_name_number_mapping <- uk_basic_companies_data[, list(count_samename = .N), by = c("CompanyName") ]
|
||||||
|
uk_company_name_number_mapping <- uk_company_name_number_mapping[ count_samename == 1 ]
|
||||||
|
uk_company_name_number_mapping[, company_number := uk_basic_companies_data[ match(uk_company_name_number_mapping$CompanyName, uk_basic_companies_data$CompanyName)]$CompanyNumber ]
|
||||||
|
uk_company_name_number_mapping[, count_samename := NULL ]
|
||||||
|
|
||||||
|
## Add this to participant IDs
|
||||||
|
participants[ kind == "corporate-entity-person-with-significant-control", participant_id_namebased := uk_company_name_number_mapping[ match(toupper(participants[kind == "corporate-entity-person-with-significant-control"]$name), toupper(uk_company_name_number_mapping$CompanyName))]$company_number]
|
||||||
|
|
||||||
|
## Perform the replacement (about 95 thousand companies more now have proper IDs)
|
||||||
|
participants[ kind == "corporate-entity-person-with-significant-control" & is.na(participant_id) & !is.na(participant_id_namebased), participant_id := participant_id_namebased ]
|
||||||
|
participants[, participant_id_namebased := NULL ]
|
||||||
|
|
||||||
|
## If match on company ID fails, use the name-dob combination
|
||||||
|
participants[is.na(participant_id), participant_id := participant_name ]
|
||||||
|
|
||||||
|
# Remove irrelevant fields (except for "kind" as it is crucial in determining the participant_id)
|
||||||
|
participants[, c("date_of_birth.month", "date_of_birth.year", "name", "name_elements.forename", "name_elements.middle_name", "name_elements.surname", "identification.country_registered", "identification.registration_number", "participant_name") := NULL ]
|
||||||
|
|
||||||
|
# Remove possible duplicates
|
||||||
|
nrow(participants) # 5502058
|
||||||
|
participants <- unique(participants, by = c("company_number", "participant_id"))
|
||||||
|
nrow(participants) # 5482200
|
||||||
|
|
||||||
|
# To long data to obtain equity shares
|
||||||
|
participants_long <- melt(participants, id.vars = c("company_number", "participant_id"), value.name = "nature_of_control", na.rm = T)
|
||||||
|
participants_long[, variable := NULL ]
|
||||||
|
|
||||||
|
participants_long[, equity_share := NA_real_]
|
||||||
|
participants_long[grepl("25-to-50", nature_of_control, perl = T), equity_share := 37.5]
|
||||||
|
participants_long[grepl("50-to-75", nature_of_control, perl = T), equity_share := 62.5]
|
||||||
|
participants_long[grepl("75-to-100", nature_of_control, perl = T), equity_share := 87.5]
|
||||||
|
|
||||||
|
# Back to participants data, but now with equity share
|
||||||
|
temp <- participants_long[, list(equity_share = max(equity_share, na.rm = T)), by = c("company_number", "participant_id")]
|
||||||
|
temp[!is.finite(equity_share), equity_share := NA]
|
||||||
|
participants <- merge(participants[, c("company_number", "participant_id", "kind"), with = F], temp, by = c("company_number", "participant_id"), all = F, sort = F)
|
||||||
|
|
||||||
|
# Replace NAs with proper value of full ownership if we have one person per entity
|
||||||
|
participants[, persons_per_entity := .N, by = "company_number" ]
|
||||||
|
participants[persons_per_entity == 1 & is.na(equity_share), equity_share := 87.5 ]
|
||||||
|
participants[, persons_per_entity := NULL ]
|
||||||
|
|
||||||
|
# Shorter kinds
|
||||||
|
participants[ kind == "individual-person-with-significant-control", kind := "individual" ]
|
||||||
|
participants[ kind == "corporate-entity-person-with-significant-control", kind := "entity" ]
|
||||||
|
participants[ kind == "legal-person-person-with-significant-control", kind := "legal_person" ]
|
||||||
|
|
||||||
|
# Proper order and save point
|
||||||
|
setorderv(participants, c("company_number", "participant_id"))
|
||||||
|
|
||||||
|
# Save point
|
||||||
|
fwrite(participants, file = "output/uk/uk_organisations_participants_2021_long_2aug21.csv", eol = "\n")
|
|
@ -0,0 +1,125 @@
|
||||||
|
# This code processes the dump of
|
||||||
|
# CorpWatch EDGAR data to create
|
||||||
|
# a CSV with entity-participant
|
||||||
|
# mapping from SEC 10-K Exhibit 21
|
||||||
|
# that we will use in the study
|
||||||
|
library(data.table)
|
||||||
|
library(lubridate)
|
||||||
|
library(stringi)
|
||||||
|
library(stringr)
|
||||||
|
|
||||||
|
# Declare working directory beforehand in an environment variable
|
||||||
|
# ALPHAICON_PATH = "path_to_your_folder"
|
||||||
|
# with the aid of usethis::edit_r_environ()
|
||||||
|
# Restart R session for the changes to make effect
|
||||||
|
setwd(Sys.getenv('ALPHAICON_PATH'))
|
||||||
|
|
||||||
|
# Load the data from http://api.corpwatch.org/ dump
|
||||||
|
# (http://api.corpwatch.org/documentation/db_dump/)
|
||||||
|
|
||||||
|
## Company information (name, id, location)
|
||||||
|
## This information includes data on parent-subsidiary relationship
|
||||||
|
company_info <- fread("data/corpwatch_api_tables_csv_14aug21/company_info.csv", encoding = "UTF-8", na.strings = c("", "NULL"))
|
||||||
|
|
||||||
|
## Company locations
|
||||||
|
company_locations <- fread("data/corpwatch_api_tables_csv_14aug21/company_locations.csv", encoding = "UTF-8", na.strings = c("", "NULL"))
|
||||||
|
|
||||||
|
## Company name-id mapping
|
||||||
|
cik_name_lookup <- fread("data/corpwatch_api_tables_csv_14aug21/cik_name_lookup.csv", encoding = "UTF-8", na.strings = c("", "NULL"))
|
||||||
|
|
||||||
|
## Add locations to the company information
|
||||||
|
company_info[, country := company_locations[ match(company_info$best_location_id, company_locations$location_id) ]$country_code ]
|
||||||
|
|
||||||
|
### Extract subsidiaries of UK companies active in 2020--2021 (whichever comes latest)
|
||||||
|
uk_subsidiary_companies_filers <- company_info[ year >= 2020 & country == "GB" & top_parent_id != cw_id, c("year", "company_name", "cw_id", "num_parents", "num_children", "top_parent_id"), with = F]
|
||||||
|
### Get the latest available year
|
||||||
|
setorderv(uk_subsidiary_companies_filers, c("cw_id", "year"), c(1, -1))
|
||||||
|
uk_subsidiary_companies_filers <- unique(uk_subsidiary_companies_filers, by = c("cw_id"))
|
||||||
|
uk_subsidiary_companies_filers[, year := NULL ]
|
||||||
|
|
||||||
|
## Parent-subsidiary ID mapping
|
||||||
|
uk_parent_subsidiary_mapping <- unique(uk_subsidiary_companies_filers[, c("cw_id", "top_parent_id", "company_name")])
|
||||||
|
setnames(uk_parent_subsidiary_mapping, c("subsidiary_cw_id", "parent_cw_id", "subsidiary_name"))
|
||||||
|
|
||||||
|
# Add namesakes
|
||||||
|
## For subsidiaries
|
||||||
|
uk_parent_subsidiary_mapping <- merge(uk_parent_subsidiary_mapping, cik_name_lookup[, c("cw_id", "edgar_name")], by.x = "subsidiary_cw_id", by.y = "cw_id", all.x = T, all.y = F)
|
||||||
|
uk_parent_subsidiary_mapping[!is.na(edgar_name) & subsidiary_name != edgar_name, subsidiary_name := edgar_name ]
|
||||||
|
uk_parent_subsidiary_mapping[, edgar_name := NULL ]
|
||||||
|
## For parents
|
||||||
|
uk_parent_subsidiary_mapping[, parent_name := company_info[year >= 2020][ match(uk_parent_subsidiary_mapping$parent_cw_id, company_info[year >= 2020]$cw_id) ]$company_name ]
|
||||||
|
uk_parent_subsidiary_mapping <- merge(uk_parent_subsidiary_mapping, cik_name_lookup[, c("cw_id", "edgar_name")], by.x = "parent_cw_id", by.y = "cw_id", all.x = T, all.y = F)
|
||||||
|
uk_parent_subsidiary_mapping[ !is.na(edgar_name) & parent_name != edgar_name, parent_name := edgar_name ]
|
||||||
|
uk_parent_subsidiary_mapping[, edgar_name := NULL ]
|
||||||
|
|
||||||
|
uk_parent_subsidiary_mapping <- unique(uk_parent_subsidiary_mapping)
|
||||||
|
|
||||||
|
# Remove self-loops
|
||||||
|
uk_parent_subsidiary_mapping <- uk_parent_subsidiary_mapping[ subsidiary_cw_id != parent_cw_id ]
|
||||||
|
|
||||||
|
uniqueN(uk_parent_subsidiary_mapping$parent_cw_id) # 1071
|
||||||
|
uniqueN(uk_parent_subsidiary_mapping$subsidiary_cw_id) # 7634
|
||||||
|
|
||||||
|
# Match the parent-subsidiart data from SEC filings with PSC register on company names
|
||||||
|
|
||||||
|
## Load the companies data prepared by 1b_process_companies_data.r
|
||||||
|
load("data/uk/uk_basic_companies_data_2021-08-01.rdata")
|
||||||
|
|
||||||
|
## Load the participants panel prepared by 2_psc_snapshot_to_participants_panel.r
|
||||||
|
participants <- fread("output/uk/uk_organisations_participants_2021_long_2aug21.csv", encoding = "UTF-8", na.strings = "")
|
||||||
|
|
||||||
|
## Keep only entities-persons with significant control
|
||||||
|
participants <- participants[ kind %in% c("entity", "legal_person") ]
|
||||||
|
|
||||||
|
## Add the names to the participants panel
|
||||||
|
participants[, company_name := uk_basic_companies_data[ match(participants$company_number, uk_basic_companies_data$CompanyNumber) ]$CompanyName ]
|
||||||
|
participants[, participant_name := uk_basic_companies_data[ match(participants$participant_id, uk_basic_companies_data$CompanyNumber) ]$CompanyName ]
|
||||||
|
participants[, participant_name_bak := stri_split_fixed(participant_id, "$", n = 2, simplify = T)[,1] ]
|
||||||
|
participants[ is.na(participant_name) & !is.na(participant_name_bak), participant_name := participant_name_bak ]
|
||||||
|
participants[, participant_name_bak := NULL ]
|
||||||
|
|
||||||
|
## Reduce the names of companies
|
||||||
|
### Name cleaner function
|
||||||
|
name_cleaner <- function(x) {
|
||||||
|
|
||||||
|
out <- gsub("(['’\n ]|[[:punct:]]|[[:space:]]|[[:cntrl:]])+", " ", x, perl = T)
|
||||||
|
|
||||||
|
out <- gsub(" LIMITED", " LTD", out, perl = T, ignore.case = T)
|
||||||
|
|
||||||
|
out <- str_trim(out)
|
||||||
|
|
||||||
|
return(out)
|
||||||
|
|
||||||
|
}
|
||||||
|
|
||||||
|
### Remove punctuation, stopwords, strip whitespaces from company_names
|
||||||
|
participants[, company_name_reduced := name_cleaner(toupper(company_name))]
|
||||||
|
participants[, participant_name_reduced := name_cleaner(toupper(participant_name))]
|
||||||
|
|
||||||
|
uk_parent_subsidiary_mapping[, subsidiary_name_reduced := name_cleaner(toupper(subsidiary_name)) ]
|
||||||
|
uk_parent_subsidiary_mapping[, parent_name_reduced := name_cleaner(toupper(parent_name)) ]
|
||||||
|
|
||||||
|
# UK company number - name mapping excluding namesakes
|
||||||
|
uk_company_name_number_mapping <- unique(participants[, c("company_number", "company_name_reduced"), with = F])
|
||||||
|
uk_company_name_number_mapping[, count_namesakes := .N, by = "company_name_reduced" ]
|
||||||
|
uk_company_name_number_mapping <- uk_company_name_number_mapping[ count_namesakes == 1]
|
||||||
|
uk_company_name_number_mapping[, count_namesakes := NULL ]
|
||||||
|
|
||||||
|
# Participant id - participant name mapping excluding namesakes
|
||||||
|
uk_participant_name_id_mapping <- unique(participants[, c("participant_id", "participant_name_reduced"), with = F])
|
||||||
|
uk_participant_name_id_mapping[, count_namesakes := .N, by = "participant_name_reduced" ]
|
||||||
|
uk_participant_name_id_mapping <- uk_participant_name_id_mapping[ count_namesakes == 1]
|
||||||
|
uk_participant_name_id_mapping[, count_namesakes := NULL ]
|
||||||
|
|
||||||
|
# Add company numbers to UK subsidiary companies from SEC 10-K Exhibit 21
|
||||||
|
uk_parent_subsidiary_mapping[, company_number := uk_company_name_number_mapping[ match(uk_parent_subsidiary_mapping$subsidiary_name_reduced, uk_company_name_number_mapping$company_name_reduced) ]$company_number ]
|
||||||
|
|
||||||
|
# Add participant_ids to parent companies from SEC 10-K Exhibit 21
|
||||||
|
uk_parent_subsidiary_mapping[, participant_id := uk_participant_name_id_mapping[ match(uk_parent_subsidiary_mapping$parent_name_reduced, uk_participant_name_id_mapping$participant_name_reduced) ]$participant_id ]
|
||||||
|
|
||||||
|
# Final list with only matched entries, excluding
|
||||||
|
# namesakes
|
||||||
|
uk_parent_subsidiary_mapping <- unique(uk_parent_subsidiary_mapping[ !is.na(company_number) & !is.na(participant_id), c("company_number", "participant_id", "subsidiary_cw_id", "parent_cw_id") ], by = c("company_number", "participant_id"))
|
||||||
|
|
||||||
|
# Export point
|
||||||
|
fwrite(uk_parent_subsidiary_mapping, file = "data/uk/uk_parent_subsidiary_mapping_2020_2021_sec_filers_exhibit21.csv", eol = "\n")
|
248
code/helper_functions/compute_power_index.r
Normal file
248
code/helper_functions/compute_power_index.r
Normal file
|
@ -0,0 +1,248 @@
|
||||||
|
# This code implements Algorithm 1
|
||||||
|
# "Label propagation for NPI"
|
||||||
|
# from Mizuno, Doi, and Kurizaki (2020, p. 7)
|
||||||
|
# (https://doi.org/10.1371/journal.pone.0237862)
|
||||||
|
library(data.table)
|
||||||
|
|
||||||
|
# Function to compute Network or Direct power index
|
||||||
|
# INPUTS: a data.table with participant-entity relations
|
||||||
|
# in long form or an igraph object with edge weights
|
||||||
|
# OUTPUTS: data.table with power index for each participant
|
||||||
|
# or named list with such table and labels from each iteration
|
||||||
|
# (if save_labels = T).
|
||||||
|
|
||||||
|
compute_power_index <- function(relations_object,
|
||||||
|
participant_var = "participant",
|
||||||
|
entity_var = "entity",
|
||||||
|
weight_var = "weight",
|
||||||
|
powerindex = "dpi", # which power index to compute
|
||||||
|
iterations = 100, # how many iterations to run
|
||||||
|
quota = 50, # majority rule, %
|
||||||
|
epsilon = 0.01, # probability to restore the original label when computing the NPI
|
||||||
|
iterations_to_discard = 20, # how many iterations to discard when computing the NPI
|
||||||
|
save_labels = FALSE # whether to save labels from each iteration
|
||||||
|
) {
|
||||||
|
|
||||||
|
# Debug: powerindex <- "dpi"; iterations <- 100; quota <- 50; epsilon <- 0.01; iterations_to_discard <- 20; save_labels <- F; participant_var = "participant"; entity_var = "entity"; weight_var = "voting_share"
|
||||||
|
|
||||||
|
# Input checks
|
||||||
|
## Does the relations object exist and is it in correct form?
|
||||||
|
stopifnot("relations_object is not specified" = !missing(relations_object))
|
||||||
|
|
||||||
|
## If the relations object is in igraph transform to a data.frame
|
||||||
|
if( "igraph" %in% class(relations_object) ) {
|
||||||
|
|
||||||
|
relations_object <- igraph::as_data_frame(relations_object, what = "edges")
|
||||||
|
|
||||||
|
# Check whether a weight column is present in the data (i.e. data.frame has at least 3 columns
|
||||||
|
stopifnot("`relations_object` graph does not have edge weights" = ncol(relations_object) >= 3)
|
||||||
|
|
||||||
|
# Proper names
|
||||||
|
names(relations_object)[1:2] <- c(participant_var, entity_var)
|
||||||
|
|
||||||
|
}
|
||||||
|
|
||||||
|
## If the relations object is not a data.table try to coerce to it
|
||||||
|
if( !("data.table" %in% class(relations_object)) ) {
|
||||||
|
|
||||||
|
data.table::setDT(relations_object)
