114 lines
7.1 KiB
Markdown
114 lines
7.1 KiB
Markdown
|
||
<table>
|
||
<tbody>
|
||
<tr>
|
||
<td valign="top" width=200><img src="https://user-images.githubusercontent.com/3776887/133301237-145e43f0-d4b3-4ae5-bf15-113efc2ad189.png"></td>
|
||
<td valign="top"><h1>α-Indirect Control in Onion-like Networks</h1>
|
||
We propose a fast, accurate, and scalable algorithm to detect ultimate controlling entities in global corporate networks. α-ICON uses company-participant links to identify super-holders who exert control in networks with millions of nodes.<br><br>
|
||
By exploiting onion-like properties of such networks we iteratively peel off the hanging vertices until a dense core remains. This procedure allows for a dramatic speed-up, uncovers meaningful structures, and handles circular ownership by design.<br><br>
|
||
Read our <a href="https://arxiv.org/abs/2109.07181" target="_blank">paper</a> with the applications. As a toy example, consider the below corporate network where α-ICON designates Mr Philip Mactaggart (in green) as the super-holder exerting control over all other entities, directly or indirectly held:
|
||
</td>
|
||
</tr>
|
||
</tbody>
|
||
</table>
|
||
|
||
<img src="https://user-images.githubusercontent.com/3776887/133299028-152f030a-e1c7-428b-83ef-e5f4e92414bc.png">
|
||
|
||
## Installation
|
||
|
||
To replicate the analysis you need to clone this repository to your local machine. Then you need to install the required versions of R dependencies listed in `DEPENDENCIES`. `code/helper_functions/install_dependencies.r` automates this step, but you may still need to install the underlying libraries manually with [Homebrew](https://brew.sh) or `apt-get`, depending on your platform. Finally, you need to declare the environment variable `ALPHAICON_PATH` in bash pointing to the repository. Or, better yet, you can add it in your `.Renviron` with
|
||
```console
|
||
user:~$ echo 'ALPHAICON_PATH="path_to_cloned_repository"' >> ~/.Renviron
|
||
```
|
||
|
||
The repository does not contain any data due to its size (10+ GB unpacked); most files in `data/` and `output/` folders are zero-byte placeholders. We provide a <a href="https://drive.google.com/drive/folders/10Tq-b4BVsG3gmq2JVa026Nilzj8eojNB" target="_blank">public Google Drive folder</a> with the populated `data/` and `output/` directories. You may still need to unzip them manually.
|
||
|
||
A self-contained example of α-ICON is also available in <a href="https://colab.research.google.com/drive/1AvO8hJzwj2LoKsyxk5LfSWK7LW1U02Mc" target="_blank">Google Colaboratory</a>.
|
||
|
||
## Repository structure
|
||
|
||
```
|
||
data/
|
||
├─uk/ # Data on UK companies and participants
|
||
| ├ persons-with-significant-control-snapshot-2021-08-02.txt # Source PSC data
|
||
| ├ BasicCompanyDataAsOneFile-2021-08-01.csv # Source data on live companies in UK
|
||
| ├ sic_2007_code_list.csv # Standard Industrial Classification codes
|
||
| ├ psc_snapshot_2021-08-02.rdata # Processed People with Significant Control data
|
||
| └ uk_basic_companies_data_2021-08-01.rdata # Processed Basic Company data
|
||
|
|
||