|
||||||
|
|
||||||
|
}
|
||||||
|
|
||||||
|
## Are the participant and entity variables in the data?
|
||||||
|
stopifnot("`participant_var` variable not found in `relations_object`" = participant_var %in% names(relations_object))
|
||||||
|
stopifnot("`entity_var` variable not found in `relations_object`" = entity_var %in% names(relations_object))
|
||||||
|
stopifnot("`weight_var` variable not found in `relations_object`" = weight_var %in% names(relations_object))
|
||||||
|
|
||||||
|
## Are the power index options correctly specified?
|
||||||
|
stopifnot("`powerindex` should be either dpi or npi" = powerindex %in% c("npi", "dpi"))
|
||||||
|
stopifnot("`save_labels` should be either TRUE or FALSE" = save_labels %in% c(TRUE, FALSE))
|
||||||
|
|
||||||
|
## Are the parameters of the required type?
|
||||||
|
stopifnot("`iterations should` be an integer" = all.equal(iterations, as.integer(iterations)) )
|
||||||
|
stopifnot("`iterations_to_discard` should be an integer not exceeding `iterations`" = (all.equal(iterations_to_discard, as.integer(iterations_to_discard)) & iterations_to_discard < iterations) | powerindex == "dpi" )
|
||||||
|
min_weight <- min(relations_object[[weight_var]], na.rm = T)
|
||||||
|
max_weight <- max(relations_object[[weight_var]], na.rm = T)
|
||||||
|
stopifnot("`weight_var` should take non-negative numeric values" = min_weight >= 0 & is.finite(max_weight) & is.finite(min_weight) )
|
||||||
|
stopifnot("epsilon should be in [0,1] range" = (epsilon >= 0 & epsilon <= 1) | powerindex == "dpi" )
|
||||||
|
|
||||||
|
## Disable the `iterations_to_discard` option
|
||||||
|
## when computing DPI
|
||||||
|
if( powerindex == "dpi" ) {
|
||||||
|
|
||||||
|
iterations_to_discard <- 0
|
||||||
|
|
||||||
|
}
|
||||||
|
|
||||||
|
# Remove observations with missing data
|
||||||
|
## Participant
|
||||||
|
if( nrow(relations_object[ is.na(get(participant_var)) ]) > 0 ) {
|
||||||
|
|
||||||
|
warning(paste0("Removing rows with missing ", participant_var, " before computation"))
|
||||||
|
relations_object <- relations_object[ !is.na(get(participant_var)) ]
|
||||||
|
|
||||||
|
}
|
||||||
|
## Entity
|
||||||
|
if( nrow(relations_object[ is.na(get(entity_var)) ]) > 0 ) {
|
||||||
|
|
||||||
|
warning(paste0("Removing rows with missing ", entity_var, " before computation"))
|
||||||
|
relations_object <- relations_object[ !is.na(get(entity_var)) ]
|
||||||
|
|
||||||
|
}
|
||||||
|
## Weight
|
||||||
|
if( nrow(relations_object[ is.na(get(weight_var)) ]) > 0 ) {
|
||||||
|
|
||||||
|
warning(paste0("Removing rows with missing ", weight_var, " before computation"))
|
||||||
|
relations_object <- relations_object[ !is.na(get(weight_var)) ]
|
||||||
|
|
||||||
|
}
|
||||||
|
|
||||||
|
# Init an object to update labels in each iteration
|
||||||
|
updating_relations <- copy(relations_object[, c(participant_var, entity_var, weight_var), with = F])
|
||||||
|
setnames(updating_relations, c("participant", "entity", "weight"))
|
||||||
|
|
||||||
|
# Init an object to store per-iteration
|
||||||
|
# entity labels (i.e. who the pivotal
|
||||||
|
# shareholder is)
|
||||||
|
if( save_labels == T) {
|
||||||
|
|
||||||
|
iteration_labels <- data.table()
|
||||||
|
|
||||||
|
# Otherwise we store overall counts of each
|
||||||
|
# label being named a pivot
|
||||||
|
} else {
|
||||||
|
|
||||||
|
pivot_counts <- updating_relations[, c("participant", "entity")]
|
||||||
|
pivot_counts[, times_pivotal := 0 ]
|
||||||
|
|
||||||
|
}
|
||||||
|
|
||||||
|
# Create a progress bar to report on iteration progess
|
||||||
|
message(paste0("Iterating through ", iterations, " permutations:"))
|
||||||
|
iterations_progess_bar <- txtProgressBar(min = 0, max = iterations, style = 3)
|
||||||
|
|
||||||
|
for(i in 1:iterations) {
|
||||||
|
|
||||||
|
# Randomly order rows within entities
|
||||||
|
updating_relations[, random_var := runif(.N)]
|
||||||
|
setorderv(updating_relations, c("entity", "random_var"))
|
||||||
|
|
||||||
|
# Breaking cycles when computing NPI: restore original
|
||||||
|
# labels for the random set of rows with epsilon
|
||||||
|
# probability
|
||||||
|
if( powerindex == "npi" & epsilon > 0 ) {
|
||||||
|
|
||||||
|
entities_to_restore <- unique(updating_relations[ random_var <= epsilon ]$entity)
|
||||||
|
|
||||||
|
if( length(entities_to_restore) > 0 ) {
|
||||||
|
|
||||||
|
restored_entities <- relations_object[ get(entity_var) %in% entities_to_restore, c(participant_var, entity_var, weight_var), with = F]
|
||||||
|
setnames(restored_entities, c("participant", "entity", "weight"))
|
||||||
|
|
||||||
|
# Randomly shuffle the labels to restore as well respect random ordering
|
||||||
|
restored_entities[, random_var := runif(.N) ]
|
||||||
|
setorderv(restored_entities, c("entity", "random_var"))
|
||||||
|
|
||||||
|
updating_relations <- rbind(updating_relations[ !( entity %in% entities_to_restore) ],
|
||||||
|
restored_entities,
|
||||||
|
fill = T)
|
||||||
|
|
||||||
|
}
|
||||||
|
|
||||||
|
}
|
||||||
|
# Compute cumulative sums of voting rights by entity
|
||||||
|
updating_relations[, cumulative_weight := cumsum(weight), by = "entity"]
|
||||||
|
|
||||||
|
# Lagged cumulative voting share
|
||||||
|
# shift() is not optimized with many groups
|
||||||
|
# (https://github.com/Rdatatable/data.table/issues/1534)
|
||||||
|
# so we cannot simply use `by` statement as below
|
||||||
|
# updating_relations[, cumulative_weight_l1 := shift(cumulative_weight, type = "lag", n = 1), by = "entity"]
|
||||||
|
#
|
||||||
|
# Instead, consider a two-step hack that uses our entity ordering
|
||||||
|
# to mark as missing lagged weights for the first participant per entity
|
||||||
|
updating_relations[, cumulative_weight_l1 := shift(cumulative_weight, type = "lag", n = 1)]
|
||||||
|
updating_relations[, entity_l1 := shift(entity, type = "lag", n = 1)]
|
||||||
|
updating_relations[entity != entity_l1, cumulative_weight_l1 := NA_real_ ]
|
||||||
|
|
||||||
|
# Identify pivots
|
||||||
|
pivots <- updating_relations[ cumulative_weight >= quota & (cumulative_weight_l1 < quota | is.na(cumulative_weight_l1) ), c("participant", "entity") ]
|
||||||
|
|
||||||
|
# Store pivots from each iteration in a designated object
|
||||||
|
if( save_labels == T) {
|
||||||
|
|
||||||
|
iteration_labels <- rbind(iteration_labels, data.table(iteration = i, pivots), fill = T)
|
||||||
|
|
||||||
|
# NB: ^ this is the most problematic step in terms of scaling.
|
||||||
|
# Where to store and access an N * number of iterations table
|
||||||
|
# with labels when N is huge and iterations = 20 000? We need
|
||||||
|
# to save pivots for ~3 million entities 20 thousand times.
|
||||||
|
#
|
||||||
|
# Alternatively you can write pivots from each iteration to a separate CSV
|
||||||
|
# but you will still end up with 20 thousand files, each having ~3 million lines.
|
||||||
|
|
||||||
|
}
|
||||||
|
|
||||||
|
# We can avoid storing the pivots altogether and just
|
||||||
|
# add to the total count of times a given partipant is
|
||||||
|
# named pivotal in a given entity. We also discard
|
||||||
|
# the first `iterations_to_discard` iterations
|
||||||
|
if( save_labels == F & i > iterations_to_discard ) {
|
||||||
|
|
||||||
|
# We row bind the current pivots to the existing counts
|
||||||
|
# and then sum. This might be faster than merging on
|
||||||
|
# entity and participant.
|
||||||
|
# In fact, it is very slow
|
||||||
|
pivot_counts <- rbind(pivot_counts, data.table(pivots, times_pivotal = 1), fill = T)
|
||||||
|
pivot_counts <- pivot_counts[, list( times_pivotal = sum(times_pivotal) ), by = c("entity", "participant")]
|
||||||
|
|
||||||
|
}
|
||||||
|
|
||||||
|
# When computing NPI: update the participant
|
||||||
|
# labels with pivots identified during this
|
||||||
|
# iteration step
|
||||||
|
if( powerindex == "npi" ) {
|
||||||
|
|
||||||
|
updating_relations[, participant_update := pivots[ match(updating_relations$participant, pivots$entity) ]$participant ]
|
||||||
|
updating_relations[ !is.na(participant_update), participant := participant_update ]
|
||||||
|
updating_relations[, participant_update := NA ]
|
||||||
|
|
||||||
|
# Sum up the updated shares for the next iteration
|
||||||
|
updating_relations <- updating_relations[, list(weight = sum(weight)), by = c("entity", "participant")]
|
||||||
|
|
||||||
|
}
|
||||||
|
|
||||||
|
# Update the progress bar
|
||||||
|
setTxtProgressBar(iterations_progess_bar, i)
|
||||||
|
|
||||||
|
}
|
||||||
|
|
||||||
|
# Compute the index itself
|
||||||
|
if( save_labels == T ) {
|
||||||
|
|
||||||
|
# Count the number of times this
|
||||||
|
# participant was named the pivotal player,
|
||||||
|
# discarding first `iterations_to_discard` iterations
|
||||||
|
index_values <- iteration_labels[iteration > iterations_to_discard, list(times_pivotal = .N), by = c("entity", "participant")]
|
||||||
|
|
||||||
|
} else {
|
||||||
|
|
||||||
|
index_values <- pivot_counts
|
||||||
|
|
||||||
|
}
|
||||||
|
|
||||||
|
# Compute the index
|
||||||
|
index_values[, index := times_pivotal/(iterations - iterations_to_discard) ]
|
||||||
|
setnames(index_values, "index", powerindex)
|
||||||
|
|
||||||
|
if( save_labels == T ) {
|
||||||
|
|
||||||
|
return(list(index_values = index_values, iteration_labels = iteration_labels))
|
||||||
|
|
||||||
|
} else {
|
||||||
|
|
||||||
|
return(index_values)
|
||||||
|
|
||||||
|
}
|
||||||
|
|
||||||
|
}
|
22
code/helper_functions/install_dependencies.r
Normal file
22
code/helper_functions/install_dependencies.r
Normal file
|
@ -0,0 +1,22 @@
|
||||||
|
# Install the required dependencies
|
||||||
|
|
||||||
|
# Declare working directory beforehand in an environment variable
|
||||||
|
# ALPHAICON_PATH = "path_to_your_folder"
|
||||||
|
# with the aid of usethis::edit_r_environ()
|
||||||
|
# Restart R session for the changes to make effect
|
||||||
|
setwd(Sys.getenv('ALPHAICON_PATH'))
|
||||||
|
|
||||||
|
# Read in the file with dependencies
|
||||||
|
packages <- readLines(con <- file("DEPENDENCIES", encoding = "UTF-8"))
|
||||||
|
packages <- gsub("^\\s+", "", packages[2:length(packages)])
|
||||||
|
packages <- gsub("\\s+.*$", "", packages)
|
||||||
|
|
||||||
|
# Install the uninstalled packages
|
||||||
|
missing_packages <- setdiff(packages, rownames(installed.packages()))
|
||||||
|
|
||||||
|
if ( length(missing_packages) > 0 ) {
|
||||||
|
|
||||||
|
# Install the latest versions of all the packages
|
||||||
|
install.packages(missing_packages, quiet = T)
|
||||||
|
|
||||||
|
}
|
0
data/uk/BasicCompanyDataAsOneFile-2021-08-01.csv
Normal file
0
data/uk/BasicCompanyDataAsOneFile-2021-08-01.csv
Normal file
|
0
data/uk/psc_snapshot_2021-08-02.rdata
Normal file
0
data/uk/psc_snapshot_2021-08-02.rdata
Normal file
0
data/uk/sic_2007_code_list.csv
Normal file
0
data/uk/sic_2007_code_list.csv
Normal file
|
0
data/uk/uk_basic_companies_data_2021-08-01.rdata
Normal file
0
data/uk/uk_basic_companies_data_2021-08-01.rdata
Normal file
BIN
output/alphaicon_paper/core_plot.pdf
Normal file
BIN
output/alphaicon_paper/core_plot.pdf
Normal file
Binary file not shown.
BIN
output/alphaicon_paper/network_examples/bajlinder.pdf
Normal file
BIN
output/alphaicon_paper/network_examples/bajlinder.pdf
Normal file
Binary file not shown.
BIN
output/alphaicon_paper/network_examples/berkeley_group.pdf
Normal file
BIN
output/alphaicon_paper/network_examples/berkeley_group.pdf
Normal file
Binary file not shown.
BIN
output/alphaicon_paper/network_examples/opus_graph.pdf
Normal file
BIN
output/alphaicon_paper/network_examples/opus_graph.pdf
Normal file
Binary file not shown.
BIN
output/alphaicon_paper/network_examples/specsavers.pdf
Normal file
BIN
output/alphaicon_paper/network_examples/specsavers.pdf
Normal file
Binary file not shown.
BIN
output/alphaicon_paper/recall_path_plot_at_1.pdf
Normal file
BIN
output/alphaicon_paper/recall_path_plot_at_1.pdf
Normal file
Binary file not shown.
BIN
output/alphaicon_paper/recall_path_plot_at_5.pdf
Normal file
BIN
output/alphaicon_paper/recall_path_plot_at_5.pdf
Normal file
Binary file not shown.
BIN
output/alphaicon_paper/recall_plot.pdf
Normal file
BIN
output/alphaicon_paper/recall_plot.pdf
Normal file
Binary file not shown.
BIN
output/alphaicon_paper/recall_plot_alphas.pdf
Normal file
BIN
output/alphaicon_paper/recall_plot_alphas.pdf
Normal file
Binary file not shown.