├─corpwatch_api_tables_csv_14aug21/ # Data from CorpWatch Dump
|
||
| ├ company_info.csv # Source companies data from SEC filings
|
||
| ├ cik_name_lookup.csv # Company name variants in SEC filings
|
||
| └ company_locations.csv # Company locations in SEC filings
|
||
|
|
||
code/
|
||
├─helper_functions/
|
||
| ├ install_dependencies.r # Installs R dependencies used in the project
|
||
| └ compute_power_index.r # Computes Mizuno et al. (2020) DPI and NPI
|
||
|
|
||
├─data_preparation/
|
||
| └─uk/
|
||
| ├ 1a_process_psc_snapshot.r # Prepare source PSC data
|
||
| ├ 1b_process_companies_data.r # Prepare source data on live companies
|
||
| ├ 2_psc_snapshot_to_participants_panel.r # PSC data to entity-participant info
|
||
| └ 3_prepare_affiliated_entities_evaluation_data.r # Process CorpWatch data
|
||
|
|
||
├─alphaicon_paper/
|
||
| ├ 1_compute_alphaicon.ipynb # Jupyter Notebook w. α-ICON (also on Google Colab)
|
||
| ├ 2_compute_npi_dpi.r # Computation of Direct and Network Power Indices
|
||
| ├ 3_summary_stat_by_node_type.r # UK PSC network statistics by core/SH/ST/I
|
||
| ├ 4_illustrate_algorithm.r # Visualise selected networks
|
||
| ├ 5_algorithm_evaluation.r # Compute recall @ k and l for various algorithms
|
||
| └ 6_rank_top_holders.r # Examine the rankings of super-holders & Kendall's tau
|
||
|
|
||
output/
|
||
├─uk/
|
||
| ├ uk_organisations_participants_2021_long_2aug21.csv # Primary ownership data
|
||
| ├ uk_organisations_participation_graph_core_periphery_membership_6aug21.csv
|
||
| ├─npi_dpi/ # Mizuno et al. (2020) computation results on UK PSC data
|
||
| | └─10000iter/
|
||
| | ├ uk_organisations_participants_2021_long_7sep21_dpi_10000iter.csv # DPI
|
||
| | └ uk_organisations_participants_2021_long_7sep21_npi_10000iter.csv # NPI
|
||
| |
|
||
| ├─transitive/ # Computed α-ICON shares on equity shares or DPI weights
|
||
| | ├ uk_organisations_transitive_ownership_alpha*_2021_long_2aug21.csv # α = *
|
||
| | └ uk_organisations_transitive_ownership_alpha*_2021_long_7sep21_dpi_....csv
|
||
| |
|
||
└─alphaicon_paper/
|
||
├ uk_orgs_algorithm_evaluation_recall.csv # Algorithm recall by k
|
||
├ uk_orgs_algorithm_evaluation_recall_by_pathlength.csv # Algorithm recall by l
|
||
├ uk_organisations_top100_holders_2021_long_2aug21.csv # Top SH in PSC network
|
||
├ uk_organisations_top100_holders_diff_npi_dpi_2021_long_2aug21.csv # Top-100 SH
|
||
| # with the largest difference betw. total DPI and NPI
|
||
├ uk_organisations_top100_holders_diff_transitive_dpi_2021_long_2aug21.csv
|
||
| # Top-100 SH with the largest difference betw. total DPI and α-ICON (α=0.999)
|
||
├ uk_organisations_top100_holders_diff_transitive_npi_2021_long_2aug21.csv
|
||
| # Top-100 SH with the largest difference betw. total NPI and α-ICON (α=0.999)
|
||
└ network_examples/ # Visualisations of selected networks
|
||
```
|
||
|
||
We provide an annotated `Makefile` that documents the data analysis in our papers.
|
||
|
||
To build the ‘<a href="https://arxiv.org/abs/2109.07181" target="_blank">α-Indirect Control in Onion-like Networks</a>’ paper run `make alphaicon_paper` when in the repository folder.