|
@ -0,0 +1,101 @@
|
||||||
|
participant_id,participant_name,ranktransitive,ranknpi,rankdpi,sumtransitive,sumnpi,sumdpi,nodes,SH,ST,I,C
|
||||||
|
SPECSAVERS OPTICAL SUPERSTORES LTD$UNITED KINGDOM$1721624,SPECSAVERS OPTICAL SUPERSTORES LTD,1,1,42441,1482.2,1453,4,1767,188,1159,420,0
|
||||||
|
PETER$NA$VALAITIS$1950$11,PETER VALAITIS,2,2,1,1392,1392,1391,1393,1,1390,2,0
|
||||||
|
PARTNERS GROUP HOLDING AG$SWITZERLAND$CHE-108.562.489,PARTNERS GROUP HOLDING AG,3,3,2,1166.5,1166.5,1164.5,1170,2,1167,1,0
|
||||||
|
AVTAR$NA$SINGH$1967$8,AVTAR SINGH,4,4,4,1051,1051,1051,1055,3,1052,0,0
|
||||||
|
ANDREW$SIMON$DAVIS$1963$7,ANDREW DAVIS,5,6,2788,881.3,869.5,14.5,1020,107,895,18,0
|
||||||
|
ROBERT$ANTHONY$JARRETT$1974$8,ROBERT JARRETT,6,5,5,873,873,873,874,1,873,0,0
|
||||||
|
SC095000,LLOYDS BANKING GROUP PLC,7,8,24872,735.6,670.5,5,956,35,596,325,0
|
||||||
|
CHRIS$NA$HADJIOANNOU$1981$1,CHRIS HADJIOANNOU,8,7,6,730,730,730,731,1,730,0,0
|
||||||
|
11443992,BRIDGEPOINT GROUP PLC,9,10,5149,669.3,591.5,11,808,13,536,259,0
|
||||||
|
READE$NA$GRIFFITH$1965$4,READE GRIFFITH,10,9,491331,657.5,636.5,1.5,868,25,374,469,0
|
||||||
|
PERSIMMON PLC$ENGLAND$1818486,PERSIMMON PLC,11,11,2911,586,578.4,14,617,4,561,52,0
|
||||||
|
INTERTRUST N.V.$THE NETHERLANDS$N/A,INTERTRUST N.V.,12,12,887094,519.7,511.8,1,530,4,353,173,0
|
||||||
|
IWG PLC$JERSEY$122154,IWG PLC,13,13,10,481.8,481.7,422,497,6,473,18,0
|
||||||
|
00604574,BARRATT DEVELOPMENTS P L C,14,17,587,481.1,473.6,31,530,9,446,75,0
|
||||||
|
06035106,FRASERS GROUP PLC,15,16,2982,480.1,474.8,14,484,2,438,44,0
|
||||||
|
GRZEGORZ$NA$SZEWCZYK$1985$7,GRZEGORZ SZEWCZYK,16,14,8,480,480,480,481,1,480,0,0
|
||||||
|
DARREN$NA$SYMES$1958$10,DARREN SYMES,17,15,9,475.5,475.5,474.5,478,2,475,1,0
|
||||||
|
08885072,PETS AT HOME GROUP PLC,18,19,1226949,468,389.1,1,770,216,514,40,0
|
||||||
|
10657226,BGF GROUP PLC,19,23,6219,462.1,343,10,674,99,378,197,0
|
||||||
|
03981143,OCTOPUS CAPITAL LIMITED,20,18,1624,414.5,411.7,19,431,4,310,117,0
|
||||||
|
11444019,M&G PLC,21,20,17408,404,386.6,6,469,14,298,157,0
|
||||||
|
00102498,BP P.L.C.,22,26,1757,377.8,336.4,18,493,5,390,96,2
|
||||||
|
10510800,IVC ACQUISITION PIKCO LTD,23,21,1535931,370,366.3,1,371,1,341,29,0
|
||||||
|
SUN LIFE FINANCIAL INC.$CANADA$F380,SUN LIFE FINANCIAL INC.,24,22,2086664,364.7,358.2,1,469,10,242,217,0
|
||||||
|
03777037,PLACES FOR PEOPLE GROUP LIMITED,25,25,458,345.8,338.7,35.5,387,11,324,52,0
|
||||||
|
KANE$STEVEN JAMES$WALLACE$1988$4,KANE WALLACE,26,24,12,342,342,342,343,1,342,0,0
|
||||||
|
01417162,LEGAL & GENERAL GROUP PLC,27,28,3477,333.1,321,13,399,14,286,99,0
|
||||||
|
04569346,DIGNITY PLC,28,27,45566,332.9,328.5,4,348,13,284,51,0
|
||||||
|
02468686,AVIVA PLC,29,29,58547,316.1,305.8,4,372,11,261,100,0
|
||||||
|
00621920,BRITISH LAND COMPANY PUBLIC LIMITED COMPANY(THE),30,30,11060,301.8,295.8,7.5,315,1,218,96,0
|
||||||
|
01142830,3I GROUP PLC,31,35,1948,290.6,276.4,17.3,392,12,176,204,0
|
||||||
|
00296805,TAYLOR WIMPEY PLC,32,33,6642,289.9,283.3,9.5,339,11,260,68,0
|
||||||
|
00082548,REACH PLC,33,31,365,289.4,286.7,39,297,1,229,67,0
|
||||||
|
BRYAN$ANTHONY$THORNTON$1955$7,BRYAN THORNTON,34,32,135,287.3,284.3,70,299,6,290,3,0
|
||||||
|
08286875,WPP JUBILEE LIMITED,35,36,268,284.8,271.2,45.8,314,6,200,108,0
|
||||||
|
RONALD$TERENCE$RICHARDS$1945$1,RONALD RICHARDS,36,34,17,280,280,280,281,1,280,0,0
|
||||||
|
02877315,REDROW PLC,37,37,176901,273.2,269.7,2,292,5,268,19,0
|
||||||
|
WOODLEY$LARKIN$HUNT$1945$11,WOODLEY HUNT,38,39,1331918,261.3,256.5,1,287,2,154,131,0
|
||||||
|
HANNOVER RUCK SE$GERMANY$HRB 6778,HANNOVER RUCK SE,39,38,82217,261,258.4,3,262,1,254,7,0
|
||||||
|
01470151,BAE SYSTEMS PLC,40,42,387,252.9,251.4,38,301,7,206,88,0
|
||||||
|
ANDREW$ROY$LEIGHTON$1949$10,ANDREW LEIGHTON,41,41,38,252.3,251.4,159.3,256,3,251,2,0
|
||||||
|
08340090,COUNTRYWIDE LIMITED,42,43,785896,248,245.5,1,249,1,217,31,0
|
||||||
|
04967001,ITV PLC,43,46,71002,247.9,242.3,3.2,274,16,209,49,0
|
||||||
|
10763268,CSC FINANCIAL SERVICES HOLDINGS LIMITED,44,44,306820,247.7,245,2,250,1,161,88,0
|
||||||
|
HEIDELBERGCEMENT AG$GERMANY$HRB 33082,HEIDELBERGCEMENT AG,45,48,210440,246.4,236.1,2,260,3,166,91,0
|
||||||
|
STEPHEN$ALLEN$SCHWARZMAN$1947$2,STEPHEN SCHWARZMAN,46,45,96,244.7,243.4,90,256,3,171,82,0
|
||||||
|
OC301825,HGCAPITAL LLP,47,47,2009,242.2,239.1,17,247,4,164,79,0
|
||||||
|
OC360196,EQUISTONE LLP,48,40,242598,241.6,252.4,2,261,4,167,90,0
|
||||||
|
03140521,MATTIOLI WOODS PLC,49,55,453,241.1,226.6,35.5,290,22,181,87,0
|
||||||
|
STEPHEN$STUART SOLOMON$CONWAY$1948$2,STEPHEN CONWAY,50,49,5851,237.5,233.6,10,317,14,230,73,0
|
||||||
|
CRH PLC$IRELAND$12965,CRH PLC,51,50,617583,235.4,230.1,1,268,7,201,60,0
|
||||||
|
OC332179,DRUCES LLP,52,52,183094,230.3,227.7,2,234,3,229,2,0
|
||||||
|
BELLWAY P.L.C.$ENGLAND$1372603,BELLWAY P.L.C.,53,53,1279,229.5,227.3,21,253,4,236,13,0
|
||||||
|
SEMPERIAN PPP INVESTMENT PARTNERS HOLDINGS LIMITED$NA$NA,SEMPERIAN PPP INVESTMENT PARTNERS HOLDINGS LIMITED,54,51,961566,229.2,229.5,1,270,4,151,115,0
|
||||||
|
MICHAEL$JOHN$HALL$1971$7,MICHAEL HALL,55,54,22,225.3,226.9,226.8,239,5,234,0,0
|
||||||
|
00521970,MORGAN SINDALL GROUP PLC,56,61,969,217.9,209.6,24,298,16,205,77,0
|
||||||
|
BENZION$SCHALOM ELIEZER$FRESHWATER$1948$4,BENZION FRESHWATER,57,56,1526,215.6,216.4,19.5,257,6,222,29,0
|
||||||
|
MICHAEL$BARRY$TABOR$1941$10,MICHAEL TABOR,58,60,194884,214.8,211.1,2,226,4,161,61,0
|
||||||
|
00306718,VISTRY GROUP PLC,59,59,173704,213.8,211.2,2,260,7,223,30,0
|
||||||
|
SC045551,NATWEST GROUP PLC,60,58,42589,213.6,211.5,4,261,8,173,79,1
|
||||||
|
06397765,OCTOPUS TITAN VCT PLC,61,62,11088,211.1,208.7,7.5,221,4,124,93,0
|
||||||
|
RADOVAN$NA$KOLJEVIC$1967$5,RADOVAN KOLJEVIC,62,63,47760,211,208.4,4,216,4,204,8,0
|
||||||
|
05172586,THE BERKELEY GROUP HOLDINGS PLC,63,64,619407,209.2,207.2,1,216,1,202,13,0
|
||||||
|
SENTTHURAN$NA$NADARAJAH$1979$11,SENTTHURAN NADARAJAH,64,66,3685,207,205.1,13,208,1,206,1,0
|
||||||
|
JOAQUIM$NA$MAGRO DE ALMEIDA$1949$1,JOAQUIM MAGRO DE ALMEIDA,65,65,27,205.8,205.5,205.5,216,4,212,0,0
|
||||||
|
OLUWOLE$NA$KOLADE$1966$11,OLUWOLE KOLADE,66,70,57071,204.4,197.2,4,235,10,97,128,0
|
||||||
|
DAVID$JAMES$BARRICK$1984$1,DAVID BARRICK,67,57,25,201,213.5,213.6,254,1,251,2,0
|
||||||
|
STACEY$LOUISE$TRAMASEUR$1997$1,STACEY TRAMASEUR,68,67,29,201,201,201,202,1,201,0,0
|
||||||
|
ROMY$ELIZABETH$SUMMERSKILL$1967$1,ROMY SUMMERSKILL,69,68,15243,200.9,199.1,6.5,217,2,142,73,0
|
||||||
|
JAY$NA$THOMAS$1981$1,JAY THOMAS,70,69,30,199,199,199,200,1,199,0,0
|
||||||
|
SC286832,ABRDN PLC,71,74,1623,194,189.6,19,204,5,157,42,0
|
||||||
|
04120344,WHITBREAD PLC,72,71,592751,193.5,191,1,200,3,170,27,0
|
||||||
|
KEITH$NA$BRESLAUER$1966$3,KEITH BRESLAUER,73,72,71852,192.9,190.1,3,200,2,131,67,0
|
||||||
|
OC313101,AUGUST EQUITY LLP,74,73,5699,191.8,189.7,10,194,2,97,92,3
|
||||||
|
PATRICK$ANTHONY$MCKENNA$1956$6,PATRICK MCKENNA,75,79,13432,185,182.9,7,197,4,167,26,0
|
||||||
|
GUY$NA$HANDS$1959$8,GUY HANDS,76,77,3793,183.4,183.9,12.5,203,3,157,43,0
|
||||||
|
REBECCA$LOUISE$CODY$1997$7,REBECCA CODY,77,80,33,182,182,182,183,1,182,0,0
|
||||||
|
JEAN-GUY$NA$DESJARDINS$1944$10,JEAN-GUY DESJARDINS,78,88,9585,180.7,165.9,8,298,12,208,78,0
|
||||||
|
00445790,TESCO PLC,79,82,1527,180.4,178.2,19.5,199,4,132,63,0
|
||||||
|
STEPHEN$JAMES$ROBERTSON$1960$3,STEPHEN ROBERTSON,80,76,156655,178.2,186.6,2.3,383,14,269,100,0
|
||||||
|
MANJIT$NA$DALE$1965$6,MANJIT DALE,81,92,162072,178.2,163.9,2.3,383,14,269,100,0
|
||||||
|
THE BERKELEY GROUP PLC$ENGLAND & WALES$1454064,THE BERKELEY GROUP PLC,82,81,381,177.4,178.2,38.5,208,2,165,41,0
|
||||||
|
MOHAMMED$NA$AYYAZ$1996$12,MOHAMMED AYYAZ,83,83,35,177.3,177.3,177.3,222,30,192,0,0
|
||||||
|
CERI$NA$JOHN$1955$7,CERI JOHN,84,84,36,176,176,176,177,1,176,0,0
|
||||||
|
04330127,WILMINGTON TRUST (UK) LIMITED,85,85,185351,174.7,172.4,2,180,2,123,55,0
|
||||||
|
08379990,LIBERTY GLOBAL PLC,86,96,4578,172.6,162.3,11.5,335,11,239,85,0
|
||||||
|
VISTRY (JERSEY) LIMITED$JERSEY$130175,VISTRY (JERSEY) LIMITED,87,99,672013,171.8,161.5,1,243,10,186,47,0
|
||||||
|
07934492,CURTIS BANKS GROUP PLC,88,87,9563,170.8,168.2,8,181,4,161,16,0
|
||||||
|
00617987,HSBC HOLDINGS PLC,89,102,2511,170.8,160.2,15.2,220,6,150,64,0
|
||||||
|
BROOKFIELD ASSET MANAGEMENT INC$CANADA$/,BROOKFIELD ASSET MANAGEMENT INC,90,75,555776,170.5,186.6,1,347,4,225,118,0
|
||||||
|
QATAR INVESTMENT AUTHORITY$QATAR$/,QATAR INVESTMENT AUTHORITY,91,114,2818663,170.5,150.9,1,347,4,225,118,0
|
||||||
|
06394563,ENDEMOL SHINE GROUP HOLDING UK LIMITED,92,86,78751,170,168.3,3,175,3,142,30,0
|
||||||
|
OC333409,TRICOR SERVICES EUROPE LLP,93,91,42092,169.8,164.1,4,221,21,159,41,0
|
||||||
|
SOFTBANK GROUP CORP$JAPAN$0104-01-056795,SOFTBANK GROUP CORP,94,93,80640,168.7,163.8,3,181,5,101,75,0
|
||||||
|
07023200,ADP PRIMARY CARE SERVICES LIMITED,95,89,962994,167.7,165.8,1,170,1,134,35,0
|
||||||
|
03199160,THE UNITE GROUP PLC,96,94,42466,164.3,162.7,4,168,2,134,32,0
|
||||||
|
11499533,SCOOBY BIDCO LIMITED,97,97,1443396,163.9,162.2,1,172,3,143,26,0
|
||||||
|
00349201,ST. MODWEN PROPERTIES PLC,98,90,195,162.4,164.7,56,200,6,164,30,0
|
||||||
|
11606773,PHOENIX GROUP HOLDINGS PLC,99,98,93217,161.8,162.1,3,177,3,134,40,0
|
||||||
|
ANDRZEJ$NA$NIEMYJSKI$1975$11,ANDRZEJ NIEMYJSKI,100,100,37,161.5,161.5,161.5,164,2,162,0,0
|
|
|
@ -0,0 +1,101 @@
|
||||||
|
participant_id,sumdpi,rankdpi,sumnpi,ranknpi,sumtransitive,ranktransitive,diff_npi_dpi,diff_transitive_dpi,diff_transitive_npi
|
||||||
|
SPECSAVERS OPTICAL SUPERSTORES LTD$UNITED KINGDOM$1721624,4,42441,1452.95022222222,1,1482.18842011308,1,1448.95022222222,1478.18842011308,29.2381978908597
|
||||||
|
ANDREW$SIMON$DAVIS$1963$7,14.5042,2788,869.487555555556,6,881.3365356159,5,854.983355555556,866.8323356159,11.8489800603431
|
||||||
|
SC095000,5,24872,670.525666666667,8,735.610780469834,7,665.525666666667,730.610780469834,65.0851138031673
|
||||||
|
READE$NA$GRIFFITH$1965$4,1.4972,491331,636.465111111111,9,657.49955601355,10,634.967911111111,656.00235601355,21.0344449024385
|
||||||
|
11443992,11,5149,591.460888888889,10,669.338549583877,9,580.460888888889,658.338549583877,77.8776606949871
|
||||||
|
PERSIMMON PLC$ENGLAND$1818486,14,2911,578.420555555556,11,586.035982151633,11,564.420555555556,572.035982151633,7.6154265960771
|
||||||
|
INTERTRUST N.V.$THE NETHERLANDS$N/A,1,887094,511.782222222222,12,519.652355682228,12,510.782222222222,518.652355682228,7.87013346000617
|
||||||
|
06035106,14,2982,474.829444444445,16,480.114910906034,15,460.829444444445,466.114910906034,5.28546646158912
|
||||||
|
00604574,31,587,473.602,17,481.125633609192,14,442.602,450.125633609192,7.5236336091923
|
||||||
|
03981143,19,1624,411.697111111111,18,414.491202616254,20,392.697111111111,395.491202616254,2.79409150514357
|
||||||
|
08885072,1,1226949,389.079222222222,19,468.031302647188,18,388.079222222222,467.031302647188,78.9520804249655
|
||||||
|
11444019,6,17408,386.593444444445,20,403.985152490125,21,380.593444444445,397.985152490125,17.3917080456807
|
||||||
|
10510800,1,1535931,366.270333333333,21,370,23,365.270333333333,369,3.72966666666673
|
||||||
|
SUN LIFE FINANCIAL INC.$CANADA$F380,1,2086664,358.225444444444,22,364.651221643269,24,357.225444444444,363.651221643269,6.42577719882496
|
||||||
|
10657226,10,6219,342.955333333334,23,462.050947342302,19,332.955333333334,452.050947342302,119.095614008968
|
||||||
|
04569346,4,45566,328.479,27,332.883401968393,28,324.479,328.883401968393,4.40440196839307
|
||||||
|
00102498,18,1757,336.391666666667,26,377.785641323169,22,318.391666666667,359.785641323169,41.3939746565019
|
||||||
|
01417162,13,3477,320.993555555555,28,333.114660129009,27,307.993555555555,320.114660129009,12.121104573454
|
||||||
|
03777037,35.4911,458,338.669111111111,25,345.770230509207,25,303.178011111111,310.279130509207,7.10111939809553
|
||||||
|
02468686,3.9928,58547,305.836888888889,29,316.091281388984,29,301.844088888889,312.098481388984,10.2543925000952
|
||||||
|
00621920,7.5086,11060,295.787333333333,30,301.826105187632,30,288.278733333333,294.317505187632,6.03877185429838
|
||||||
|
00296805,9.5097,6642,283.340333333333,33,289.902171627357,32,273.830633333333,280.392471627357,6.56183829402386
|
||||||
|
02877315,2,176901,269.693888888889,37,273.241267886102,37,267.693888888889,271.241267886102,3.54737899721323
|
||||||
|
01142830,17.3204,1948,276.395222222222,35,290.62995167509,31,259.074822222222,273.30955167509,14.2347294528681
|
||||||
|
WOODLEY$LARKIN$HUNT$1945$11,1,1331918,256.523555555556,39,261.339361555954,38,255.523555555556,260.339361555954,4.81580600039837
|
||||||
|
HANNOVER RUCK SE$GERMANY$HRB 6778,3,82217,258.389888888889,38,261,39,255.389888888889,258,2.61011111111122
|
||||||
|
OC360196,2,242598,252.407222222222,40,241.635711925833,48,250.407222222222,239.635711925833,-10.7715102963891
|
||||||
|
00082548,39,365,286.73,31,289.415186936244,33,247.73,250.415186936244,2.68518693624372
|
||||||
|
08340090,1,785896,245.526666666667,43,248,42,244.526666666667,247,2.47333333333344
|
||||||
|
10763268,2,306820,245.016666666667,44,247.665776742879,44,243.016666666667,245.665776742879,2.6491100762122
|
||||||
|
04967001,3.1677,71002,242.283111111111,46,247.855191284282,43,239.115411111111,244.687491284282,5.57208017317043
|
||||||
|
HEIDELBERGCEMENT AG$GERMANY$HRB 33082,2,210440,236.095777777778,48,246.443097797991,45,234.095777777778,244.443097797991,10.3473200202133
|
||||||
|
CRH PLC$IRELAND$12965,1,617583,230.075888888889,50,235.350874688043,51,229.075888888889,234.350874688043,5.27498579915408
|
||||||
|
SEMPERIAN PPP INVESTMENT PARTNERS HOLDINGS LIMITED$NA$NA,1,961566,229.452111111111,51,229.246004751229,54,228.452111111111,228.246004751229,-0.206106359882426
|
||||||
|
OC332179,2,183094,227.677555555556,52,230.332888592395,52,225.677555555556,228.332888592395,2.65533303683935
|
||||||
|
08286875,45.8382,268,271.165444444445,36,284.837428399735,35,225.327244444445,238.999228399736,13.6719839552909
|
||||||
|
STEPHEN$STUART SOLOMON$CONWAY$1948$2,10,5851,233.584888888889,49,237.537965323381,50,223.584888888889,227.537965323381,3.95307643449249
|
||||||
|
OC301825,17,2009,239.057666666667,47,242.212988425641,47,222.057666666667,225.212988425641,3.15532175897408
|
||||||
|
BRYAN$ANTHONY$THORNTON$1955$7,69.9992,135,284.260888888889,32,287.332221480987,34,214.261688888889,217.333021480987,3.07133259209854
|
||||||
|
01470151,38,387,251.364888888889,42,252.900520102143,40,213.364888888889,214.900520102143,1.53563121325368
|
||||||
|
00306718,2,173704,211.167444444445,59,213.813343161875,59,209.167444444445,211.813343161875,2.64589871743056
|
||||||
|
MICHAEL$BARRY$TABOR$1941$10,2,194884,211.140666666667,60,214.829760965527,58,209.140666666667,212.829760965527,3.68909429886025
|
||||||
|
SC045551,4,42589,211.497,58,213.557523551435,60,207.497,209.557523551435,2.06052355143524
|
||||||
|
BELLWAY P.L.C.$ENGLAND$1372603,21,1279,227.340222222222,53,229.460570633214,53,206.340222222222,208.460570633214,2.12034841099197
|
||||||
|
05172586,1,619407,207.170222222222,64,209.167889557863,63,206.170222222222,208.167889557863,1.99766733564073
|
||||||
|
RADOVAN$NA$KOLJEVIC$1967$5,4,47760,208.412777777778,63,210.999332888592,62,204.412777777778,206.999332888592,2.58655511081454
|
||||||
|
06397765,7.5069,11088,208.652111111111,62,211.130367917775,61,201.145211111111,203.623467917775,2.47825680666418
|
||||||
|
BENZION$SCHALOM ELIEZER$FRESHWATER$1948$4,19.5484,1526,216.430333333333,56,215.557869788565,57,196.881933333333,196.009469788565,-0.872463544768436
|
||||||
|
OLUWOLE$NA$KOLADE$1966$11,4,57071,197.168333333333,70,204.402107259893,66,193.168333333333,200.402107259893,7.23377392655939
|
||||||
|
ROMY$ELIZABETH$SUMMERSKILL$1967$1,6.5042,15243,199.066222222222,68,200.878082442063,69,192.562022222222,194.373882442063,1.81186021984072
|
||||||
|
SENTTHURAN$NA$NADARAJAH$1979$11,13,3685,205.072666666667,66,207,64,192.072666666667,194,1.92733333333339
|
||||||
|
03140521,35.5041,453,226.638222222222,55,241.120645742615,49,191.134122222222,205.616545742615,14.4824235203928
|
||||||
|
04120344,1,592751,191.048666666667,71,193.500249906766,72,190.048666666667,192.500249906766,2.45158324009947
|
||||||
|
KEITH$NA$BRESLAUER$1966$3,3.0098,71852,190.085222222222,72,192.94594185951,73,187.075422222222,189.93614185951,2.86071963728801
|
||||||
|
BROOKFIELD ASSET MANAGEMENT INC$CANADA$/,1.0136,555776,186.598,75,170.469159583876,90,185.5844,169.455559583876,-16.1288404161239
|
||||||
|
00521970,24,969,209.580444444445,61,217.943276147564,56,185.580444444444,193.943276147564,8.3628317031195
|
||||||
|
STEPHEN$JAMES$ROBERTSON$1960$3,2.3488,156655,186.561666666667,76,178.248028826349,80,184.212866666667,175.899228826349,-8.31363784031754
|
||||||
|
TIMOTHY$PATRICK CAMROUX$OLIVER$1944$3,0.2488,3911463,183.432777777778,78,150.791367871721,113,183.183977777778,150.542567871721,-32.6414099060569
|
||||||
|
OC313101,10.0091,5699,189.673777777778,73,191.798555400488,74,179.664677777778,181.789455400488,2.12477762271021
|
||||||
|
PATRICK$ANTHONY$MCKENNA$1956$6,7,13432,182.905444444444,79,184.950746253802,75,175.905444444444,177.950746253802,2.04530180935731
|
||||||
|
GUY$NA$HANDS$1959$8,12.5038,3793,183.917666666667,77,183.377417210637,76,171.413866666667,170.873617210637,-0.540249456029898
|
||||||
|
SC286832,19,1623,189.588777777778,74,194.009419943477,71,170.588777777778,175.009419943477,4.42064216569923
|
||||||
|
04330127,2,185351,172.353,85,174.666277436318,85,170.353,172.666277436318,2.31327743631806
|
||||||
|
06394563,3,78751,168.266,86,170.02573434901,92,165.266,167.02573434901,1.75973434901024
|
||||||
|
07023200,1,962994,165.817333333333,89,167.665219974605,95,164.817333333333,166.665219974605,1.84788664127171
|
||||||
|
MANJIT$NA$DALE$1965$6,2.3176,162072,163.911666666667,92,178.248028826349,81,161.594066666667,175.930428826349,14.3363621596826
|
||||||
|
CHARLES$GUY$CAMROUX-OLIVER$1970$4,1.2452,551192,162.649666666667,95,151.791367871721,110,161.404466666667,150.546167871721,-10.8582987949457
|
||||||
|
11499533,1,1443396,162.169888888889,97,163.898038919407,97,161.169888888889,162.898038919407,1.72815003051784
|
||||||
|
SOFTBANK GROUP CORP$JAPAN$0104-01-056795,3,80640,163.836444444444,93,168.66025626587,94,160.836444444444,165.66025626587,4.82381182142512
|
||||||
|
VISTRY (JERSEY) LIMITED$JERSEY$130175,1,672013,161.530777777778,99,171.767151202623,87,160.530777777778,170.767151202623,10.2363734248455
|
||||||
|
07934492,8,9563,168.187111111111,87,170.819869720549,88,160.187111111111,162.819869720549,2.63275860943807
|
||||||
|
OC333409,4,42092,164.090222222222,91,169.773887544448,93,160.090222222222,165.773887544448,5.68366532222564
|
||||||
|
11606773,3,93217,162.071444444444,98,161.809404862073,99,159.071444444444,158.809404862073,-0.262039582371528
|
||||||
|
03199160,4,42466,162.718555555556,94,164.33318506947,96,158.718555555556,160.33318506947,1.61462951391485
|
||||||
|
00445790,19.5101,1527,178.167555555556,82,180.399232361494,79,158.657455555556,160.889132361494,2.23167680593886
|
||||||
|
02708030,2,179758,160.509555555556,101,161.051230246867,101,158.509555555556,159.051230246867,0.54167469131167
|
||||||
|
JEAN-GUY$NA$DESJARDINS$1944$10,8,9585,165.875,88,180.731962006548,78,157.875,172.731962006548,14.8569620065477
|
||||||
|
CASTLE BIDCO LIMITED$UNITED KINGDOM$5988526,1,609460,157.867666666667,105,158.345681428497,104,156.867666666667,157.345681428497,0.478014761829996
|
||||||
|
SEAN$MICHAEL$DINNEN$1968$3,1,2659434,157.004444444444,106,158.573288802633,103,156.004444444444,157.573288802633,1.56884435818873
|
||||||
|
STEPHEN$ALLEN$SCHWARZMAN$1947$2,90,96,243.444333333333,45,244.6992587951,46,153.444333333333,154.6992587951,1.25492546176619
|
||||||
|
THE BRITISH UNITED PROVIDENT ASSOCIATION LIMITED$ENGLAND$432511,1,656307,154.393222222222,108,155.868768815271,106,153.393222222222,154.868768815271,1.47554659304876
|
||||||
|
"LENDLEASE INTERNATIONAL PTY LIMITED$NEW SOUTH WALES, AUSTRALIA$000489109",1,649756,151.971666666667,112,93.8837570262932,251,150.971666666667,92.8837570262932,-58.0879096403735
|
||||||
|
OC329299,7,13415,157.924333333333,104,153.688376714201,109,150.924333333333,146.688376714201,-4.23595661913217
|
||||||
|
08379990,11.4977,4578,162.343222222222,96,172.558543709203,86,150.845522222222,161.060843709203,10.2153214869812
|
||||||
|
QATAR INVESTMENT AUTHORITY$QATAR$/,0.9864,2818663,150.943333333333,114,170.469159583876,91,149.956933333333,169.482759583876,19.5258262505427
|
||||||
|
IMRAN$NA$HAKIM$1977$9,4.6661,33921,154.101666666667,110,158.30270905129,105,149.435566666667,153.63660905129,4.20104238462346
|
||||||
|
BRIDGEPOINT EUROPE (SGP) LIMITED$SCOTLAND$SC332267,5.5008,22630,154.380333333333,109,95.1858779163316,247,148.879533333333,89.6850779163316,-59.1944554170018
|
||||||
|
00293262,1,594882,148.146333333333,117,150.022282279097,114,147.146333333333,149.022282279097,1.87594894576387
|
||||||
|
THE LEADERS ROMANS MIDCO2 LIMITED$ENGLAND$9939213,1,1399100,147.481333333333,118,149,116,146.481333333333,148,1.51866666666672
|
||||||
|
00617987,15.2227,2511,160.153666666667,102,170.760746414119,89,144.930966666667,155.538046414119,10.6070797474518
|
||||||
|
MARK$DAVID$STOTT$1972$4,8,9812,151.257777777778,113,148.326943547396,119,143.257777777778,140.326943547396,-2.93083423038166
|
||||||
|
08669482,6.507,15133,149.444111111111,116,148.622813846579,117,142.937111111111,142.115813846579,-0.821297264532518
|
||||||
|
OC301604,9.5009,6764,150.549333333333,115,147.836555181346,120,141.048433333333,138.335655181346,-2.71277815198775
|
||||||
|
00998314,18.9966,1677,159.077,103,160,102,140.0804,141.0034,0.922999999999945
|
||||||
|
THE BERKELEY GROUP PLC$ENGLAND & WALES$1454064,38.4924,381,178.171555555556,81,177.436350655372,82,139.679155555556,138.943950655372,-0.73520490018322
|
||||||
|
03942129,7,12849,146.559666666667,119,148.500250109757,118,139.559666666667,141.500250109757,1.94058344308993
|
||||||
|
04369054,2,211643,141.481333333333,129,143.298790128817,125,139.481333333333,141.298790128817,1.81745679548422
|
||||||
|
ALISTAIR$NA$STEELE$1953$11,6,20851,144.591888888889,121,146,121,138.591888888889,140,1.40811111111108
|
||||||
|
SEGRO PLC$ENGLAND AND WALES$167591,3.4979,65636,139.189555555556,133,140.16761135978,133,135.691655555556,136.66971135978,0.978055804224397
|
||||||
|
OC319972,5.8467,21435,140.911888888889,130,142.333333333333,128,135.065188888889,136.486633333333,1.42144444444446
|
|
|
@ -0,0 +1,101 @@
|
||||||
|
participant_id,sumdpi,rankdpi,sumnpi,ranknpi,sumtransitive,ranktransitive,diff_npi_dpi,diff_transitive_dpi,diff_transitive_npi
|
||||||
|
SPECSAVERS OPTICAL SUPERSTORES LTD$UNITED KINGDOM$1721624,4,42441,1452.95022222222,1,1482.18842011308,1,1448.95022222222,1478.18842011308,29.2381978908597
|
||||||
|
ANDREW$SIMON$DAVIS$1963$7,14.5042,2788,869.487555555556,6,881.3365356159,5,854.983355555556,866.8323356159,11.8489800603431
|
||||||
|
SC095000,5,24872,670.525666666667,8,735.610780469834,7,665.525666666667,730.610780469834,65.0851138031673
|
||||||
|
11443992,11,5149,591.460888888889,10,669.338549583877,9,580.460888888889,658.338549583877,77.8776606949871
|
||||||
|
READE$NA$GRIFFITH$1965$4,1.4972,491331,636.465111111111,9,657.49955601355,10,634.967911111111,656.00235601355,21.0344449024385
|
||||||
|
PERSIMMON PLC$ENGLAND$1818486,14,2911,578.420555555556,11,586.035982151633,11,564.420555555556,572.035982151633,7.6154265960771
|
||||||
|
INTERTRUST N.V.$THE NETHERLANDS$N/A,1,887094,511.782222222222,12,519.652355682228,12,510.782222222222,518.652355682228,7.87013346000617
|
||||||
|
08885072,1,1226949,389.079222222222,19,468.031302647188,18,388.079222222222,467.031302647188,78.9520804249655
|
||||||
|
06035106,14,2982,474.829444444445,16,480.114910906034,15,460.829444444445,466.114910906034,5.28546646158912
|
||||||
|
10657226,10,6219,342.955333333334,23,462.050947342302,19,332.955333333334,452.050947342302,119.095614008968
|
||||||
|
00604574,31,587,473.602,17,481.125633609192,14,442.602,450.125633609192,7.5236336091923
|
||||||
|
11444019,6,17408,386.593444444445,20,403.985152490125,21,380.593444444445,397.985152490125,17.3917080456807
|
||||||
|
03981143,19,1624,411.697111111111,18,414.491202616254,20,392.697111111111,395.491202616254,2.79409150514357
|
||||||
|
10510800,1,1535931,366.270333333333,21,370,23,365.270333333333,369,3.72966666666673
|
||||||
|
SUN LIFE FINANCIAL INC.$CANADA$F380,1,2086664,358.225444444444,22,364.651221643269,24,357.225444444444,363.651221643269,6.42577719882496
|
||||||
|
00102498,18,1757,336.391666666667,26,377.785641323169,22,318.391666666667,359.785641323169,41.3939746565019
|
||||||
|
04569346,4,45566,328.479,27,332.883401968393,28,324.479,328.883401968393,4.40440196839307
|
||||||
|
01417162,13,3477,320.993555555555,28,333.114660129009,27,307.993555555555,320.114660129009,12.121104573454
|
||||||
|