|
||
|
||
Please note that those commands will not produce any publication-ready output files (e.g. tables or figures): the export statements are commented out in the code. Our intention is to make the analysis pipeline transparent to the readers with the aid of `make`:
|
||
|
||
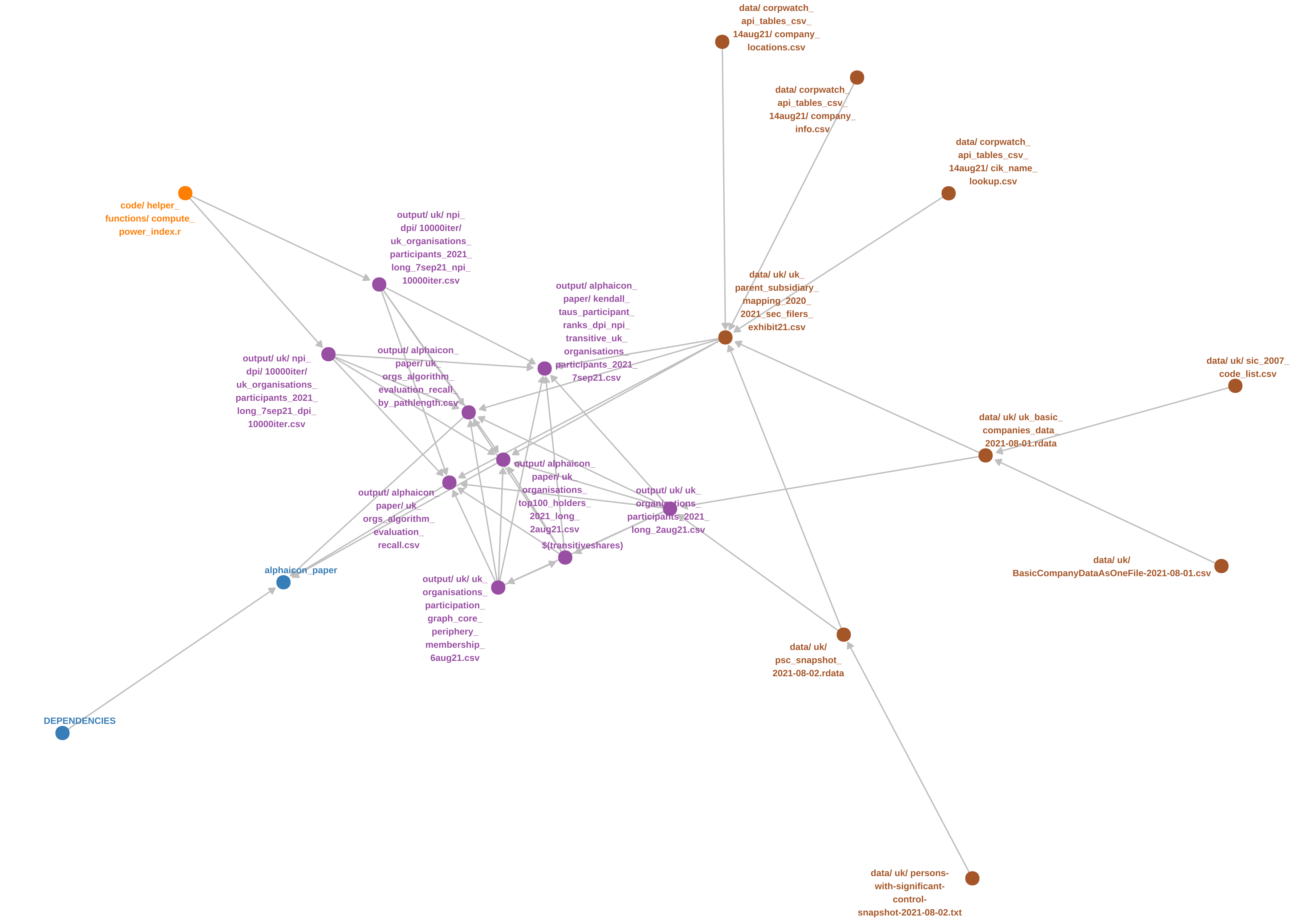
|
||
|
||
|
||
## Licence
|
||
<a rel="license" href="http://creativecommons.org/licenses/by/4.0/"><img alt="Creative Commons License" style="border-width:0" src="https://i.creativecommons.org/l/by/4.0/88x31.png" /></a><br />
|
||
Creative Commons License Attribution 4.0 International (CC BY 4.0).
|
||
|
||
Copyright © the respective contributors, as shown by the `AUTHORS` file.
|
||
|
||
People with Significant Control data is <a href="http://download.companieshouse.gov.uk/en_pscdata.html">distributed</a> by Companies House under <a href="https://www.nationalarchives.gov.uk/doc/open-government-licence/version/3/">Open Government Licence v3.0</a>.
|
||
|
||
Free Company Data Product is <a href="http://download.companieshouse.gov.uk/en_output.html">distributed</a> by Companies House under <a href="https://www.nationalarchives.gov.uk/doc/open-government-licence/version/3/">Open Government Licence v3.0</a>.
|
||
|
||
|
||
## Contacts
|
||
Dmitriy Skougarevskiy, Ph.D.
|
||
|
||
dskougarevskiy@eu.spb.ru
|