02468686,3.9928,58547,305.836888888889,29,316.091281388984,29,301.844088888889,312.098481388984,10.2543925000952
|
||||||
|
03777037,35.4911,458,338.669111111111,25,345.770230509207,25,303.178011111111,310.279130509207,7.10111939809553
|
||||||
|
00621920,7.5086,11060,295.787333333333,30,301.826105187632,30,288.278733333333,294.317505187632,6.03877185429838
|
||||||
|
00296805,9.5097,6642,283.340333333333,33,289.902171627357,32,273.830633333333,280.392471627357,6.56183829402386
|
||||||
|
01142830,17.3204,1948,276.395222222222,35,290.62995167509,31,259.074822222222,273.30955167509,14.2347294528681
|
||||||
|
02877315,2,176901,269.693888888889,37,273.241267886102,37,267.693888888889,271.241267886102,3.54737899721323
|
||||||
|
WOODLEY$LARKIN$HUNT$1945$11,1,1331918,256.523555555556,39,261.339361555954,38,255.523555555556,260.339361555954,4.81580600039837
|
||||||
|
HANNOVER RUCK SE$GERMANY$HRB 6778,3,82217,258.389888888889,38,261,39,255.389888888889,258,2.61011111111122
|
||||||
|
00082548,39,365,286.73,31,289.415186936244,33,247.73,250.415186936244,2.68518693624372
|
||||||
|
08340090,1,785896,245.526666666667,43,248,42,244.526666666667,247,2.47333333333344
|
||||||
|
10763268,2,306820,245.016666666667,44,247.665776742879,44,243.016666666667,245.665776742879,2.6491100762122
|
||||||
|
04967001,3.1677,71002,242.283111111111,46,247.855191284282,43,239.115411111111,244.687491284282,5.57208017317043
|
||||||
|
HEIDELBERGCEMENT AG$GERMANY$HRB 33082,2,210440,236.095777777778,48,246.443097797991,45,234.095777777778,244.443097797991,10.3473200202133
|
||||||
|
OC360196,2,242598,252.407222222222,40,241.635711925833,48,250.407222222222,239.635711925833,-10.7715102963891
|
||||||
|
08286875,45.8382,268,271.165444444445,36,284.837428399735,35,225.327244444445,238.999228399736,13.6719839552909
|
||||||
|
CRH PLC$IRELAND$12965,1,617583,230.075888888889,50,235.350874688043,51,229.075888888889,234.350874688043,5.27498579915408
|
||||||
|
OC332179,2,183094,227.677555555556,52,230.332888592395,52,225.677555555556,228.332888592395,2.65533303683935
|
||||||
|
SEMPERIAN PPP INVESTMENT PARTNERS HOLDINGS LIMITED$NA$NA,1,961566,229.452111111111,51,229.246004751229,54,228.452111111111,228.246004751229,-0.206106359882426
|
||||||
|
STEPHEN$STUART SOLOMON$CONWAY$1948$2,10,5851,233.584888888889,49,237.537965323381,50,223.584888888889,227.537965323381,3.95307643449249
|
||||||
|
OC301825,17,2009,239.057666666667,47,242.212988425641,47,222.057666666667,225.212988425641,3.15532175897408
|
||||||
|
BRYAN$ANTHONY$THORNTON$1955$7,69.9992,135,284.260888888889,32,287.332221480987,34,214.261688888889,217.333021480987,3.07133259209854
|
||||||
|
01470151,38,387,251.364888888889,42,252.900520102143,40,213.364888888889,214.900520102143,1.53563121325368
|
||||||
|
MICHAEL$BARRY$TABOR$1941$10,2,194884,211.140666666667,60,214.829760965527,58,209.140666666667,212.829760965527,3.68909429886025
|
||||||
|
00306718,2,173704,211.167444444445,59,213.813343161875,59,209.167444444445,211.813343161875,2.64589871743056
|
||||||
|
SC045551,4,42589,211.497,58,213.557523551435,60,207.497,209.557523551435,2.06052355143524
|
||||||
|
BELLWAY P.L.C.$ENGLAND$1372603,21,1279,227.340222222222,53,229.460570633214,53,206.340222222222,208.460570633214,2.12034841099197
|
||||||
|
05172586,1,619407,207.170222222222,64,209.167889557863,63,206.170222222222,208.167889557863,1.99766733564073
|
||||||
|
RADOVAN$NA$KOLJEVIC$1967$5,4,47760,208.412777777778,63,210.999332888592,62,204.412777777778,206.999332888592,2.58655511081454
|
||||||
|
03140521,35.5041,453,226.638222222222,55,241.120645742615,49,191.134122222222,205.616545742615,14.4824235203928
|
||||||
|
06397765,7.5069,11088,208.652111111111,62,211.130367917775,61,201.145211111111,203.623467917775,2.47825680666418
|
||||||
|
OLUWOLE$NA$KOLADE$1966$11,4,57071,197.168333333333,70,204.402107259893,66,193.168333333333,200.402107259893,7.23377392655939
|
||||||
|
BENZION$SCHALOM ELIEZER$FRESHWATER$1948$4,19.5484,1526,216.430333333333,56,215.557869788565,57,196.881933333333,196.009469788565,-0.872463544768436
|
||||||
|
ROMY$ELIZABETH$SUMMERSKILL$1967$1,6.5042,15243,199.066222222222,68,200.878082442063,69,192.562022222222,194.373882442063,1.81186021984072
|
||||||
|
SENTTHURAN$NA$NADARAJAH$1979$11,13,3685,205.072666666667,66,207,64,192.072666666667,194,1.92733333333339
|
||||||
|
00521970,24,969,209.580444444445,61,217.943276147564,56,185.580444444444,193.943276147564,8.3628317031195
|
||||||
|
04120344,1,592751,191.048666666667,71,193.500249906766,72,190.048666666667,192.500249906766,2.45158324009947
|
||||||
|
KEITH$NA$BRESLAUER$1966$3,3.0098,71852,190.085222222222,72,192.94594185951,73,187.075422222222,189.93614185951,2.86071963728801
|
||||||
|
OC313101,10.0091,5699,189.673777777778,73,191.798555400488,74,179.664677777778,181.789455400488,2.12477762271021
|
||||||
|
PATRICK$ANTHONY$MCKENNA$1956$6,7,13432,182.905444444444,79,184.950746253802,75,175.905444444444,177.950746253802,2.04530180935731
|
||||||
|
MANJIT$NA$DALE$1965$6,2.3176,162072,163.911666666667,92,178.248028826349,81,161.594066666667,175.930428826349,14.3363621596826
|
||||||
|
STEPHEN$JAMES$ROBERTSON$1960$3,2.3488,156655,186.561666666667,76,178.248028826349,80,184.212866666667,175.899228826349,-8.31363784031754
|
||||||
|
SC286832,19,1623,189.588777777778,74,194.009419943477,71,170.588777777778,175.009419943477,4.42064216569923
|
||||||
|
JEAN-GUY$NA$DESJARDINS$1944$10,8,9585,165.875,88,180.731962006548,78,157.875,172.731962006548,14.8569620065477
|
||||||
|
04330127,2,185351,172.353,85,174.666277436318,85,170.353,172.666277436318,2.31327743631806
|
||||||
|
GUY$NA$HANDS$1959$8,12.5038,3793,183.917666666667,77,183.377417210637,76,171.413866666667,170.873617210637,-0.540249456029898
|
||||||
|
VISTRY (JERSEY) LIMITED$JERSEY$130175,1,672013,161.530777777778,99,171.767151202623,87,160.530777777778,170.767151202623,10.2363734248455
|
||||||
|
QATAR INVESTMENT AUTHORITY$QATAR$/,0.9864,2818663,150.943333333333,114,170.469159583876,91,149.956933333333,169.482759583876,19.5258262505427
|
||||||
|
BROOKFIELD ASSET MANAGEMENT INC$CANADA$/,1.0136,555776,186.598,75,170.469159583876,90,185.5844,169.455559583876,-16.1288404161239
|
||||||
|
06394563,3,78751,168.266,86,170.02573434901,92,165.266,167.02573434901,1.75973434901024
|
||||||
|
07023200,1,962994,165.817333333333,89,167.665219974605,95,164.817333333333,166.665219974605,1.84788664127171
|
||||||
|
OC333409,4,42092,164.090222222222,91,169.773887544448,93,160.090222222222,165.773887544448,5.68366532222564
|
||||||
|
SOFTBANK GROUP CORP$JAPAN$0104-01-056795,3,80640,163.836444444444,93,168.66025626587,94,160.836444444444,165.66025626587,4.82381182142512
|
||||||
|
11499533,1,1443396,162.169888888889,97,163.898038919407,97,161.169888888889,162.898038919407,1.72815003051784
|
||||||
|
07934492,8,9563,168.187111111111,87,170.819869720549,88,160.187111111111,162.819869720549,2.63275860943807
|
||||||
|
08379990,11.4977,4578,162.343222222222,96,172.558543709203,86,150.845522222222,161.060843709203,10.2153214869812
|
||||||
|
00445790,19.5101,1527,178.167555555556,82,180.399232361494,79,158.657455555556,160.889132361494,2.23167680593886
|
||||||
|
03199160,4,42466,162.718555555556,94,164.33318506947,96,158.718555555556,160.33318506947,1.61462951391485
|
||||||
|
02708030,2,179758,160.509555555556,101,161.051230246867,101,158.509555555556,159.051230246867,0.54167469131167
|
||||||
|
11606773,3,93217,162.071444444444,98,161.809404862073,99,159.071444444444,158.809404862073,-0.262039582371528
|
||||||
|
SEAN$MICHAEL$DINNEN$1968$3,1,2659434,157.004444444444,106,158.573288802633,103,156.004444444444,157.573288802633,1.56884435818873
|
||||||
|
CASTLE BIDCO LIMITED$UNITED KINGDOM$5988526,1,609460,157.867666666667,105,158.345681428497,104,156.867666666667,157.345681428497,0.478014761829996
|
||||||
|
00617987,15.2227,2511,160.153666666667,102,170.760746414119,89,144.930966666667,155.538046414119,10.6070797474518
|
||||||
|
THE BRITISH UNITED PROVIDENT ASSOCIATION LIMITED$ENGLAND$432511,1,656307,154.393222222222,108,155.868768815271,106,153.393222222222,154.868768815271,1.47554659304876
|
||||||
|
STEPHEN$ALLEN$SCHWARZMAN$1947$2,90,96,243.444333333333,45,244.6992587951,46,153.444333333333,154.6992587951,1.25492546176619
|
||||||
|
IMRAN$NA$HAKIM$1977$9,4.6661,33921,154.101666666667,110,158.30270905129,105,149.435566666667,153.63660905129,4.20104238462346
|
||||||
|
CHARLES$GUY$CAMROUX-OLIVER$1970$4,1.2452,551192,162.649666666667,95,151.791367871721,110,161.404466666667,150.546167871721,-10.8582987949457
|
||||||
|
TIMOTHY$PATRICK CAMROUX$OLIVER$1944$3,0.2488,3911463,183.432777777778,78,150.791367871721,113,183.183977777778,150.542567871721,-32.6414099060569
|
||||||
|
JAMES$RICHARD$CAMROUX-OLIVER$1967$9,0.2506,3909000,129.433666666667,144,150.791367871721,111,129.183066666667,150.540767871721,21.3577012050542
|
||||||
|
ALEXA$KATE LOUISE CAMROUX$PEARMUND$1974$12,0.2554,3903961,122.389,164,150.791367871721,112,122.1336,150.535967871721,28.402367871721
|
||||||
|
00293262,1,594882,148.146333333333,117,150.022282279097,114,147.146333333333,149.022282279097,1.87594894576387
|
||||||
|
THE LEADERS ROMANS MIDCO2 LIMITED$ENGLAND$9939213,1,1399100,147.481333333333,118,149,116,146.481333333333,148,1.51866666666672
|
||||||
|
OC329299,7,13415,157.924333333333,104,153.688376714201,109,150.924333333333,146.688376714201,-4.23595661913217
|
||||||
|
08669482,6.507,15133,149.444111111111,116,148.622813846579,117,142.937111111111,142.115813846579,-0.821297264532518
|
||||||
|
03942129,7,12849,146.559666666667,119,148.500250109757,118,139.559666666667,141.500250109757,1.94058344308993
|
||||||
|
04369054,2,211643,141.481333333333,129,143.298790128817,125,139.481333333333,141.298790128817,1.81745679548422
|
||||||
|
00998314,18.9966,1677,159.077,103,160,102,140.0804,141.0034,0.922999999999945
|
||||||
|
MARK$DAVID$STOTT$1972$4,8,9812,151.257777777778,113,148.326943547396,119,143.257777777778,140.326943547396,-2.93083423038166
|
||||||
|
ALISTAIR$NA$STEELE$1953$11,6,20851,144.591888888889,121,146,121,138.591888888889,140,1.40811111111108
|
||||||
|
GRANDVISION N.V.$NETHERLANDS$50338269,2,226915,135.064333333333,141,141.853357055101,131,133.064333333333,139.853357055101,6.78902372176793
|
||||||
|
SONY CORPORATION$JAPAN$5010401067252,10,5898,143.083555555556,126,149.025068804305,115,133.083555555556,139.025068804305,5.94151324874986
|
||||||
|
THE BERKELEY GROUP PLC$ENGLAND & WALES$1454064,38.4924,381,178.171555555556,81,177.436350655372,82,139.679155555556,138.943950655372,-0.73520490018322
|
||||||
|
OC301604,9.5009,6764,150.549333333333,115,147.836555181346,120,141.048433333333,138.335655181346,-2.71277815198775
|
|
|
@ -0,0 +1,101 @@
|
||||||
|
participant_id,sumdpi,rankdpi,sumnpi,ranknpi,sumtransitive,ranktransitive,diff_npi_dpi,diff_transitive_dpi,diff_transitive_npi
|
||||||
|
10657226,10,6219,342.955333333334,23,462.050947342302,19,332.955333333334,452.050947342302,119.095614008968
|
||||||
|
08885072,1,1226949,389.079222222222,19,468.031302647188,18,388.079222222222,467.031302647188,78.9520804249655
|
||||||
|
11443992,11,5149,591.460888888889,10,669.338549583877,9,580.460888888889,658.338549583877,77.8776606949871
|
||||||
|
SC095000,5,24872,670.525666666667,8,735.610780469834,7,665.525666666667,730.610780469834,65.0851138031673
|
||||||
|
03954113,0,3928492,0,3928492,56.3302557872759,564,0,56.3302557872759,56.3302557872759
|
||||||
|
00102498,18,1757,336.391666666667,26,377.785641323169,22,318.391666666667,359.785641323169,41.3939746565019
|
||||||
|
04220587,0,3938440,0,3938440,40.9288958121612,898,0,40.9288958121612,40.9288958121612
|
||||||
|
SURINDER$SINGH$TOOR$1972$3,2.5015,140665,39.3167777777778,947,73.9610142643602,357,36.8152777777778,71.4595142643602,34.6442364865824
|
||||||
|
02622406,1,953107,38.5817777777778,985,70.2113074441665,391,37.5817777777778,69.2113074441665,31.6295296663887
|
||||||
|
GARY$WILLIAM$TIPPER$1963$7,1.3341,536858,99.1909999999999,223,129.988376131723,146,97.8569,128.654276131723,30.7973761317235
|
||||||
|
SPECSAVERS OPTICAL SUPERSTORES LTD$UNITED KINGDOM$1721624,4,42441,1452.95022222222,1,1482.18842011308,1,1448.95022222222,1478.18842011308,29.2381978908597
|
||||||
|
RICHARD$LEONARD$SIMMONDS$1979$12,6.5138,15035,6.48166666666667,20445,35.4645679773307,1114,-0.0321333333333325,28.9507679773307,28.9829013106641
|
||||||
|
RUPERT$CHARLES GIFFORD$LYWOOD$1958$5,7.9889,10834,47.7837777777778,702,76.5569512712771,338,39.7948777777778,68.568051271277,28.7731734934993
|
||||||
|
ALEXA$KATE LOUISE CAMROUX$PEARMUND$1974$12,0.2554,3903961,122.389,164,150.791367871721,112,122.1336,150.535967871721,28.402367871721
|
||||||
|
MARK$NA$JOSPEH$1968$4,0,4034257,0,4034257,25.1249995937727,1853,0,25.1249995937727,25.1249995937727
|
||||||
|
HEINZ$HEINRICH$BAUER$1939$10,0,3971319,0,3971319,24.4692916321143,1966,0,24.4692916321143,24.4692916321143
|
||||||
|
BAJLINDER$KAUR$BOPARAN$1968$1,0,3961511,0,3961511,24.3750001727124,1972,0,24.3750001727124,24.3750001727124
|
||||||
|
OC326165,6,17447,103.840111111111,209,127.677935557153,150,97.8401111111111,121.677935557153,23.8378244460417
|
||||||
|
MATTHEW$JAMES$WOODS$1975$12,3.8241,59659,36.409,1084,58.7450903400284,538,32.5849,54.9209903400284,22.3360903400283
|
||||||
|
JAMES$RICHARD$CAMROUX-OLIVER$1967$9,0.2506,3909000,129.433666666667,144,150.791367871721,111,129.183066666667,150.540767871721,21.3577012050542
|
||||||
|
READE$NA$GRIFFITH$1965$4,1.4972,491331,636.465111111111,9,657.49955601355,10,634.967911111111,656.00235601355,21.0344449024385
|
||||||
|
JOHN$NA$MCDONAGH$1969$5,1,1427664,87.3861111111112,276,107.213226733802,198,86.3861111111112,106.213226733802,19.8271156226908
|
||||||
|
QATAR INVESTMENT AUTHORITY$QATAR$/,0.9864,2818663,150.943333333333,114,170.469159583876,91,149.956933333333,169.482759583876,19.5258262505427
|
||||||
|
MICHELE$CAROLINE$GIDDENS$1965$9,0.9972,2793601,64.0042222222222,457,82.8732036087854,304,63.0070222222222,81.8760036087855,18.8689813865632
|
||||||
|
MINISTRY OF FINANCE$NA$NA,1,876006,1,846460,19.8318760999104,2774,0,18.8318760999104,18.8318760999104
|
||||||
|
SAMUEL$GEORGE ARMSTRONG$VESTEY$1941$3,1,593454,1,604909,19.1250009915149,2878,0,18.1250009915149,18.1250009915149
|
||||||
|
LAURENCE$JON$FUMAGALLI$1972$4,0.3379,3785704,37.7572222222222,1026,55.6425456988741,572,37.4193222222222,55.3046456988741,17.8853234766518
|
||||||
|
TAREQ$NA$NAQIB$1971$12,0,3948415,0,3948415,17.6250004758762,3357,0,17.6250004758762,17.6250004758762
|
||||||
|
11444019,6,17408,386.593444444445,20,403.985152490125,21,380.593444444445,397.985152490125,17.3917080456807
|
||||||
|
JENNIFER$CHRISTINE$LANCE$1966$9,0,3935050,0,3935050,16.7499169617751,3669,0,16.7499169617751,16.7499169617751
|
||||||
|
MICHAEL$JOSEPH$RYAN$1966$4,1,1431899,91.9212222222223,255,107.213226725418,199,90.9212222222223,106.213226725418,15.2920045031962
|
||||||
|
RONAN$NA$KILDUFF$1977$11,2,258052,40.3115555555556,909,55.5248600177933,575,38.3115555555556,53.5248600177933,15.2133044622377
|
||||||
|
JEAN-GUY$NA$DESJARDINS$1944$10,8,9585,165.875,88,180.731962006548,78,157.875,172.731962006548,14.8569620065477
|
||||||
|
03140521,35.5041,453,226.638222222222,55,241.120645742615,49,191.134122222222,205.616545742615,14.4824235203928
|
||||||
|
MANJIT$NA$DALE$1965$6,2.3176,162072,163.911666666667,92,178.248028826349,81,161.594066666667,175.930428826349,14.3363621596826
|
||||||
|
FREDERICK$PAUL$GRAHAM-WATSON$1957$9,0.6741,2838997,0.337333333333333,3651011,14.583333305857,4612,-0.336766666666667,13.909233305857,14.2459999725236
|
||||||
|
01142830,17.3204,1948,276.395222222222,35,290.62995167509,31,259.074822222222,273.30955167509,14.2347294528681
|
||||||
|
09405653,2.3244,161817,11.1937777777778,7225,25.270673368002,1841,8.86937777777778,22.946273368002,14.0768955902242
|
||||||
|
08286875,45.8382,268,271.165444444445,36,284.837428399735,35,225.327244444445,238.999228399736,13.6719839552909
|
||||||
|
LORRAINE$GERALDINE$SPENCER$1959$5,0.4936,3669613,0.491888888888889,3568226,14.1200884354501,4801,-0.001711111111111,13.6264884354501,13.6281995465612
|
||||||
|
DAVID$JEREMY$COURTENAY-STAMP$1962$4,0.4911,3727778,0.495222222222222,3462306,14.1200884354501,4800,0.00412222222222203,13.6289884354501,13.6248662132279
|
||||||
|
00395826,8,9152,107.014666666667,196,120.608813410091,167,99.0146666666666,112.608813410091,13.5941467434246
|
||||||
|
LEON$PAUL$JONES$1980$4,4.9752,33464,4.993,36314,18.564442877988,3069,0.0177999999999994,13.589242877988,13.571442877988
|
||||||
|
INFLEXION PARTNERSHIP CAPITAL FUND I GENERAL PARTNER GUERNSEY LIMITED (AS GP OF INFLEXION PARTNERSHIP CAPITAL FUND I GENERAL PARTNER GUERNSEY LP)$GUERNSEY$2075,0,4017857,0,4017857,13.4999998990969,5310,0,13.4999998990969,13.4999998990969
|
||||||
|
WILLIAM$BRUCE$KENDALL$1961$8,3.3652,68795,25.8097777777778,1799,39.2585890720939,949,22.4445777777778,35.8933890720939,13.4488112943161
|
||||||
|
EMILIO$NA$DI SPIEZIO SARDO$1977$3,0.4986,3404264,0.502333333333333,3035959,13.9507883608242,5119,0.00373333333333298,13.4521883608242,13.4484550274908
|
||||||
|
TRISTAN$NICHOLAS$RAMUS$1972$9,1.5078,415660,10.7518888888889,8051,24,1998,9.24408888888889,22.4922,13.2481111111111
|
||||||
|
STEPHEN$BERNARD$LILLEY$1968$4,0.3332,3834029,42.5912222222222,840,55.6425456988741,573,42.2580222222222,55.3093456988741,13.0513234766518
|
||||||
|
PETER$STEVEN CHRISTOPHER$BRODNICKI$1962$5,2,200423,40.178,913,53.145803755235,604,38.178,51.145803755235,12.967803755235
|
||||||
|
12242839,0,3952163,0,3952163,12.950317378398,5822,0,12.950317378398,12.950317378398
|
||||||
|
"LO HOLDING SA$GENEVA, SWITZERLAND$CH-660.1.793.002-5",1,971706,19.2664444444445,2881,31.9039719623088,1325,18.2664444444445,30.9039719623088,12.6375275178643
|
||||||
|
ETL INTERNATIONAL AG$GERMANY$DE281293152,1,1101695,34.641,1163,47.1368207299801,720,33.641,46.1368207299801,12.4958207299801
|
||||||
|
ANN$HERON$GLOAG$1942$12,14.8211,2760,48.6741111111111,673,60.894495044748,507,33.8530111111111,46.073395044748,12.2203839336369
|
||||||
|
01417162,13,3477,320.993555555555,28,333.114660129009,27,307.993555555555,320.114660129009,12.121104573454
|
||||||
|
BARRY$MICHAEL$LAMBERT$1950$6,0.5063,2939590,0.487777777777778,3613444,12.5000001377942,5977,-0.018522222222222,11.9937001377942,12.0122223600164
|
||||||
|
"ARES MANAGEMENT, LP$UNITED STATES$5434226",0,3978278,0,3978278,12.0000016961216,6231,0,12.0000016961216,12.0000016961216
|
||||||
|
ASHLEY$NA$SHAFFER$1998$6,0,3990947,0,3990947,11.8975784234647,6695,0,11.8975784234647,11.8975784234647
|
||||||
|
ANDREW$SIMON$DAVIS$1963$7,14.5042,2788,869.487555555556,6,881.3365356159,5,854.983355555556,866.8323356159,11.8489800603431
|
||||||
|
SCULPTOR FINANCE (CO) IRELAND DAC$IRELAND$IE505730,0,4001939,0,4001939,11.6249997005068,6844,0,11.6249997005068,11.6249997005068
|
||||||
|
SANDRA$JANE$THOMAS$1959$6,0.4972,3497712,0.498111111111111,3306292,11.7499998716466,6765,0.000911111111111029,11.2527998716466,11.2518887605355
|
||||||
|
JOHN$ANDREW$GATLEY$1964$10,0.5029,3104410,1.48466666666667,527841,12.5623124763371,5969,0.981766666666668,12.0594124763371,11.0776458096704
|
||||||
|
CORRINA$DAWN$BRYANT$1969$5,0,3933043,0,3933043,10.9503433326613,7818,0,10.9503433326613,10.9503433326613
|
||||||
|
DANIEL$NA$GRABINER$1989$6,1.6637,405971,1.656,412609,12.5892858526144,5956,-0.00769999999999915,10.9255858526144,10.9332858526144
|
||||||
|
THOMAS$HENRY GREVILLE$HOWARD$1983$8,0,3935727,0,3935727,10.9146088862447,7829,0,10.9146088862447,10.9146088862447
|
||||||
|
ANDREW$WILLIAM$JOHNSON$1963$9,3.3326,69890,37.232,1048,47.8429172054689,714,33.8994,44.5103172054689,10.6109172054689
|
||||||
|
00617987,15.2227,2511,160.153666666667,102,170.760746414119,89,144.930966666667,155.538046414119,10.6070797474518
|
||||||
|
MICHAEL$DOMINIC WELLS$HALL$1972$3,0.5027,3111819,0.486333333333333,3618157,10.9999998810223,7805,-0.016366666666667,10.4972998810223,10.513666547689
|
||||||
|
HEIDELBERGCEMENT AG$GERMANY$HRB 33082,2,210440,236.095777777778,48,246.443097797991,45,234.095777777778,244.443097797991,10.3473200202133
|
||||||
|
MICHAEL$JAMES$BLAKE$1968$2,0.494,3657945,27.7928888888889,1590,38.1248797687994,1002,27.2988888888889,37.6308797687994,10.3319908799105
|
||||||
|
RICHARD$NICHOLAS ROSTRON$JONES$1966$4,2,303842,3.98344444444444,63402,14.2451502669142,4761,1.98344444444444,12.2451502669142,10.2617058224698
|
||||||
|
02468686,3.9928,58547,305.836888888889,29,316.091281388984,29,301.844088888889,312.098481388984,10.2543925000952
|
||||||
|
VISTRY (JERSEY) LIMITED$JERSEY$130175,1,672013,161.530777777778,99,171.767151202623,87,160.530777777778,170.767151202623,10.2363734248455
|
||||||
|
08379990,11.4977,4578,162.343222222222,96,172.558543709203,86,150.845522222222,161.060843709203,10.2153214869812
|
||||||
|
HENRY$WILLIAM$MCCOWEN$1971$12,2.8206,129307,2.83877777777778,138598,13.0007567655629,5423,0.0181777777777778,10.1801567655629,10.1619789877851
|
||||||
|
SAMIT$NA$UNADKAT$1984$12,2.5044,135873,3.36233333333333,78854,13.4999999623873,5309,0.857933333333333,10.9955999623873,10.1376666290539
|
||||||
|
NAOMI$NA$ACKERMAN$1945$6,1.4977,486950,1.49566666666667,502378,11.625,6837,-0.00203333333333311,10.1273,10.1293333333333
|
||||||
|
ISSAM$NA$HAMID$1975$10,2,299961,2,292484,12.1249999906485,6199,0,10.1249999906485,10.1249999906485
|
||||||
|
03633621,2,172902,90.2366666666666,267,100.349439779867,219,88.2366666666666,98.3494397798666,10.1127731132
|
||||||
|
ZACHARY$NA$BISHTI$1988$7,3,108334,20.333,2634,30.4273895728086,1397,17.333,27.4273895728086,10.0943895728086
|
||||||
|
01043742,1,625767,14.8533333333333,4592,24.9024019556525,1921,13.8533333333333,23.9024019556525,10.0490686223191
|
||||||
|
PETER$ALBERT$COOPER$1953$10,4,42113,4,49247,14,5095,0,10,10
|
||||||
|
SACHIN$NA$UNADKAT$1982$6,15.5009,2459,15.5034444444444,4190,25.4999999623873,1824,0.00254444444444424,9.99909996238727,9.99655551794283
|
||||||
|
GARRY$NA$WARD$1961$7,2.3325,159728,13.4524444444444,5312,23.3249281555421,2108,11.1199444444444,20.9924281555421,9.87248371109761
|
||||||
|
GREENCORE HOLDINGS DESIGNATED ACTIVITY COMPANY$IRELAND$189728,0,3963575,0,3963575,9.75000020519733,9402,0,9.75000020519733,9.75000020519733
|
||||||
|
"INTERNATIONAL CONSOLIDATED AIRLINES GROUP S.A$SPAIN$M-492,129",0,3926334,0,3926334,9.6976830456954,9454,0,9.6976830456954,9.6976830456954
|
||||||
|
KELLY$PATRICIA$LYNCH$1978$1,0,4014781,0,4014781,9.68490491039554,9458,0,9.68490491039554,9.68490491039554
|
||||||
|
01571671,0,3926881,0,3926881,9.58333352036852,9574,0,9.58333352036852,9.58333352036852
|
||||||
|
JOANNA$AVRIL$GREENSLADE$1971$6,3.9861,58877,68.6014444444444,406,78.1836318506682,334,64.6153444444445,74.1975318506682,9.58218740622378
|
||||||
|
SOCIETE GENERALE SA$FRANCE$522 120 222,1,686548,33.0712222222222,1241,42.4430451234882,853,32.0712222222222,41.4430451234882,9.37182290126601
|
||||||
|
MITSUI FUDOSAN COMPANY LIMITED$JAPAN$6010001034957,1,645719,22.8772222222222,2179,32.2480230510692,1287,21.8772222222222,31.2480230510692,9.37080082884702
|
||||||
|
FREDERICK$MATTHEW$GOLTZ$1971$2,3.51,60556,25.4353333333333,1831,34.6923156826883,1149,21.9253333333333,31.1823156826883,9.25698234935495
|
||||||
|
OC343897,18,1781,22.2437777777778,2288,31.4839373881916,1339,4.24377777777778,13.4839373881916,9.24015961041386
|
||||||
|
"MITSUI & CO., LTD.$TOKYO, JAPAN$0100-01-008767",2,174235,3.978,64852,13.1056937212174,5403,1.978,11.1056937212174,9.12769372121744
|
||||||
|
AGNES$MARY$BARTON$1954$11,1.5056,423987,1.49044444444445,522171,10.5000000375751,8027,-0.0151555555555551,8.99440003757511,9.00955559313067
|
||||||
|
STEWART$JOHN$BAIRD$1971$6,1.4977,486982,1.50177777777778,457485,10.5000000826251,8026,0.00407777777777785,9.00230008262511,8.99822230484733
|
||||||
|
09223445,8,9761,105.484333333333,202,114.285513828357,187,97.4843333333334,106.285513828357,8.80118049502339
|
||||||
|
LONDON PENSIONS FUND AUTHORITY$NA$NA,3,124668,4.47844444444444,44617,13.2738780233112,5361,1.47844444444444,10.2738780233112,8.79543357886673
|
||||||
|
PIERRE-ANTOINE$NA$DE SELANCY$1971$7,1,1916920,11.3902222222222,7126,20.1008173766366,2638,10.3902222222222,19.1008173766366,8.71059515441439
|
||||||
|
KRISTEN$NA$ODENDAAL$1974$8,0.8324,2829267,5.71855555555556,27189,14.4122614737835,4709,4.88615555555556,13.5798614737835,8.69370591822793
|
||||||
|
DECLAN$NA$WALSH$1966$3,1,697237,1,685912,9.69015582182692,9456,0,8.69015582182692,8.69015582182692
|
|
491
output/alphaicon_paper/uk_orgs_algorithm_evaluation_recall.csv
Normal file
491
output/alphaicon_paper/uk_orgs_algorithm_evaluation_recall.csv
Normal file
|
@ -0,0 +1,491 @@
|
||||||
|
algorithm,k,superholderpriority,recall
|
||||||
|
baseline,1,0,0.384309831181728
|
||||||
|
dpi,1,0,0.385302879841112
|
||||||
|
dpi,1,1,0.386295928500497
|
||||||
|
npi,1,0,0.893743793445879
|
||||||
|
npi,1,1,0.893743793445879
|
||||||
|
transitive_alpha0.0,1,0,0.384309831181728
|
||||||
|
transitive_alpha0.0,1,1,0.386295928500497
|
||||||
|
transitive_alpha0.1,1,0,0.385302879841112
|
||||||
|
transitive_alpha0.1,1,1,0.950347567030784
|
||||||
|
transitive_alpha0.2,1,0,0.385302879841112
|
||||||
|
transitive_alpha0.2,1,1,0.950347567030784
|
||||||
|
transitive_alpha0.3,1,0,0.386295928500497
|
||||||
|
transitive_alpha0.3,1,1,0.950347567030784
|
||||||
|
transitive_alpha0.4,1,0,0.386295928500497
|
||||||
|
transitive_alpha0.4,1,1,0.950347567030784
|
||||||
|
transitive_alpha0.5,1,0,0.399205561072493
|
||||||
|
transitive_alpha0.5,1,1,0.952333664349553
|
||||||
|
transitive_alpha0.6,1,0,0.405163853028798
|
||||||
|
transitive_alpha0.6,1,1,0.952333664349553
|
||||||
|
transitive_alpha0.7,1,0,0.407149950347567
|
||||||
|
transitive_alpha0.7,1,1,0.953326713008937
|
||||||
|
transitive_alpha0.8,1,0,0.483614697120159
|
||||||
|
transitive_alpha0.8,1,1,0.960278053624628
|
||||||
|
transitive_alpha0.9,1,0,0.491559086395233
|
||||||
|
transitive_alpha0.9,1,1,0.961271102284012
|
||||||
|
transitive_alpha0.999,1,0,0.491559086395233
|
||||||
|
transitive_alpha0.999,1,1,0.961271102284012
|
||||||
|
transitivedpi_alpha0.0,1,0,0.385302879841112
|
||||||
|
transitivedpi_alpha0.0,1,1,0.385302879841112
|
||||||
|
transitivedpi_alpha0.1,1,0,0.385302879841112
|
||||||
|
transitivedpi_alpha0.1,1,1,0.899702085402185
|
||||||
|
transitivedpi_alpha0.2,1,0,0.385302879841112
|
||||||
|
transitivedpi_alpha0.2,1,1,0.899702085402185
|
||||||
|
transitivedpi_alpha0.3,1,0,0.385302879841112
|
||||||
|
transitivedpi_alpha0.3,1,1,0.899702085402185
|
||||||
|
transitivedpi_alpha0.4,1,0,0.385302879841112
|
||||||
|
transitivedpi_alpha0.4,1,1,0.899702085402185
|
||||||
|
transitivedpi_alpha0.5,1,0,0.39622641509434
|
||||||
|
transitivedpi_alpha0.5,1,1,0.901688182720953
|
||||||
|
transitivedpi_alpha0.6,1,0,0.404170804369414
|
||||||
|
transitivedpi_alpha0.6,1,1,0.901688182720953
|
||||||
|
transitivedpi_alpha0.7,1,0,0.405163853028798
|
||||||
|
transitivedpi_alpha0.7,1,1,0.901688182720953
|
||||||
|
transitivedpi_alpha0.8,1,0,0.479642502482622
|
||||||
|
transitivedpi_alpha0.8,1,1,0.910625620655412
|
||||||
|
transitivedpi_alpha0.9,1,0,0.479642502482622
|
||||||
|
transitivedpi_alpha0.9,1,1,0.910625620655412
|
||||||
|
transitivedpi_alpha0.999,1,0,0.479642502482622
|
||||||
|
transitivedpi_alpha0.999,1,1,0.910625620655412
|
||||||
|
baseline,2,0,0.388282025819265
|
||||||
|
dpi,2,0,0.388282025819265
|
||||||
|
dpi,2,1,0.388282025819265
|
||||||
|
npi,2,0,0.95630585898709
|
||||||
|
npi,2,1,0.95630585898709
|
||||||
|
transitive_alpha0.0,2,0,0.388282025819265
|
||||||
|
transitive_alpha0.0,2,1,0.388282025819265
|
||||||
|
transitive_alpha0.1,2,0,0.609731876861966
|
||||||
|
transitive_alpha0.1,2,1,0.975173783515392
|
||||||
|
transitive_alpha0.2,2,0,0.609731876861966
|
||||||
|
transitive_alpha0.2,2,1,0.975173783515392
|
||||||
|
transitive_alpha0.3,2,0,0.610724925521351
|
||||||
|
transitive_alpha0.3,2,1,0.975173783515392
|
||||||
|
transitive_alpha0.4,2,0,0.612711022840119
|
||||||
|
transitive_alpha0.4,2,1,0.975173783515392
|
||||||
|
transitive_alpha0.5,2,0,0.655412115193645
|
||||||
|
transitive_alpha0.5,2,1,0.974180734856008
|
||||||
|
transitive_alpha0.6,2,0,0.66137040714995
|
||||||
|
transitive_alpha0.6,2,1,0.974180734856008
|
||||||
|
transitive_alpha0.7,2,0,0.664349553128103
|
||||||
|
transitive_alpha0.7,2,1,0.975173783515392
|
||||||
|
transitive_alpha0.8,2,0,0.715988083416088
|
||||||
|
transitive_alpha0.8,2,1,0.978152929493545
|
||||||
|
transitive_alpha0.9,2,0,0.736842105263158
|
||||||
|
transitive_alpha0.9,2,1,0.978152929493545
|
||||||
|
transitive_alpha0.999,2,0,0.739821251241311
|
||||||
|
transitive_alpha0.999,2,1,0.978152929493545
|
||||||
|
transitivedpi_alpha0.0,2,0,0.387288977159881
|
||||||
|
transitivedpi_alpha0.0,2,1,0.387288977159881
|
||||||
|
transitivedpi_alpha0.1,2,0,0.609731876861966
|
||||||
|
transitivedpi_alpha0.1,2,1,0.974180734856008
|
||||||
|
transitivedpi_alpha0.2,2,0,0.609731876861966
|
||||||
|
transitivedpi_alpha0.2,2,1,0.974180734856008
|
||||||
|
transitivedpi_alpha0.3,2,0,0.609731876861966
|
||||||
|
transitivedpi_alpha0.3,2,1,0.974180734856008
|
||||||
|
transitivedpi_alpha0.4,2,0,0.610724925521351
|
||||||
|
transitivedpi_alpha0.4,2,1,0.974180734856008
|
||||||
|
transitivedpi_alpha0.5,2,0,0.656405163853029
|
||||||
|
transitivedpi_alpha0.5,2,1,0.979145978152929
|
||||||
|
transitivedpi_alpha0.6,2,0,0.658391261171798
|
||||||
|
transitivedpi_alpha0.6,2,1,0.973187686196624
|
||||||
|
transitivedpi_alpha0.7,2,0,0.659384309831182
|
||||||
|
transitivedpi_alpha0.7,2,1,0.974180734856008
|
||||||
|
transitivedpi_alpha0.8,2,0,0.692154915590864
|
||||||
|
transitivedpi_alpha0.8,2,1,0.977159880834161
|
||||||
|
transitivedpi_alpha0.9,2,0,0.710029791459782
|
||||||
|
transitivedpi_alpha0.9,2,1,0.977159880834161
|
||||||
|
transitivedpi_alpha0.999,2,0,0.710029791459782
|
||||||
|
transitivedpi_alpha0.999,2,1,0.977159880834161
|
||||||
|
baseline,3,0,0.388282025819265
|
||||||
|
dpi,3,0,0.388282025819265
|
||||||
|
dpi,3,1,0.388282025819265
|
||||||
|
npi,3,0,0.971201588877855
|
||||||
|
npi,3,1,0.971201588877855
|
||||||
|
transitive_alpha0.0,3,0,0.388282025819265
|
||||||
|
transitive_alpha0.0,3,1,0.388282025819265
|
||||||
|
transitive_alpha0.1,3,0,0.786494538232373
|
||||||
|
transitive_alpha0.1,3,1,0.99304865938431
|
||||||
|
transitive_alpha0.2,3,0,0.786494538232373
|
||||||
|
transitive_alpha0.2,3,1,0.99304865938431
|
||||||
|
transitive_alpha0.3,3,0,0.787487586891758
|
||||||
|
transitive_alpha0.3,3,1,0.99304865938431
|
||||||
|
transitive_alpha0.4,3,0,0.788480635551142
|
||||||
|
transitive_alpha0.4,3,1,0.99304865938431
|
||||||
|
transitive_alpha0.5,3,0,0.808341608738828
|
||||||
|
transitive_alpha0.5,3,1,0.991062562065541
|
||||||
|
transitive_alpha0.6,3,0,0.811320754716981
|
||||||
|
transitive_alpha0.6,3,1,0.991062562065541
|
||||||
|
transitive_alpha0.7,3,0,0.815292949354518
|
||||||
|
transitive_alpha0.7,3,1,0.992055610724926
|
||||||
|
transitive_alpha0.8,3,0,0.843098311817279
|
||||||
|
transitive_alpha0.8,3,1,0.98609731876862
|
||||||
|
transitive_alpha0.9,3,0,0.862959285004965
|
||||||
|
transitive_alpha0.9,3,1,0.98609731876862
|
||||||
|
transitive_alpha0.999,3,0,0.867924528301887
|
||||||
|
transitive_alpha0.999,3,1,0.98609731876862
|
||||||
|
transitivedpi_alpha0.0,3,0,0.387288977159881
|
||||||
|
transitivedpi_alpha0.0,3,1,0.387288977159881
|
||||||
|
transitivedpi_alpha0.1,3,0,0.789473684210526
|
||||||
|
transitivedpi_alpha0.1,3,1,0.992055610724926
|
||||||
|
transitivedpi_alpha0.2,3,0,0.789473684210526
|
||||||
|
transitivedpi_alpha0.2,3,1,0.992055610724926
|
||||||
|
transitivedpi_alpha0.3,3,0,0.789473684210526
|
||||||
|
transitivedpi_alpha0.3,3,1,0.992055610724926
|
||||||
|
transitivedpi_alpha0.4,3,0,0.789473684210526
|
||||||
|
transitivedpi_alpha0.4,3,1,0.992055610724926
|
||||||
|
transitivedpi_alpha0.5,3,0,0.805362462760675
|
||||||
|
transitivedpi_alpha0.5,3,1,0.995034756703079
|
||||||
|
transitivedpi_alpha0.6,3,0,0.807348560079444
|
||||||
|
transitivedpi_alpha0.6,3,1,0.990069513406157
|
||||||
|
transitivedpi_alpha0.7,3,0,0.808341608738828
|
||||||
|
transitivedpi_alpha0.7,3,1,0.991062562065541
|
||||||
|
transitivedpi_alpha0.8,3,0,0.823237338629593
|
||||||
|
transitivedpi_alpha0.8,3,1,0.985104270109235
|
||||||
|
transitivedpi_alpha0.9,3,0,0.843098311817279
|
||||||
|
transitivedpi_alpha0.9,3,1,0.985104270109235
|
||||||
|
transitivedpi_alpha0.999,3,0,0.845084409136048
|
||||||
|
transitivedpi_alpha0.999,3,1,0.985104270109235
|
||||||
|
baseline,4,0,0.388282025819265
|
||||||
|
dpi,4,0,0.388282025819265
|
||||||
|
dpi,4,1,0.388282025819265
|
||||||
|
npi,4,0,0.971201588877855
|
||||||
|
npi,4,1,0.971201588877855
|
||||||
|
transitive_alpha0.0,4,0,0.388282025819265
|
||||||
|
transitive_alpha0.0,4,1,0.388282025819265
|
||||||
|
transitive_alpha0.1,4,0,0.867924528301887
|
||||||
|
transitive_alpha0.1,4,1,0.999006951340616
|
||||||
|
transitive_alpha0.2,4,0,0.867924528301887
|
||||||
|
transitive_alpha0.2,4,1,0.999006951340616
|
||||||
|
transitive_alpha0.3,4,0,0.872889771598808
|
||||||
|
transitive_alpha0.3,4,1,0.999006951340616
|
||||||
|
transitive_alpha0.4,4,0,0.872889771598808
|
||||||
|
transitive_alpha0.4,4,1,0.999006951340616
|
||||||
|
transitive_alpha0.5,4,0,0.876861966236346
|
||||||
|
transitive_alpha0.5,4,1,0.998013902681231
|
||||||
|
transitive_alpha0.6,4,0,0.87785501489573
|
||||||
|
transitive_alpha0.6,4,1,0.998013902681231
|
||||||
|
transitive_alpha0.7,4,0,0.87785501489573
|
||||||
|
transitive_alpha0.7,4,1,0.998013902681231
|
||||||
|
transitive_alpha0.8,4,0,0.89175769612711
|
||||||
|
transitive_alpha0.8,4,1,0.99304865938431
|
||||||
|
transitive_alpha0.9,4,0,0.933465739821251
|
||||||
|
transitive_alpha0.9,4,1,0.99304865938431
|
||||||
|
transitive_alpha0.999,4,0,0.966236345580933
|
||||||
|
transitive_alpha0.999,4,1,0.99304865938431
|
||||||
|
transitivedpi_alpha0.0,4,0,0.387288977159881
|
||||||
|
transitivedpi_alpha0.0,4,1,0.387288977159881
|
||||||
|
transitivedpi_alpha0.1,4,0,0.866931479642503
|
||||||
|
transitivedpi_alpha0.1,4,1,0.998013902681231
|
||||||
|
transitivedpi_alpha0.2,4,0,0.866931479642503
|
||||||
|
transitivedpi_alpha0.2,4,1,0.998013902681231
|
||||||
|
transitivedpi_alpha0.3,4,0,0.866931479642503
|
||||||
|
transitivedpi_alpha0.3,4,1,0.998013902681231
|
||||||
|
transitivedpi_alpha0.4,4,0,0.866931479642503
|
||||||
|
transitivedpi_alpha0.4,4,1,0.998013902681231
|
||||||
|
transitivedpi_alpha0.5,4,0,0.869910625620656
|
||||||
|
transitivedpi_alpha0.5,4,1,0.999006951340616
|
||||||
|
transitivedpi_alpha0.6,4,0,0.868917576961271
|
||||||
|
transitivedpi_alpha0.6,4,1,0.997020854021847
|
||||||
|
transitivedpi_alpha0.7,4,0,0.868917576961271
|
||||||
|
transitivedpi_alpha0.7,4,1,0.997020854021847
|
||||||
|
transitivedpi_alpha0.8,4,0,0.883813306852036
|
||||||
|
transitivedpi_alpha0.8,4,1,0.992055610724926
|
||||||
|
transitivedpi_alpha0.9,4,0,0.902681231380338
|
||||||
|
transitivedpi_alpha0.9,4,1,0.992055610724926
|
||||||
|
transitivedpi_alpha0.999,4,0,0.913604766633565
|
||||||
|
transitivedpi_alpha0.999,4,1,0.992055610724926
|
||||||
|
baseline,5,0,0.388282025819265
|
||||||
|
dpi,5,0,0.388282025819265
|
||||||
|
dpi,5,1,0.388282025819265
|
||||||
|
npi,5,0,0.971201588877855
|
||||||
|
npi,5,1,0.971201588877855
|
||||||
|
transitive_alpha0.0,5,0,0.388282025819265
|
||||||
|
transitive_alpha0.0,5,1,0.388282025819265
|
||||||
|
transitive_alpha0.1,5,0,0.906653426017875
|
||||||
|
transitive_alpha0.1,5,1,1
|
||||||
|
transitive_alpha0.2,5,0,0.906653426017875
|
||||||
|
transitive_alpha0.2,5,1,1
|
||||||
|
transitive_alpha0.3,5,0,0.908639523336644
|
||||||
|
transitive_alpha0.3,5,1,1
|
||||||
|
transitive_alpha0.4,5,0,0.908639523336644
|
||||||
|
transitive_alpha0.4,5,1,1
|
||||||
|
transitive_alpha0.5,5,0,0.916583912611718
|
||||||
|
transitive_alpha0.5,5,1,1
|
||||||
|
transitive_alpha0.6,5,0,0.916583912611718
|
||||||
|
transitive_alpha0.6,5,1,1
|
||||||
|
transitive_alpha0.7,5,0,0.916583912611718
|
||||||
|
transitive_alpha0.7,5,1,1
|
||||||
|
transitive_alpha0.8,5,0,0.925521350546177
|
||||||
|
transitive_alpha0.8,5,1,0.998013902681231
|
||||||
|
transitive_alpha0.9,5,0,0.972194637537239
|
||||||
|
transitive_alpha0.9,5,1,0.998013902681231
|
||||||
|
transitive_alpha0.999,5,0,0.99304865938431
|
||||||
|
transitive_alpha0.999,5,1,0.998013902681231
|
||||||
|
transitivedpi_alpha0.0,5,0,0.387288977159881
|
||||||
|
transitivedpi_alpha0.0,5,1,0.387288977159881
|
||||||
|
transitivedpi_alpha0.1,5,0,0.905660377358491
|
||||||
|
transitivedpi_alpha0.1,5,1,0.999006951340616
|
||||||
|
transitivedpi_alpha0.2,5,0,0.905660377358491
|
||||||
|
transitivedpi_alpha0.2,5,1,0.999006951340616
|
||||||
|
transitivedpi_alpha0.3,5,0,0.905660377358491
|
||||||
|
transitivedpi_alpha0.3,5,1,0.999006951340616
|
||||||
|
transitivedpi_alpha0.4,5,0,0.905660377358491
|
||||||
|
transitivedpi_alpha0.4,5,1,0.999006951340616
|
||||||
|
transitivedpi_alpha0.5,5,0,0.906653426017875
|
||||||
|
transitivedpi_alpha0.5,5,1,0.999006951340616
|
||||||
|
transitivedpi_alpha0.6,5,0,0.907646474677259
|
||||||
|
transitivedpi_alpha0.6,5,1,0.999006951340616
|
||||||
|
transitivedpi_alpha0.7,5,0,0.907646474677259
|
||||||
|
transitivedpi_alpha0.7,5,1,0.999006951340616
|
||||||
|
transitivedpi_alpha0.8,5,0,0.916583912611718
|
||||||
|
transitivedpi_alpha0.8,5,1,0.997020854021847
|
||||||
|
transitivedpi_alpha0.9,5,0,0.938430983118173
|
||||||
|
transitivedpi_alpha0.9,5,1,0.997020854021847
|
||||||
|
transitivedpi_alpha0.999,5,0,0.98609731876862
|
||||||
|
transitivedpi_alpha0.999,5,1,0.997020854021847
|
||||||
|
baseline,6,0,0.388282025819265
|
||||||
|
dpi,6,0,0.388282025819265
|
||||||
|
dpi,6,1,0.388282025819265
|
||||||
|
npi,6,0,0.971201588877855
|
||||||
|
npi,6,1,0.971201588877855
|
||||||
|
transitive_alpha0.0,6,0,0.388282025819265
|
||||||
|
transitive_alpha0.0,6,1,0.388282025819265
|
||||||
|
transitive_alpha0.1,6,0,0.924528301886792
|
||||||
|
transitive_alpha0.1,6,1,1
|
||||||
|
transitive_alpha0.2,6,0,0.924528301886792
|
||||||
|
transitive_alpha0.2,6,1,1
|
||||||
|
transitive_alpha0.3,6,0,0.925521350546177
|
||||||
|
transitive_alpha0.3,6,1,1
|
||||||
|
transitive_alpha0.4,6,0,0.925521350546177
|
||||||
|
transitive_alpha0.4,6,1,1
|
||||||
|
transitive_alpha0.5,6,0,0.930486593843098
|
||||||
|
transitive_alpha0.5,6,1,1
|
||||||
|
transitive_alpha0.6,6,0,0.930486593843098
|
||||||
|
transitive_alpha0.6,6,1,1
|
||||||
|
transitive_alpha0.7,6,0,0.930486593843098
|
||||||
|
transitive_alpha0.7,6,1,1
|
||||||
|
transitive_alpha0.8,6,0,0.94240317775571
|
||||||
|
transitive_alpha0.8,6,1,1
|
||||||
|
transitive_alpha0.9,6,0,0.990069513406157
|
||||||
|
transitive_alpha0.9,6,1,1
|
||||||
|
transitive_alpha0.999,6,0,0.998013902681231
|
||||||
|
transitive_alpha0.999,6,1,1
|
||||||
|
transitivedpi_alpha0.0,6,0,0.387288977159881
|
||||||
|
transitivedpi_alpha0.0,6,1,0.387288977159881
|
||||||
|
transitivedpi_alpha0.1,6,0,0.923535253227408
|
||||||
|
transitivedpi_alpha0.1,6,1,0.999006951340616
|
||||||
|
transitivedpi_alpha0.2,6,0,0.923535253227408
|
||||||
|
transitivedpi_alpha0.2,6,1,0.999006951340616
|
||||||
|
transitivedpi_alpha0.3,6,0,0.923535253227408
|
||||||
|
transitivedpi_alpha0.3,6,1,0.999006951340616
|
||||||
|
transitivedpi_alpha0.4,6,0,0.923535253227408
|
||||||
|
transitivedpi_alpha0.4,6,1,0.999006951340616
|
||||||
|
transitivedpi_alpha0.5,6,0,0.924528301886792
|
||||||
|
transitivedpi_alpha0.5,6,1,0.999006951340616
|
||||||
|
transitivedpi_alpha0.6,6,0,0.929493545183714
|
||||||
|
transitivedpi_alpha0.6,6,1,0.999006951340616
|
||||||
|
transitivedpi_alpha0.7,6,0,0.929493545183714
|
||||||
|
transitivedpi_alpha0.7,6,1,0.999006951340616
|
||||||
|
transitivedpi_alpha0.8,6,0,0.938430983118173
|
||||||
|
transitivedpi_alpha0.8,6,1,0.999006951340616
|
||||||
|
transitivedpi_alpha0.9,6,0,0.95630585898709
|
||||||
|
transitivedpi_alpha0.9,6,1,0.999006951340616
|
||||||
|
transitivedpi_alpha0.999,6,0,0.996027805362463
|
||||||
|
transitivedpi_alpha0.999,6,1,0.999006951340616
|
||||||
|
baseline,7,0,0.388282025819265
|
||||||
|
dpi,7,0,0.388282025819265
|
||||||
|
dpi,7,1,0.388282025819265
|
||||||
|
npi,7,0,0.971201588877855
|
||||||
|
npi,7,1,0.971201588877855
|
||||||
|
transitive_alpha0.0,7,0,0.388282025819265
|
||||||
|
transitive_alpha0.0,7,1,0.388282025819265
|
||||||
|
transitive_alpha0.1,7,0,0.941410129096326
|
||||||
|
transitive_alpha0.1,7,1,1
|
||||||
|
transitive_alpha0.2,7,0,0.941410129096326
|
||||||
|
transitive_alpha0.2,7,1,1
|
||||||
|
transitive_alpha0.3,7,0,0.941410129096326
|
||||||
|
transitive_alpha0.3,7,1,1
|
||||||
|
transitive_alpha0.4,7,0,0.941410129096326
|
||||||
|
transitive_alpha0.4,7,1,1
|
||||||
|
transitive_alpha0.5,7,0,0.946375372393247
|
||||||
|
transitive_alpha0.5,7,1,1
|
||||||
|
transitive_alpha0.6,7,0,0.946375372393247
|
||||||
|
transitive_alpha0.6,7,1,1
|
||||||
|
transitive_alpha0.7,7,0,0.946375372393247
|
||||||
|
transitive_alpha0.7,7,1,1
|
||||||
|
transitive_alpha0.8,7,0,0.953326713008937
|
||||||
|
transitive_alpha0.8,7,1,1
|
||||||
|
transitive_alpha0.9,7,0,0.997020854021847
|
||||||
|
transitive_alpha0.9,7,1,1
|
||||||
|
transitive_alpha0.999,7,0,1
|
||||||
|
transitive_alpha0.999,7,1,1
|
||||||
|
transitivedpi_alpha0.0,7,0,0.387288977159881
|
||||||
|
transitivedpi_alpha0.0,7,1,0.387288977159881
|
||||||
|
transitivedpi_alpha0.1,7,0,0.936444885799404
|
||||||
|
transitivedpi_alpha0.1,7,1,0.999006951340616
|
||||||
|
transitivedpi_alpha0.2,7,0,0.936444885799404
|
||||||
|
transitivedpi_alpha0.2,7,1,0.999006951340616
|
||||||
|
transitivedpi_alpha0.3,7,0,0.936444885799404
|
||||||
|
transitivedpi_alpha0.3,7,1,0.999006951340616
|
||||||
|
transitivedpi_alpha0.4,7,0,0.936444885799404
|
||||||
|
transitivedpi_alpha0.4,7,1,0.999006951340616
|
||||||
|
transitivedpi_alpha0.5,7,0,0.941410129096326
|
||||||
|
transitivedpi_alpha0.5,7,1,0.999006951340616
|
||||||
|
transitivedpi_alpha0.6,7,0,0.941410129096326
|
||||||
|
transitivedpi_alpha0.6,7,1,0.999006951340616
|
||||||
|
transitivedpi_alpha0.7,7,0,0.941410129096326
|
||||||
|
transitivedpi_alpha0.7,7,1,0.999006951340616
|
||||||
|
transitivedpi_alpha0.8,7,0,0.944389275074479
|
||||||
|
transitivedpi_alpha0.8,7,1,0.999006951340616
|
||||||
|
transitivedpi_alpha0.9,7,0,0.971201588877855
|
||||||
|
transitivedpi_alpha0.9,7,1,0.999006951340616
|
||||||
|
transitivedpi_alpha0.999,7,0,0.999006951340616
|
||||||
|
transitivedpi_alpha0.999,7,1,0.999006951340616
|
||||||
|
baseline,8,0,0.388282025819265
|
||||||
|
dpi,8,0,0.388282025819265
|
||||||
|
dpi,8,1,0.388282025819265
|
||||||
|
npi,8,0,0.971201588877855
|
||||||
|
npi,8,1,0.971201588877855
|
||||||
|
transitive_alpha0.0,8,0,0.388282025819265
|
||||||
|
transitive_alpha0.0,8,1,0.388282025819265
|
||||||
|
transitive_alpha0.1,8,0,0.951340615690169
|
||||||
|
transitive_alpha0.1,8,1,1
|
||||||
|
transitive_alpha0.2,8,0,0.951340615690169
|
||||||
|
transitive_alpha0.2,8,1,1
|
||||||
|
transitive_alpha0.3,8,0,0.951340615690169
|
||||||
|
transitive_alpha0.3,8,1,1
|
||||||
|
transitive_alpha0.4,8,0,0.951340615690169
|
||||||
|
transitive_alpha0.4,8,1,1
|
||||||
|
transitive_alpha0.5,8,0,0.967229394240318
|
||||||
|
transitive_alpha0.5,8,1,1
|
||||||
|
transitive_alpha0.6,8,0,0.967229394240318
|
||||||
|
transitive_alpha0.6,8,1,1
|
||||||
|
transitive_alpha0.7,8,0,0.969215491559086
|
||||||
|
transitive_alpha0.7,8,1,1
|
||||||
|
transitive_alpha0.8,8,0,0.978152929493545
|
||||||
|
transitive_alpha0.8,8,1,1
|
||||||
|
transitive_alpha0.9,8,0,0.999006951340616
|
||||||
|
transitive_alpha0.9,8,1,1
|
||||||
|
transitive_alpha0.999,8,0,1
|
||||||
|
transitive_alpha0.999,8,1,1
|
||||||
|
transitivedpi_alpha0.0,8,0,0.387288977159881
|
||||||
|
transitivedpi_alpha0.0,8,1,0.387288977159881
|
||||||
|
transitivedpi_alpha0.1,8,0,0.951340615690169
|
||||||
|
transitivedpi_alpha0.1,8,1,0.999006951340616
|
||||||
|
transitivedpi_alpha0.2,8,0,0.951340615690169
|
||||||
|
transitivedpi_alpha0.2,8,1,0.999006951340616
|
||||||
|
transitivedpi_alpha0.3,8,0,0.951340615690169
|
||||||
|
transitivedpi_alpha0.3,8,1,0.999006951340616
|
||||||
|
transitivedpi_alpha0.4,8,0,0.951340615690169
|
||||||
|
transitivedpi_alpha0.4,8,1,0.999006951340616
|
||||||
|
transitivedpi_alpha0.5,8,0,0.951340615690169
|
||||||
|
transitivedpi_alpha0.5,8,1,0.999006951340616
|
||||||
|
transitivedpi_alpha0.6,8,0,0.95630585898709
|
||||||
|
transitivedpi_alpha0.6,8,1,0.999006951340616
|
||||||
|
transitivedpi_alpha0.7,8,0,0.95630585898709
|
||||||
|
transitivedpi_alpha0.7,8,1,0.999006951340616
|
||||||
|
transitivedpi_alpha0.8,8,0,0.95630585898709
|
||||||
|
transitivedpi_alpha0.8,8,1,0.999006951340616
|
||||||
|
transitivedpi_alpha0.9,8,0,0.984111221449851
|
||||||
|
transitivedpi_alpha0.9,8,1,0.999006951340616
|
||||||
|
transitivedpi_alpha0.999,8,0,0.999006951340616
|
||||||
|
transitivedpi_alpha0.999,8,1,0.999006951340616
|
||||||
|
baseline,9,0,0.388282025819265
|
||||||
|
dpi,9,0,0.388282025819265
|
||||||
|
dpi,9,1,0.388282025819265
|
||||||
|
npi,9,0,0.971201588877855
|
||||||
|
npi,9,1,0.971201588877855
|
||||||
|
transitive_alpha0.0,9,0,0.388282025819265
|
||||||
|
transitive_alpha0.0,9,1,0.388282025819265
|
||||||
|
transitive_alpha0.1,9,0,0.959285004965243
|
||||||
|
transitive_alpha0.1,9,1,1
|
||||||
|
transitive_alpha0.2,9,0,0.959285004965243
|
||||||
|
transitive_alpha0.2,9,1,1
|
||||||
|
transitive_alpha0.3,9,0,0.959285004965243
|
||||||
|
transitive_alpha0.3,9,1,1
|
||||||
|
transitive_alpha0.4,9,0,0.959285004965243
|
||||||
|
transitive_alpha0.4,9,1,1
|
||||||
|
transitive_alpha0.5,9,0,0.982125124131082
|
||||||
|
transitive_alpha0.5,9,1,1
|
||||||
|
transitive_alpha0.6,9,0,0.982125124131082
|
||||||
|
transitive_alpha0.6,9,1,1
|
||||||
|
transitive_alpha0.7,9,0,0.983118172790467
|
||||||
|
transitive_alpha0.7,9,1,1
|
||||||
|
transitive_alpha0.8,9,0,0.995034756703079
|
||||||
|
transitive_alpha0.8,9,1,1
|
||||||
|
transitive_alpha0.9,9,0,1
|
||||||
|
transitive_alpha0.9,9,1,1
|
||||||
|
transitive_alpha0.999,9,0,1
|
||||||
|
transitive_alpha0.999,9,1,1
|
||||||
|
transitivedpi_alpha0.0,9,0,0.387288977159881
|
||||||
|
transitivedpi_alpha0.0,9,1,0.387288977159881
|
||||||
|
transitivedpi_alpha0.1,9,0,0.954319761668322
|
||||||
|
transitivedpi_alpha0.1,9,1,0.999006951340616
|
||||||
|
transitivedpi_alpha0.2,9,0,0.954319761668322
|
||||||
|
transitivedpi_alpha0.2,9,1,0.999006951340616
|
||||||
|
transitivedpi_alpha0.3,9,0,0.954319761668322
|
||||||
|
transitivedpi_alpha0.3,9,1,0.999006951340616
|
||||||
|
transitivedpi_alpha0.4,9,0,0.954319761668322
|
||||||
|
transitivedpi_alpha0.4,9,1,0.999006951340616
|
||||||
|
transitivedpi_alpha0.5,9,0,0.959285004965243
|
||||||
|
transitivedpi_alpha0.5,9,1,0.999006951340616
|
||||||
|
transitivedpi_alpha0.6,9,0,0.970208540218471
|
||||||
|
transitivedpi_alpha0.6,9,1,0.999006951340616
|
||||||
|
transitivedpi_alpha0.7,9,0,0.970208540218471
|
||||||
|
transitivedpi_alpha0.7,9,1,0.999006951340616
|
||||||
|
transitivedpi_alpha0.8,9,0,0.970208540218471
|
||||||
|
transitivedpi_alpha0.8,9,1,0.999006951340616
|
||||||
|
transitivedpi_alpha0.9,9,0,0.998013902681231
|
||||||
|
transitivedpi_alpha0.9,9,1,0.999006951340616
|
||||||
|
transitivedpi_alpha0.999,9,0,0.999006951340616
|
||||||
|
transitivedpi_alpha0.999,9,1,0.999006951340616
|
||||||
|
baseline,10,0,0.388282025819265
|
||||||
|
dpi,10,0,0.388282025819265
|
||||||
|
dpi,10,1,0.388282025819265
|
||||||
|
npi,10,0,0.971201588877855
|
||||||
|
npi,10,1,0.971201588877855
|
||||||
|
transitive_alpha0.0,10,0,0.388282025819265
|
||||||
|
transitive_alpha0.0,10,1,0.388282025819265
|
||||||
|
transitive_alpha0.1,10,0,0.973187686196624
|
||||||
|
transitive_alpha0.1,10,1,1
|
||||||
|
transitive_alpha0.2,10,0,0.973187686196624
|
||||||
|
transitive_alpha0.2,10,1,1
|
||||||
|
transitive_alpha0.3,10,0,0.973187686196624
|
||||||
|
transitive_alpha0.3,10,1,1
|
||||||
|
transitive_alpha0.4,10,0,0.971201588877855
|
||||||
|
transitive_alpha0.4,10,1,1
|
||||||
|
transitive_alpha0.5,10,0,0.998013902681231
|
||||||
|
transitive_alpha0.5,10,1,1
|
||||||
|
transitive_alpha0.6,10,0,0.998013902681231
|
||||||
|
transitive_alpha0.6,10,1,1
|
||||||
|
transitive_alpha0.7,10,0,0.998013902681231
|
||||||
|
transitive_alpha0.7,10,1,1
|
||||||
|
transitive_alpha0.8,10,0,0.998013902681231
|
||||||
|
transitive_alpha0.8,10,1,1
|
||||||
|
transitive_alpha0.9,10,0,1
|
||||||
|
transitive_alpha0.9,10,1,1
|
||||||
|
transitive_alpha0.999,10,0,1
|
||||||
|
transitive_alpha0.999,10,1,1
|
||||||
|
transitivedpi_alpha0.0,10,0,0.387288977159881
|
||||||
|
transitivedpi_alpha0.0,10,1,0.387288977159881
|
||||||
|
transitivedpi_alpha0.1,10,0,0.960278053624628
|
||||||
|
transitivedpi_alpha0.1,10,1,0.999006951340616
|
||||||
|
transitivedpi_alpha0.2,10,0,0.960278053624628
|
||||||
|
transitivedpi_alpha0.2,10,1,0.999006951340616
|
||||||
|
transitivedpi_alpha0.3,10,0,0.960278053624628
|
||||||
|
transitivedpi_alpha0.3,10,1,0.999006951340616
|
||||||
|
transitivedpi_alpha0.4,10,0,0.960278053624628
|
||||||
|
transitivedpi_alpha0.4,10,1,0.999006951340616
|
||||||
|
transitivedpi_alpha0.5,10,0,0.971201588877855
|
||||||
|
transitivedpi_alpha0.5,10,1,0.999006951340616
|
||||||
|
transitivedpi_alpha0.6,10,0,0.984111221449851
|
||||||
|
transitivedpi_alpha0.6,10,1,0.999006951340616
|
||||||
|
transitivedpi_alpha0.7,10,0,0.984111221449851
|
||||||
|
transitivedpi_alpha0.7,10,1,0.999006951340616
|
||||||
|
transitivedpi_alpha0.8,10,0,0.984111221449851
|
||||||
|
transitivedpi_alpha0.8,10,1,0.999006951340616
|
||||||
|
transitivedpi_alpha0.9,10,0,0.999006951340616
|
||||||
|
transitivedpi_alpha0.9,10,1,0.999006951340616
|
||||||
|
transitivedpi_alpha0.999,10,0,0.999006951340616
|
||||||
|
transitivedpi_alpha0.999,10,1,0.999006951340616
|
|
File diff suppressed because it is too large
Load diff
|
|
|
|
|
|
|
|
|
|
|
|
|
|
|
|
|
|
|
|
|
|
|
Loading…
Reference in a new issue